- Manchester Institute of Education, University of Manchester, Manchester, United Kingdom
Background: Latent class analysis (LCA) can be used to identify subgroups of children with similar patterns of mental health symptoms and/or strengths. The method is becoming more commonly used in child mental health research, but there are reservations about the replicability, reliability, and validity of findings.
Objective: A systematic literature review was conducted to investigate the extent to which LCA has been used to study population mental health in children, and whether replicable, reliable and valid findings have been demonstrated.
Methods: Preferred Reporting Items for Systematic Reviews and Meta-Analyses (PRISMA) guidelines were followed. A search of literature, published between January 1998 and December 2017, was carried out using MEDLINE, EMBASE, PsycInfo, Scopus, ERIC, ASSIA, and Google Scholar. A total of 2,748 studies were initially identified, of which 23 were eligible for review. The review examined the methods which studies had used to choose the number of mental health classes, the classes that they found, and whether there was evidence for the validity and reliability of the classes.
Results: Reviewed studies used LCA to investigate both disparate mental health symptoms, and those associated with specific disorders. The corpus of studies using similar indicators was small. Differences in the criteria used to select the final LCA model were found between studies. All studies found meaningful or useful subgroups, but there were differences in the extent to which the validity and reliability of classes were explicitly demonstrated.
Conclusions : LCA is a useful tool for studying and classifying child mental health at the population level. Recommendations are made to improve the application and reporting of LCA and to increase confidence in findings in the future, including use of a range of indices and criteria when enumerating classes, clear reporting of methods for replicability, and making efforts to establish the validity and reliability of identified classes.
Introduction
Rationale
It is important for researchers in the field of child mental health to be able to identify subgroups of children, within the general population, who have similar patterns of mental health symptoms and/or strengths. These subgroups can be studied further to investigate matters such as which mental health classes are commonly found in the general population, how prevalent they are, what causes them, what future outcomes they predict, and whether mental health classes change over time. In addition, identifying these subgroups is important for practitioners in the field because it allows a targeted approach to mental health promotion.
An increasingly popular method for identifying subgroups is latent class analysis (LCA). LCA is a cross-sectional latent variable mixture modeling approach. Like all latent variable mixture modeling approaches, LCA aims to find heterogeneity within the population. It does this by analyzing individuals' patterns of behavior, such as mental health indicators, and finding common types, called classes (Collins and Lanza, 2010). Each individual is probabilistically assigned to a class. That results in subgroups of individuals, who are most similar to each other and most distinct from those in other classes (Berlin et al., 2013). LCA can be carried out with categorical and/or continuous indicators, although LCA with continuous indicators is often called latent profile analysis (Muthen and Muthen, 1998-2017). For simplicity, here the term LCA is used for both analyses.
LCA has many strengths over other methods that can also be used to identify subgroups of children with similar patterns of mental health indicators. For example, children are often classified into groups according to specific mental health diagnoses on DSM-5 (American Psychiatric Association, 2013) or by exceeding a cut-off point on a continuous mental health measure (e.g., clinical cut-off points on the Strengths and Difficulties Questionnaire; Goodman, 2001). However, in both cases, individuals either side of the threshold for classification are very similar. Furthermore, heterogeneity within subgroups could be substantial (Dowdy and Kamphaus, 2007). Because those methods result in artificial, indistinct subgroups, any associations between them and other factors will be attenuated or spurious (MacCallum et al., 2002). LCA, on the other hand, uses maximum likelihood estimation to form subgroups which are internally homogenous and externally heterogeneous (Berlin et al., 2013). Therefore, researchers can more confidently use those subgroups to investigate relationships with other salient constructs, such as risk or promotive factors.
Another strength of LCA is that it is a model-based technique. A key advantage of model-based techniques over heuristic cluster techniques (e.g., k-means clustering) is that they provide fit statistics. Fit statistics assist researchers in choosing the most appropriate model for the data (Vermunt and Magidson, 2002), and can be used to compare models for hypothesis testing (Miettunen et al., 2016). Furthermore, LCA provides information on the probability that an individual is within a particular class (Vermunt and Magidson, 2002) and, as models can be extended to include covariates, this classification information can be retained in the broader model so measurement error can be accounted for (Muthen and Muthen, 1998-2017; DiStefano and Kamphaus, 2006). Thus, researchers have more flexibility and accuracy when studying mental health subtypes and associated factors.
Despite those potential benefits, critics have raised major concerns about the application of latent variable mixture modeling techniques like LCA (Bauer and Curran, 2003; Lenzenweger, 2004; Sterba and Bauer, 2010). First, LCA is usually conducted in an exploratory manner, whereby an increasing number of classes are fitted to the data and the best fitting model is chosen. The final class solution is decided upon by the researcher, who may use various criteria to choose the final model. Because this decision relies somewhat on the researchers judgement, results may not be replicable (van de Schoot et al., 2017). Second, LCA is a data-driven approach, meaning that identified classes could be statistical artifacts which lack validity and reliability (Bauer and Curran, 2004). Without establishing validity and reliability of classes, it is difficult to infer whether the classes represents naturally occurring subgroups in the population or whether they are sample specific.
There are ways to address some of the issues raised above. For example, researchers can be transparent about the methods used to derive classes and the decisions made, so that findings can be critically appraised and replicated (Collins and Lanza, 2010; van de Schoot et al., 2017). In addition, identified classes can be validated by investigating whether there are expected relationships between classes and other variables (Lenzenweger, 2004; Collins and Lanza, 2010; Hicks et al., 2017). Reliability of classes can be tested by conducting the same analysis in different samples, or with a subset of the same sample, to see if they are consistently found (Bauer and Curran, 2004; Lenzenweger, 2004). Studies may not always apply that level of rigor to their analyses.
Despite such concerns, LCA is being used more widely. This is partly due to the availability of software and increased computational capacity, which are required to carry out the analysis (Miettunen et al., 2016). In addition, a number of researchers have published papers encouraging the use of LCA in mental health and developmental research because it is well suited to addressing pertinent questions in the field. For example, Lanza and Cooper (2016) encourage the application of LCA for studying complex multidimensional phenomena, such as mental health, because multiple aspects of individual functioning can be studied holistically. Other researchers have suggested that LCA is an important analytic tool for studying developmental heterogeneity in the population (von Eye and Bergman, 2003; Scotto Rosato and Baer, 2012; Berlin et al., 2013). In addition, it can be used to assess the impact of universal mental health interventions on groups with similar mental health typologies (Greenberg and Abenavoli, 2017). LCA, then, has utility in the field of child mental health and may continue to grow in popularity as a statistical tool for researchers and practitioners.
Objectives
Despite the potential utility of LCA in the field, to date, there has been no research synthesis of studies which have used this method to investigate patterns of mental health symptoms and/or strengths in children in the general population. Therefore, it is not known to what extent LCA has been used for this purpose, whether there is a corpus of studies which produced similar findings, whether models are selected using appropriate criteria, or whether theoretically or practically meaningful mental health subtypes have been identified.
The current systematic review was carried out to provide an overview of research which has used LCA to study subgroups of children with similar patterns of mental health symptoms and/or strengths. In particular, the review focused on children in the general population, who are aged between 4 and 11 years. The age range was restricted in order to allow comparability between studies. Comparing the mental health of very young children to older children is problematic because behavior that are considered developmentally appropriate for a very young child, (e.g., hitting out or tantrums) are symptoms of externalizing problems in older children (Campbell et al., 2000; Carter et al., 2004; Fanti and Henrich, 2010). Similarly, mental health among adolescents may be qualitatively different from that of younger children. Adolescence is an important period of physical, social, and emotional change, which may impact an individual's mental health. In addition, a number of specific mental health problems become more prevalent in adolescence, such as alcohol and drug abuse, risky sexual behaviors, criminal activity, eating disorders, and self-harm (Moffitt, 1993; Eccles et al., 1996). That means comparing adolescent and child mental health cannot be done on a like-for-like basis. In addition, only studies including samples that were approximately representative of the general population were included. Studies that target specific mental health or “at risk” groups may identify mental health classes which are not commonly seen in the general population, making a comparison between findings difficult.
The main aim of the review was to investigate which aspects of mental health have been studied using LCA, and whether results were comparable. In addition, the review aimed to compare the methods used to select the final class models across studies, and investigate the extent to which classes were shown to be valid and reliable. This is important in order to be able to provide researchers and practitioners in the field of child mental health with (a) an appraisal of the current applications and potential utility of LCA for studying population mental health, (b) an indication of the rigor with which the method is currently applied, including whether the validity and reliability of found classes are demonstrated, and (c) recommendations for improving the way in which LCA is used in this area.
Research Questions
1) Which aspects of population child mental health have been studied using latent class analysis and is there a corpus of comparable results?
2) What methods have been used to decide on the final number of mental health classes?
3) To what extent are found classes shown to be valid and reliable?
Methods
The methodology and inclusion/exclusion criteria were specified in advance and documented in a protocol (https://www.crd.york.ac.uk/prospero/ Reference: CRD42017083749).
Eligibility Criteria
Studies Were Included in the Review if They Met the Following Criteria:
• Participants were aged between 4 and 11 years (inclusive).
• A general population sample was used, i.e., the sample was not targeted at or “enriched” for a specific group, such as those with a mental health diagnosis or those identified as being at high risk for developing mental health difficulties.
• Cross-sectional latent class analysis was used with categorical or continuous indicators. Studies that used longitudinal data to create the classes, for example, studies that used latent class growth analysis to create classes of children with specific mental health trajectories, were not included. That was because the longitudinal nature of the data adds another layer of meaning and it would make it difficult to compare results between studies with different designs. However, studies were not excluded purely on the basis of including longitudinal data, for example, studies with distal outcomes were included, as were studies that had carried out latent transition analysis (a longitudinal extension to latent class analysis), providing they reported latent class analyses for each time point separately.
• The LCA analysis used mental health indicators only—mental health indicators could include mental health symptoms and/or subjective wellbeing, as set out in the dual-factor model of mental health (Greenspoon and Saklofske, 2001; Suldo and Shaffer, 2008; Antaramian et al., 2010). If non-mental health indicators were included in the latent class analysis, the study was not included because this would influence the formation of the classes (Berlin et al., 2013) and would not produce subtypes based purely on mental health. Therefore, it would make it impossible to compare study results on a like-for- like basis.
Studies were excluded if eligibility criteria were not met, if the article was not available in English, or if methods and results were not reported in sufficient detail to assess eligibility and compare studies. As a minimum, studies were required to report the sample age and population from which it was drawn, the mental health indicators used to derive classes, the statistical method(s) employed, the procedure followed for deriving classes, and the name and number of derived classes.
Search Strategy
The search strategy included terms relating to (a) children, (b) latent class or latent profile analysis, and (c) mental health. The search terms were adapted for use with each bibliographic database and their thesaurus and mapping functions (see Supplementary Material S1 for full search terms).
Data Sources
The following electronic bibliographic databases were searched: MEDLINE, EMBASE, PsycINFO, Scopus, ERIC, and ASSIA. In addition, Google Scholar was searched, along with the reference lists of eligible studies and review articles.
To reduce publication bias, research was considered from a variety of sources, including peer reviewed journals, unpublished research, conference papers, and doctoral theses. The database Scopus was chosen because it includes gray literature, and Google Scholar was searched because of its inclusion of a wide range of sources (Haddaway et al., 2015).
Study Selection
Results from the initial searches were saved to Endnote and duplicates were removed. The first author scanned the title and abstract of all remaining records to identify studies that potentially met the inclusion criteria. The second author checked 20% of those to ensure consistency in sorting. The full-text versions of the remaining papers were assessed against the full inclusion and exclusion criteria by the first two authors. Disagreements or ambiguities were resolved through discussion with all authors.
Data Extraction
A data extraction sheet was developed based on the review aims1. Information on sample characteristics, method, and results for each reviewed study were extracted. Study authors were contacted for further information when a potentially eligible study was found, but not enough information was provided for review (e.g., conference abstracts, unpublished thesis; n = 13).
Data Analysis
Data on the aspects of mental health investigated using LCA, the method used for class selection, the findings and evidence for class validity or reliability were compared using summary tables.
Quality Analysis
An adapted version of the Guidelines for Reporting on Latent Trajectory Studies (GRoLTS) checklist (van de Schoot et al., 2017) was used to perform quality assessments for the included studies. The adapted checklist contained 15 yes/no items, such as, “Is the software mentioned,” “Is entropy reported?,” “Are plots/bar charts included with the response patterns of the classes/profiles in the final solution.”2 This checklist was important because clear and transparent reporting is required to be able to interpret and critically appraise results, and draw comparisons between studies.
Results
Study Selection and Characteristics
The review process identified 23 eligible studies from the 2,748 studies gathered through the initial searches (see Figure 1 for PRISMA flowchart of studies retained and excluded at each stage of the review process). Within these studies, 97 eligible analyses were carried out. Eleven studies also carried out other analyses which did not meet the criteria for review (see Table 1).
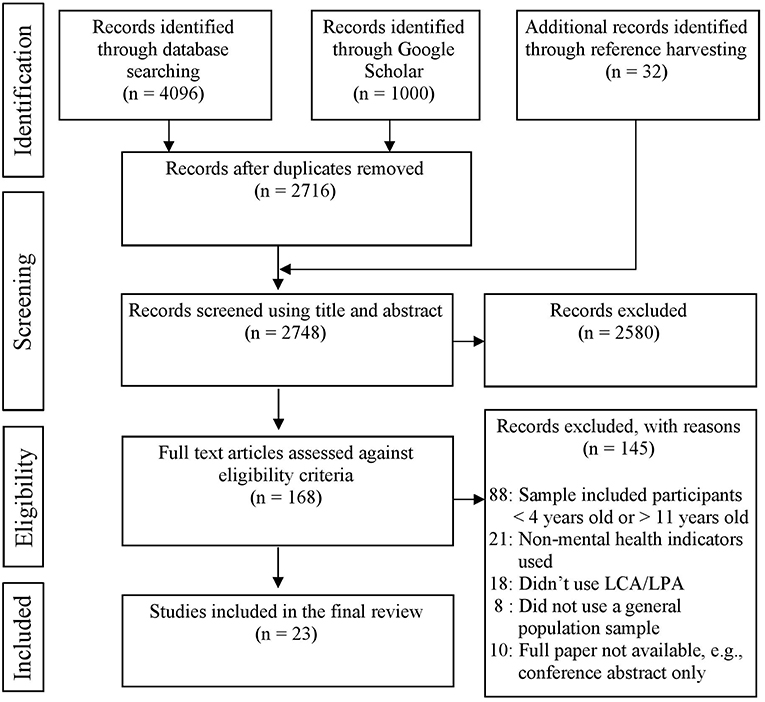
Figure 1. PRISMA flow diagram illustrating the flow of information at each stage of the review process.
Samples were drawn from a range of sources, including; large national databases, such as the Netherlands Twin Registry; large community wide research projects, such as The Family Life Project; or from datasets obtained through other research projects (see Table 1). Three pairs of studies used identical or very similar samples and the same mental health indicators for one of their latent class analyses (Baillargeon et al., 1999 and Lee et al., 2007; Basten et al., 2013 and 2016; van Lier et al., 2003a and 2003b). Sample sizes ranged between 189 and 12,334. Fifteen studies examined mental health classes in mixed sex samples and eight examined mental health classes for boys and girls separately.
Quality Analysis Results
The full quality of reporting and replicability assessment against relevant GRoLTS criteria is presented in the Supplementary Material S4. No studies were excluded from the review on the basis of reporting quality. However, the results highlight areas which are inconsistently or poorly reported, including; entropy values, the number of random start values and final iterations, and plots for all class solutions.
Individual Study Characteristics and Results
Individual study characteristics, such as sample, LCA method, identified classes and evidence for validity and/or reliability of classes are presented in Table 1.
Synthesized Findings
Comparison of Study Findings
All reviewed studies used indicators of psychopathology, but some chose indicators specific to a particular symptom (e.g., physical aggression), a specific disorder (e.g., attention deficit hyperactivity disorder; ADHD), or a broadband view of mental health which included internalizing and externalizing symptoms (see Table 1 for studies ordered according to aspects of mental health they examined). Only two studies considered positive aspects of behavior alongside symptoms (Bradshaw et al., 2015; Racz et al., 2015); no studies considered subjective wellbeing. General comparisons between studies looking at similar aspects of mental health are discussed.
Studies Using Internalizing Symptoms as Indicators
Both studies that used LCA to investigate anxiety types found ordinal classes of anxiety, despite examining different forms of anxiety and using different indicators. They also found that the low symptom class was not the largest group. Wadsworth et al. (2001) similarly found ordinal classes when investigating symptoms of depression and anxiety, and low and mild symptom groups were approximately equal in size. Indicators of another internalizing disorder, however, resulted in qualitatively different classes. Althoff et al. (2009) examined classes of obsessive-compulsive symptoms in four different samples and found that four classes consistently emerged; a large no symptom class, a high symptom class, and two other classes which represented specific symptom types.
Studies Using Externalizing Behaviors as Indicators
Of all the LCA analyses that investigated patterns of externalizing symptoms, 72 out of 77 (93%) found the non-symptomatic class to be the largest.
Studies that used indicators for a specific aspect of mental health, such as hyperactivity, inattention, or physical aggression, found classes that differed in symptom severity alone (Baillargeon et al., 1999; Hudziak et al., 1999; Romano et al., 2002; Lee et al., 2007). When indicators were broader and covered a particular disorder, such as attention deficit disorder, oppositional defiant behavior, or a broad range of behavior problems, classes differed in both type and severity (van Lier et al., 2003a,b; Althoff et al., 2006; Kuny et al., 2013).
Additionally, two studies examined conduct problems in the home and school context by combining responses from parents and teachers. One carried out two analyses with the same sample at different time points (Sulik et al., 2017). All analyses identified subgroups of children with situation specific conduct problems—home or school. There was also a large group of children with no conduct problems, and a small group with generalized conduct problems.
Studies Using a Broad Range of Mental Health Indicators
Table 1 shows that seven studies focused on multiple aspects of mental health, spanning both internalizing and externalizing problems, with two also including indicators of positive behavior (Bradshaw et al., 2015; Racz et al., 2015). Studies used different measures as indicators of mental health (see Table 1). Measures varied from non-clinical school behavior checklists, such as the Teacher Observation of Classroom Adaptation-Checklist, to more clinical measures, such as the Kiddie Schedule for Affective Disorders and Schizophrenia. Due to the range of indicators used, results were not directly comparable. However, all studies using a broad range of mental health indicators found that low symptom classes were the largest group, with the exception of Vendlinski et al. (2014), who found that the most prevalent class for girls was the mildly anxious class, and Racz et al. (2015), who found that the well-adapted and concentration problems groups were equally large in their one of their analysis.
Some studies reported similar classes to each other despite using different measures. McElroy et al. (2017) and Basten et al. (2013) found a low symptom class, a comorbid class, a pure internalizing class, and a pure externalizing class or externalizing/emotionally reactive class. Vendlinski et al. (2014) also found similar classes, with the addition of specific mental health problems classes referred to as “moderately anxious” and “moderately impulsive and inattentive.”
Bradshaw et al. (2015) was the only study to find a class that had better than average functioning, and the only multiple aspects of mental health study to find ordinal categories. All other studies found qualitative differences as well as differences in problem severity.
Studies Using Indicators for two Distinct Mental Health Problems
The remaining two studies looked at two distinct aspects of mental health. Lanza (2011) studied oppositional disorder symptoms and depression and Wall et al. (2016) studied callous-unemotional traits and conduct problems. Those studies were largely concerned with identifying subgroups of children with pure and comorbid symptoms. Both studies found small comorbid classes, and large no symptom classes, as well as classes which had higher symptoms in one disorder than the other.
General Patterns of Results Across Studies
LCA was used to identify subgroups of children with distinct patterns of mental health. The mental health indicators used in different studies varied in their specificity to a particular disorder and their severity (i.e., clinical measure or school measure). This influenced the types of classes found, although some general patterns emerged. For instance, subgroups of children with qualitatively different patterns of mental health symptoms were identified when a wide range of indicators were used. Subgroups that differed in symptom severity alone were identified when the range of symptoms used as indicators was narrow or for certain symptom types (i.e., anxiety and depression). Additionally, most analyses (89%) found the largest class was the low or no symptom class, which contained between 17% and 91% of the sample, depending on the indicators used and total number of classes found. Some comparable classes were found in studies that had used similar indicators, but the number of studies was small.
Comparison of Study Methodologies
Studies used a variety of programs to estimate models (see Table 1). Fit statistics were the main tool for model selection in all included studies. Bayesian Information criteria (BIC) was used in 20 studies (87%). Nineteen of these studies (83%) used additional criteria, such as Akaike Information Criteria (AIC), sample size adjusted BIC (ssaBIC) Bootstrap Likelihood Ratio Test (BLRT), and Bayes factor. Seven studies (30%) used likelihood ratio difference tests either instead of BIC or in conjunction with it. Sixteen studies (70%) reported using other criteria, such as entropy, interpretability of classes, and model parsimony, to select the best model.
Evidence for the Validity of Identified Classes
The external validity of classes was demonstrated when differential relationships with other factors were identified. Thirteen of the 23 studies (57%) examined whether covariates differentially predicted class membership. Of those, three found that the classes had different antecedents (Basten et al., 2013; Nozadi et al., 2016; Sulik et al., 2017), one found that classes differentially predicted other outcomes (Fergusson et al., 2009), and eight found cross-sectional associations (van Lier et al., 2003a,b; Lanza, 2011; Basten et al., 2013; Bradshaw et al., 2015; Racz et al., 2015; Wall et al., 2016; Carey et al., 2017). All studies found that classes had differential relationships with at least one covariate. Four studies also identified some covariates that did not have different associations with class (van Lier et al., 2003b; Lanza, 2011; Nozadi et al., 2016; Wall et al., 2016). Overall, the results from these 13 studies indicate that LCA produced externally valid classes.
The validity of classes was also indicated by the extent to which they showed practical or theoretical utility. Table 1 indicates whether studies found meaningful classes, by identifying classes which represented either heritable phenotypes or theorized mental health typologies. Studies that found theoretically and practically interesting classes representing subgroups of children who followed different developmental courses, are also indicated, as are those which identified mental health differences among observed groups of children such, as age or sex differences. Classes which had practical utility, in that they classified children into mutually exclusive groups, so that symptom prevalence in the population could be estimated, are also noted. Overall, each study in the review produced classes that served a practical or theoretical purpose, attesting to their validity as meaningful constructs.
Evidence for the Reliability of Identified Classes
Six studies (indicated in Table 1) reported that they had carried out formal statistical tests of measurement invariance to assess whether the same classes were found in different samples. Invariance or partial invariance was found in all cases apart from in one study (Baillargeon et al., 1999), which found that classes of aggression were non-invariant across gender- in other words, the classes were not structurally equivalent for boys and girls.
Ten studies (43%) made informal assessments of whether the same classes were found in different samples (see Table 1). Althoff et al. (2006) found different classes of ADHD behavior in boys compared to girls. However, the other nine studies concluded that classes were generally similar in different samples. Although not as robust as formal tests of measurement invariance, these studies suggest that similar classes can be found in different samples and show a degree of reliability. Only one study made explicit comparisons with the classes found in their study and those found using other samples (Sulik et al., 2017); the authors concluded that their class solution was consistent over time, and with other similar studies.
Overall, 16 out of the 23 studies (70%) carried out the analysis in more than one sample and reliability of classes is generally supported. Seven studies (30%) did not carry out additional analyses so the reliability of classes is unknown.
Discussion
This systematic review identified 23 eligible studies, which conducted 97 LCA analyses of mental health indicators, for children in the general population. The review found that studies had used a range of mental health indicators to study mental health types but few had used the same indicators. Therefore, we did not find a large corpus of similar results for any area of population mental health. Comparing study methodologies and results revealed that there was variation in how the final number of classes were selected and the extent to which the validity or reliability of found classes was demonstrated.
Overview of How LCA Has Been Used to Study Population Mental Health in Children
Each study in the review identified subgroups of children with particular patterns of mental health, thus capitalizing on the fact that LCA is a person-oriented method, which focuses on heterogeneity in the population. It is noteworthy that, even in this small sample of studies, LCA has been applied in different ways. For example, it has been used to classify children into distinct subgroups to investigate population prevalence of symptoms (e.g., Baillargeon et al., 1999), to determine whether subtypes of disorders emerged or whether the difference between children's symptoms was simply quantitative (e.g., Carey et al., 2017), to investigate symptoms expressed in a particular context (e.g., Sulik et al., 2017), and to investigate the comorbidity of distinct disorders (e.g., Wall et al., 2016). Once classes have been derived, these can be included in other analyses, for example to test the heritability of class types (e.g., Vendlinski et al., 2014), how they change over time (e.g., Basten et al., 2016), and what other factors predict them (van Lier et al., 2003a). It is clear that LCA is a useful and flexible statistical tool for researchers interested in researching population mental health in children.
All studies investigated symptoms of psychopathology in the general population and only two studies included some indicators of positive behavior (Bradshaw et al., 2015; Racz et al., 2015). Consequently, the majority of studies found that the no symptom class was the largest. This is consistent with evidence that most children in the general population do not experience mental health difficulties (Sadler et al., 2018). Therefore, it is surprising that 11% of studies found that the no symptom group was not the largest. In those studies, symptomatic groups may have been more prevalent because of cohort differences. It could also be because the measures tested a more normative set of feelings and behaviors. Providing an indication of average responses in the overall sample and for each class could help to interpret results like these better.
Focusing solely on mental health symptoms rather than positive mental health and wellbeing also means that heterogeneity was generally only observed in symptomatic groups. Research indicates that considering a wider range of symptoms and strengths captures a greater range of mental health heterogeneity (Rose et al., 2017; St Clair et al., 2017). Two of the reviewed studies examined a range of behavior from symptomatic to positive, leaving more opportunity for heterogeneity in none symptomatic children to be identified. One of those studies (Bradshaw et al., 2015), did, indeed, find a “better than average functioning” class, suggesting heterogeneity in the non-symptomatic groups. If researchers are interested in heterogeneity across the whole population rather than identifying symptomatic groups alone, LCA can be applied for that purpose. Proponents of the dual-factor model of mental health suggest that a focus on symptoms, at the expense of subjective or psychological wellbeing, fails to identify important groups, such as those that have few symptoms but low levels of wellbeing, or those with many symptoms but high levels of wellbeing (Greenspoon and Saklofske, 2001; Suldo and Shaffer, 2008; Antaramian et al., 2010; Lyons et al., 2012). Therefore, researchers interested in mental health development in general population samples may consider including a broad range of mental health indicators to capture more heterogeneity.
Rather than finding a corpus of studies using similar indicators to measure mental health, the review produced studies which had used an array of indicators. Thus, it is difficult to draw firm conclusions about what mental health classes exist in the population. However, it indicates areas where LCA has been used, and some patterns between findings have been identified. The review highlighted that when studies used indicators for a narrow aspect of mental health, they tended to find classes which differed in severity of symptoms alone. This is unsurprising, as distinct mental health patterns are unlikely to arise when symptoms are very similar in nature. The area of mental health being investigated may also influence what kinds of classes are found. For example, LCA studies that used a range of anxiety types, or anxiety and depression symptoms, found ordinal classes. This suggests that the level of anxiety and depression differentiates children more than the particular types of symptoms experienced. Because only a few studies have looked at this within the general population, further research using different indicators and samples would be needed to ascertain whether pure forms of anxiety or depression are commonly found in children.
In addition, studies that used a range of internalizing and externalizing problems as indicators, tended to find a large no symptoms class, a small multi-morbid class, and then some qualitatively different symptom classes, such as internalizing only, externalizing only, or a specific disorder. This suggests that similar classes are often observed in the general population. However, the particular indicators used can subtly alter the nature of the identified classes. It is, therefore, important for researchers to consider how the indicators used for latent class analysis may enable or restrict which classes are identified.
Differences in Method of Class Selection
Studies included in the review often used different criteria to enumerate classes, reflecting the fact that there is no sole criterion for choosing the most appropriate latent class model (Nylund et al., 2007). The majority of studies in the review used BIC to test the relative fit of two models with different numbers of classes. Lower BIC values indicate better model fit, when parsimony is considered (Collins and Lanza, 2010). When conducting exploratory LCA, the best class solution is found by testing models of increasing numbers of classes and selecting the model with the lowest BIC. This is a useful method recommended by a number of researchers (e.g., Hagenaars and McCutcheon, 2002; Nylund et al., 2007), but, in cases where samples are large, relying on BIC alone can lead to the over extraction of classes (Specht et al., 2014). Three studies in this review (Baillargeon et al., 1999; Hudziak et al., 1999; Wadsworth et al., 2001) did not use BIC at all. Instead, they used chi-square statistics in order to choose between competing latent class models. Chi-square fit statistics test the absolute fit of the model, however, they are not always appropriate for testing different class models in LCA because, when data is sparse (i.e., when there are many possible response patterns with small frequencies) the chi-squared distribution may not be well approximated and, therefore, p-values can be unreliable. Parametric bootstrapping and posterior predictive checks can be used to overcome problems such as these (Nylund et al., 2007; Collins and Lanza, 2010; Masyn, 2013). Sixteen studies reported fit statistics in addition to BIC. Of these, nine reported additional information criteria such as AIC and ssaBIC. Research indicates the power of information criteria to detect the best solution depends on the degree of separation between classes, sample size and the number of indicators used, therefore, examining more than one statistic may be preferable (Tein et al., 2013). Other information criteria may also be used to help enumerate classes such as Consistent Akaike Information Criterion (CAIC) and Approximate Weight of Evidence (AWE) Criterion, although these were not applied in the studies included for review. All information criteria statistics indicate relative model fit when complexity of the model is taken in to account. Lower values indicate better fit, so class solutions with the lowest values are favored (Masyn, 2013; Nylund-Gibson and Choi, 2018). Nine studies also used likelihood-ratio tests (i.e., LMR-LRT and BLRT) to indicate whether adding a class significantly improved model fit. Studies have shown that these fit statistics provide useful additional information when choosing between different class models (Nylund et al., 2007; Tein et al., 2013). For both the LMR-LRT and BLRT, a significant p-value indicates that the model fit is significantly better for the k-class model, than the model with k-1 classes (Nylund et al., 2007). Two reviewed studies also applied Bayes factor to compare the fit of adjacent models. This is a Bayesian method which indicates the strength of support for a model compared to a model with one class more. Scores less than 3 indicate weak support and scores greater than 10 indicate strong support for the more parsimonious model (Nylund-Gibson and Choi, 2018). As research suggests that fit statistics have different strengths and provide different information, which can be used to aid the class enumeration, researchers should be encouraged to use and report multiple fit indices. This was not evident in all reviewed studies, and it is an area for improvement in future research.
While fit statistics may guide model selection, even the use of several fit indices cannot provide a definitive indication of the best model. Indeed, they may contradict each other. Therefore, after fit statistics have been used to narrow down the number of possible models, the substantive meaning of the classes should be considered in order to select the best class solution. For example, one might consider whether classes are in line with what would be expected from theory, whether they are easy they are to interpret, whether classes are large enough to be of interest to the researcher, and whether the solution is parsimonious (Collins and Lanza, 2010; Meeus et al., 2011; Masyn, 2013). Thirteen studies in this review (57%) did not report making any of these considerations when selecting the best class solution, suggesting that opportunities to select the most theoretically and practically meaningful classes might have been missed. Approximately a third of studies (30%) reported using classification quality information to select the best model, such as entropy and mean posterior probability scores. Other studies have also found that entropy is frequently used in the model enumeration process (Tein et al., 2013). Entropy is a measure of overall classification quality. Scores range from zero to one, with the higher value being the better value as far as classification is concerned. Whereas, mean posterior probability scores indicate the certainty to which individuals are assigned to each class. While classification information is useful for understanding the precision of latent class assignment, and should be reported, it does not indicate whether the model is a good fit to the data, and should not be used as a model selection tool (Masyn, 2013). Overall, the results of this review have highlighted that a range of fit indices and considerations about the substantive meaning of classes are being used for class selection in LCA in this specific field. Moving forward, researchers should consider whether they have used all the model selection tools available to them when choosing a final latent class solution.
Clear and detailed reporting of the methods is needed to allow replicability and the critical appraisal of results, because numerous decisions have to be made when selecting the final LCA model (van de Schoot et al., 2017). Quality analysis using the adapted GRoLTS checklist indicated that the majority of studies did report key information, such as the software used for the analysis, fit statistics used for model selection, and plots or charts of the final class solution. Other important aspects were inconsistently reported, such as entropy, and the total number of fitted models with fit indices. In addition, some aspects, which would be necessary for full transparency and to allow replicability, were poorly reported. For example, only a third of studies were clear as to whether they had applied parameter restrictions, and no studies provided the plots or bar charts for all class solutions, or made the syntax available as Supplementary Material. These findings are similar to assessments of the quality of reporting in latent trajectory studies (van de Schoot et al., 2017; see comparison figures included as Supplementary Material S4). Researchers may consider online Supplementary Material as an alternative avenue for displaying such information.
Reliability and Validity of Mental Health Classes for Children in the General Population
In the review, few studies used the same indicators, making it difficult to draw comparisons between results. Where studies had used comparable indicators, some similarities emerged. For example, studies that looked at a broad range of internalizing and externalizing symptoms tended to find classes which represented internalizing, externalizing, and comorbid groups- something which is also found in the wider literature (Lilienfeld, 2003). Studies that looked at physical aggression found low, medium and high symptom groups. Additionally, studies which looked at parent and teacher reports of behavior found- no behavior problems high cross-context behavior problems, and context-specific behavior problem classes. Further research would need to be carried out to see if the same mental health classes are reliably found in different samples, especially where fewer studies have been carried out, such as with anxiety and depressive symptoms.
Many studies in the review demonstrated reliability in the classes they identified by finding the same classes in multiple analyses. However, none of the reviewed studies explicitly stated that the repeated analyses were conducted to test for reliability of classes, and the samples used for each analysis often differed in important ways. Additional analyses were mainly conducted to examine mental health differences in boys and girls or in different age groups. To establish confidence that classes are reliable and not sample specific, researchers should include formal tests of reliability with similar samples; this can be achieved by testing the model in more than one sample and carrying out tests to establish whether the classes are structurally similar, or by testing the model in half of the sample.
Validity of children's mental health classes were demonstrated in a number of ways in the reviewed studies. Each study showed that the classes were meaningful in some sense, however, most did not explicitly test the validity of classes by hypothesizing which classes should be identified and what relationship they would be expected to have with other factors. Identifying expected classes and expected relationships with other factors would indicate that the classes aligned with current theory and research as expected, and it would indicate that the classes were valid. Furthermore, when there is already a strong reason for assuming that there are subgroups of individuals with similar mental health symptoms or strengths, LCA can be applied as a confirmatory approach. By specifying the classes a priori, according to theory, and by setting parameter restrictions on the LCA model, more theoretically robust classes could be identified (Finch and Bronk, 2011; Schmiege et al., 2018). All reviewed studies used exploratory LCA, where different class models were generated and the best fitting model was chosen. Future studies should consider to what extent there is sufficient research evidence and theory for testing specific hypotheses about the classes that may be found. If so, formally testing them using confirmatory methods would be more appropriate.
Strengths, Limitations, and Future Directions
Extensive searches of relevant databases, Google Scholar, and hand-searches were conducted. The search was limited to a 20-year period starting January 1997. Although some earlier studies may have been excluded, it was assumed that the majority of LCA studies in this area were conducted after 1997, since most software packages for LCA analyses were developed after this point (e.g., Muthen and Muthen, 1998-2017; Vermunt and Magidson, 2000; Lanza et al., 2007). A further limitation is that the review only included studies which examined the mental health of children aged between 4 and 11 years and those from the general population. As discussed previously, these restrictions were made in order to allow results to be compared on a like-for-like basis. Further research would need to be carried out to compare the results of studies that had used LCA to examine mental health in other groups.
Despite those limitations, this is the first study to systematically review the application of LCA to investigate subgroups of children with similar mental health symptoms and strengths, in the general population. It has not only compared the results from studies in this area, but also indicated which different model selection methods have been applied and the extent to which studies evaluated evidence for class validity and reliability. In doing so, the review highlights that LCA has been used effectively in the field, although further work is needed to improve the rigor with which the method is applied. A checklist for practitioners or researchers intending to use LCA for the study of population mental health is provided (see Figure 2). This can be used to improve the application of LCA in this area in the future.
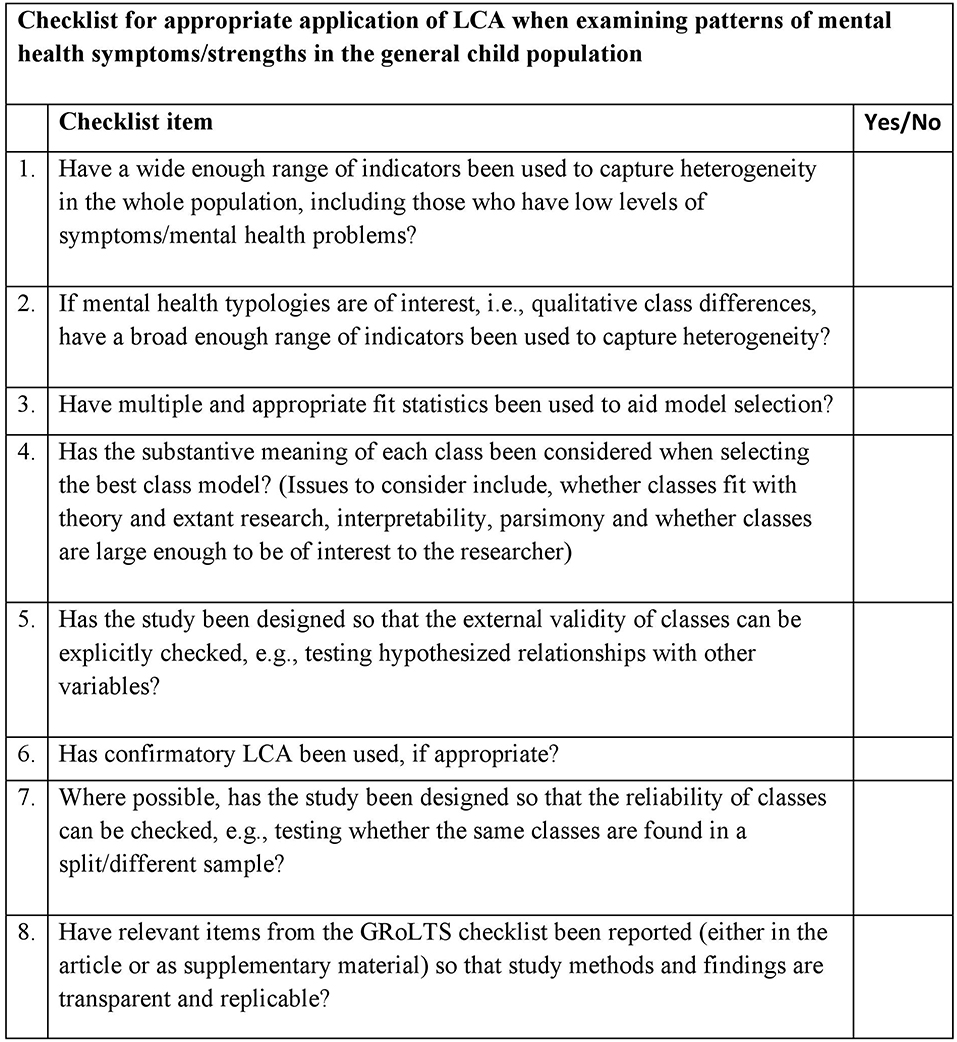
Figure 2. Checklist for appropriate application of LCA, when examining patterns of mental health symptoms/strengths in the general child population.
Author Contributions
All authors contributed to the conception and design of the study. KP devised the search terms, searched the databases, did an initial sort based on paper titles and abstracts, and a further full paper sort of remaining papers, against the inclusion/exclusion criteria. PQ sorted over 20% of papers in the initial title and abstract sorting phase and 100% of papers in the full text sorting phase to ensure consistency in sorting. Any ambiguities in sorting were discussed among all authors. KP carried out data extraction and tabulated the results. KP wrote the manuscript and PQ and NH contributed to manuscript revision. All authors read, and approved the submitted version.
Funding
This paper was completed as part of a PhD funded by the School of Environment, Education and Development, University of Manchester, UK.
Conflict of Interest Statement
The authors declare that the research was conducted in the absence of any commercial or financial relationships that could be construed as a potential conflict of interest.
Supplementary Material
The Supplementary Material for this article can be found online at: https://www.frontiersin.org/articles/10.3389/fpsyg.2019.01214/full#supplementary-material
Footnotes
1. ^Data extraction sheet template available as Supplementary Material S2.
2. ^Adapted GRoLTS checklist available as Supplementary Material S3.
References
Althoff, R. R., Copeland, W. E., Stanger, C., Derks, E. M., Todd, R. D., Neuman, R. J., et al. (2006). The latent class structure of ADHD is stable across informants. Twin Res. Human Genet. 9, 507–522. doi: 10.1375/twin.9.4.507
Althoff, R. R., Rettew, D. C., Boomsma, D. I., and Hudziak, J. J. (2009). Latent class analysis of the child behavior checklist obsessive-compulsive scale. Compr. Psychiatry 50, 584–592. doi: 10.1016/j.comppsych.2009.01.005
American Psychiatric Association (2013). Diagnostic and statistical manual of mental disorders (5th ed). Washington, DC: American Psychiatric Association.
Antaramian, S. P., Scott Huebner, E., Hills, K. J., and Valois, R. F. (2010). A dual-factor model of mental health: toward a more comprehensive understanding of youth functioning. Am. J. Orthopsychiatry 80, 462–472. doi: 10.1111/j.1939-0025.2010.01049.x
Baillargeon, R. H., Tremblay, R. E., and Willms, D. (1999). The Prevalence of Physical Aggression in Canadian Children: A Multi-group Latent Class Analysis of Data From the First Collection Cycle (1994-1995) of the NLSCY. Retrieved from https://ruor.uottawa.ca/bitstream/10393/12870/1/Baillargeon_Raymond_1999_The_prevalence_of_physical_aggression_in_Canadian_children.pdf (accessed November 23, 2018).
Basten, M., Tiemeier, H., Althoff, R. R., van de Schoot, R., Jaddoe, V. W., Hofman, A., et al. (2016). The stability of problem behavior across the preschool years: an empirical approach in the general population. J. Abnorm. Child Psychol. 44, 393–404. doi: 10.1007/s10802-015-9993-y
Basten, M. M., Althoff, R. R., Tiemeier, H., Jaddoe, V. W., Hofman, A., Hudziak, J. J., et al. (2013). The dysregulation profile in young children: empirically defined classes in the Generation R study. J. Am. Acad. Child Adolesc. Psychiatry 52, 841–850. doi: 10.1016/j.jaac.2013.05.007
Bauer, D. J., and Curran, P. J. (2003). Overextraction of latent trajectory classes: much ado about nothing? Reply to Rindskopf (2003), Muthen (2003), and Cudeck and Henly (2003). Psychol. Methods 8, 384–393. doi: 10.1037/1082-989X.8.3.384
Bauer, D. J., and Curran, P. J. (2004). The integration of continuous and discrete latent variable models: potential problems and promising opportunities. Psychol. Methods 9, 3–29. doi: 10.1037/1082-989X.9.1.3
Berlin, K. S., Williams, N. A., and Parra, G. R. (2013). An introduction to latent variable mixture modeling (part 1): overview and cross-sectional latent class and latent profile analyses. J. Pediatr. Psychol. 39, 174–187. doi: 10.1093/jpepsy/jst084
Bradshaw, C. P., Waasdorp, T. E., and Leaf, P. J. (2015). Examining variation in the impact of school-wide positive behavioral interventions and supports: findings from a randomized controlled effectiveness trial. J. Educ. Psychol. 107, 546–557. doi: 10.1037/a0037630
Campbell, S. B., Shaw, D. S., and Gilliom, M. (2000). Early externalizing behavior problems: toddlers and preschoolers at risk for later maladjustment. Dev. Psychopathol. 12, 467–488. doi: 10.1017/S0954579400003114
Carey, E., Devine, A., Hill, F., and Szucs, D. (2017). Differentiating anxiety forms and their role in academic performance from primary to secondary school. PLoS ONE 12:e0174418. doi: 10.1371/journal.pone.0174418
Carter, A. S., Briggs-Gowan, M. J., and Davis, N. O. (2004). Assessment of young children's social-emotional development and psychopathology: recent advances and recommendations for practice. J. Child Psychol. Psychiatry 45, 109–134. doi: 10.1046/j.0021-9630.2003.00316.x
Collins, L., and Lanza, S. T. (2010). Latent Class and Latent Transition Analysis. Hoboken, NJ: Wiley.
DiStefano, C., and Kamphaus, R. (2006). Investigating subtypes of child development: a comparison of cluster analysis and latent class cluster analysis in typology creation. Educ. Psychol. Meas. 66, 778–794. doi: 10.1177/0013164405284033
Dowdy, E., and Kamphaus, R. W. (2007). A comparison of classification methods for use in predicting school-based outcomes. Calif. Sch. Psychol. 12, 121–132. doi: 10.1007/BF03340936
Eccles, J. S., Lord, S. E., and Roeser, R. W. (1996). “Round holes, square pegs, rocky roads, and sore feet: The impact of stage-environment fit on young adolescents' experiences in schools,” in Adolescence: Opportunities and Challenges, eds D. Cicchetti and S. Toth (New York, NY: University of Rochester Press), 47–92.
Fanti, K. A., and Henrich, C. C. (2010). Trajectories of pure and co-occurring internalizing and externalizing problems from age 2 to age 12: findings from the National Institute of Child Health and Human Development Study of Early Child Care. Dev. Psychol. 46, 1159–1175. doi: 10.1037/a0020659
Ferdinand, R. F., Bongers, I. L., van der Ende, J., van Gastel, W., Tick, N., Utens, E., et al. (2006). Distinctions between separation anxiety and social anxiety in children and adolescents. Behav. Res. Ther. 44, 1523–1535. doi: 10.1016/j.brat.2005.11.006
Fergusson, D. M., Boden, J. M., and Horwood, L. J. (2009). Situational and generalised conduct problems and later life outcomes: evidence from a New Zealand birth cohort. J. Child Psychol. Psychiatry 50, 1084–1092. doi: 10.1111/j.1469-7610.2009.02070.x
Finch, W. H., and Bronk, K. C. (2011). Conducting confirmatory latent class analysis using Mplus. Struct. Equation Model. 18, 132–151. doi: 10.1080/10705511.2011.532732
Goodman, R. (2001). Psychometric properties of the strengths and difficulties questionnaire. J. Am. Acad. Child Adolesc. Psychiatry 40, 1337–1345. doi: 10.1097/00004583-200111000-00015
Greenberg, M. T., and Abenavoli, R. (2017). Universal interventions: fully exploring their impacts and potential to produce population-level impacts. J. Res. Educ. Eff. 10, 40–67. doi: 10.1080/19345747.2016.1246632
Greenspoon, P. J., and Saklofske, D. H. (2001). Toward an integration of subjective well-being and psychopathology. Soc. Indic. Res. 54, 81–108. doi: 10.1023/A:1007219227883
Haddaway, N. R., Collins, A. M., Coughlin, D., and Kirk, S. (2015). The role of Google Scholar in evidence reviews and its applicability to grey literature searching. PLoS ONE 10:e0138237. doi: 10.1371/journal.pone.0138237
Hagenaars, J. A., and McCutcheon, A. L. (2002). Applied Latent Class Analysis. Cambridge: Cambridge University Press.
Hicks, B. M., Clark, D. A., and Durbin, C. E. (2017). Person-centered approaches in the study of personality disorders. Personal. Disord. 8, 288–297. doi: 10.1037/per0000212
Hudziak, J. J., Heath, A. C., Madden, P. F., Reich, W., Bucholz, K. K., Slutske, W., et al. (1998). Latent class and factor analysis of DSM-IV ADHD: a twin study of female adolescents. J. Am. Acad. Child Adolesc. Psychiatry, 37, 848–857.
Hudziak, J. J., Wadsworth, M. E., Heath, A. C., and Achenbach, T. M. (1999). Latent class analysis of Child Behavior Checklist attention problems. J. Am. Acad. Child Adolesc. Psychiatry, 38, 985–991. doi: 10.1097/00004583-199908000-00014
Kuny, A. V., Althoff, R. R., Copeland, W., Bartels, M., Van Beijsterveldt, C. E., Baer, J., et al. (2013). Separating the domains of oppositional behavior: comparing latent models of the conners' oppositional subscale. J. Am. Acad. Child Adolesc. Psychiatry 52, 172–183. doi: 10.1016/j.jaac.2012.10.005
Lanza, H. I. (2011). Co-Occurring Oppositional Defiant and Depressive Symptoms: Emotion Dysregulation as an Underlying Process and Developmental Patterns Across Middle Childhood. Doctoral dissertation. Retrieved from ProQuest Dissertations and Theses database. (UMI No. 3408857).
Lanza, S. T., Collins, L. M., Lemmon, D. R., and Schafer, J. L. (2007). PROC LCA: a SAS procedure for latent class analysis. Struct. Equation Model. 14, 671–694. doi: 10.1080/10705510701575602
Lanza, S. T., and Cooper, B. R. (2016). Latent class analysis for developmental research. Child Dev. Perspect. 10, 59–64. doi: 10.1111/cdep.12163
Lee, K. H., Baillargeon, R. H., Vermunt, J. K., Wu, H. X., and Tremblay, R. E. (2007). Age differences in the prevalence of physical aggression among 5-11-year-old Canadian boys and girls. Aggress. Behav. 33, 26–37. doi: 10.1002/ab.20164
Lenzenweger, M. F. (2004). Consideration of the challenges, complications, and pitfalls of taxometric analysis. J. Abnorm. Psychol. 113, 10–23. doi: 10.1037/0021-843X.113.1.10
Lilienfeld, S. O. (2003). Comorbidity between and within childhood externalizing and internalizing disorders: reflections and directions. J. Abnorm. Child Psychol. 31, 285–291. doi: 10.1023/A:1023229529866
Lyons, M. D., Huebner, E. S., Hills, K. J., and Shinkareva, S. V. (2012). The dual-factor model of mental health: further study of the determinants of group differences. Can. J. Sch. Psychol. 27, 183–196. doi: 10.1177/0829573512443669
MacCallum, R. C., Zhang, S., Preacher, K. J., and Rucker, D. D. (2002). On the practice of dichotomization of quantitative variables. Psychol. Methods 7, 19–40. doi: 10.1037/1082-989X.7.1.19
Masyn, K. E. (2013). “Latent class analysis and finite mixture modeling,” in The Oxford Handbook of Quantitative Methods, ed T. D. Little (New York, NY: Oxford University Press), 551–611.
McElroy, E., Shevlin, M., and Murphy, J. (2017). Internalizing and externalizing disorders in childhood and adolescence: a latent transition analysis using ALSPAC data. Compr. Psychiatry 75, 75–84. doi: 10.1016/j.comppsych.2017.03.003
Meeus, W., Van de Schoot, R., Klimstra, T., and Branje, S. (2011). Personality types in adolescence: change and stability and links with adjustment and relationships: a five-wave longitudinal study. Dev. Psychol. 47, 1181–1195. doi: 10.1037/a0023816
Miettunen, J., Nordström, T., Kaakinen, M., and Ahmed, A. (2016). Latent variable mixture modeling in psychiatric research–a review and application. Psychol. Med. 46, 457–467. doi: 10.1017/S0033291715002305
Moffitt, T. E. (1993). Adolescence-limited and life-course persistent anti- social behavior: a developmental taxonomy. Psychol. Rev. 100, 674–701. doi: 10.1037/0033-295X.100.4.674
Muthen, L., and Muthen, B. (1998-2017). Mplus User's Guide, 8th Edn. Los Angeles, CA: Muthén Muthén.
Nozadi, S. S., Troller-Renfree, S., White, L. K., Frenkel, T., Degnan, K. A., Bar-Haim, Y., et al. (2016). The moderating role of attention biases in understanding the link between behavioral inhibition and anxiety. J. Exp. Psychopathol. 7, 451–465. doi: 10.5127/jep.052515
Nylund, K. L., Asparouhov, T., and Muthén, B. O. (2007). Deciding on the number of classes in latent class analysis and growth mixture modeling: a monte carlo simulation study. Struct. Equation Model. 14, 535–569. doi: 10.1080/10705510701575396
Nylund-Gibson, K., and Choi, A. Y. (2018). Ten frequently asked questions about latent class analysis. Transl. Issues Psychol. Sci. 4, 440–461. doi: 10.1037/tps0000176
Racz, S. J., O'Brennan, L. M., Bradshaw, C. P., and Leaf, P. J. (2015). The influence of family and teacher factors on early disruptive school behaviors: a latent profile transition analysis. J. Emot. Behav. Disord. 24, 67–81. doi: 10.1177/1063426615599541
Romano, E., Baillargeon, R. H., and Tremblay, R. E. (2002). Prevalence of Hyperactivity-Impulsivity and Inattention Among Canadian Children: Findings from the First Data Collection Cycle (1994-1995) of the National Longitudinal Survey of Children and Youth. Final Report. Retrieved from https://files.eric.ed.gov/fulltext/ED505317.pdf (accessed November 23, 2018).
Rose, T., Lindsey, M. A., Xiao, Y., Finigan-Carr, N. M., and Joe, S. (2017). Mental health and educational experiences among black youth: a latent class analysis. J. Youth Adolesc. 46, 2321–2340. doi: 10.1007/s10964-017-0723-3
Sadler, K., Vizard, T., Ford, T, Marcheselli, F., Pearce, N., et al. (2018). Mental Health of Children and Young People in England, 2017: Summary of Key Findings. Retrieved from NHS Digital website: https://files.digital.nhs.uk/F6/A5706C/MHCYP%202017%20Summary.pdf (accessed January 4, 2019).
Schmiege, S. J., Masyn, K. E., and Bryan, A. D. (2018). Confirmatory latent class analysis: illustrations of empirically driven and theoretically driven model constraints. Org. Res. Methods 21, 983–1001. doi: 10.1177/1094428117747689
Scotto Rosato, N., and Baer, J. C. (2012). Latent class analysis: a method for capturing heterogeneity. Soc. Work Res. 36, 61–69. doi: 10.1093/swr/svs006
Specht, J., Luhmann, M., and Geiser, C. (2014). On the consistency of personality types across adulthood: latent profile analyses in two large-scale panel studies. J. Pers. Soc. Psychol. 107, 540–556. doi: 10.1037/a0036863
St Clair, M. C., Neufeld, S., Jones, P. B., Fonagy, P., Bullmore, E. T., Dolan, R. J., et al. (2017). Characterising the latent structure and organisation of self-reported thoughts, feelings and behaviours in adolescents and young adults. PLoS ONE 12:e0175381. doi: 10.1371/journal.pone.0175381
Sterba, S. K., and Bauer, D. J. (2010). Matching method with theory in person-oriented developmental psychopathology research. Dev. Psychopathol. 22, 239–254. doi: 10.1017/S0954579410000015
Suldo, S. M., and Shaffer, E. J. (2008). Looking beyond psychopathology: the dual-factor model of mental health in youth. Sch. Psych. Rev. 37, 52–68.
Sulik, M. J., Blair, C., and Greenberg, M. (2017). Child conduct problems across home and school contexts: a person-centered approach. J. Psychopathol. Behav. Assess. 39, 46–57. doi: 10.1007/s10862-016-9564-8
Tein, J.-Y., Coxe, S., and Cham, H. (2013). Statistical power to detect the correct number of classes in latent profile analysis. Struct. Equation Model. 20, 640–657. doi: 10.1080/10705511.2013.824781
van de Schoot, R., Sijbrandij, M., Winter, S. D., Depaoli, S., and Vermunt, J. K. (2017). The GRoLTS-checklist: guidelines for reporting on latent trajectory studies. Struct. Equation Model. 24, 451–467. doi: 10.1080/10705511.2016.1247646
van Lier, P. A., Verhulst, F. C., and Crijnen, A. A. (2003a). Screening for disruptive behavior syndromes in children: the application of latent class analyses and implications for prevention programs. J. Consult. Clin. Psychol. 71, 353–363. doi: 10.1037/0022-006X.71.2.353
van Lier, P. A., Verhulst, F. C., van der Ende, J., and Crijnen, A. A. (2003b). Classes of disruptive behaviour in a sample of young elementary school children. J. Child Psychol. Psychiatry 44, 377–387. doi: 10.1111/1469-7610.00128
Vendlinski, M. K., Javaras, K. N., Van Hulle, C. A., Lemery-Chalfant, K., Maier, R., Davidson, R. J., et al. (2014). Relative influence of genetics and shared environment on child mental health symptoms depends on comorbidity. PLoS ONE 9:e103080. doi: 10.1371/journal.pone.0103080
Vermunt, J. K., and Magidson, J. (2000). Latent Gold: User's Manual. Boston, MA: Statistical Innovations Inc.
Vermunt, J. K., and Magidson, J. (2002). “Latent class cluster analysis,” in Applied Latent Class Analysis, eds J. A. Hagenaars and A. L. McCutcheon (Cambridge, UK: Cambridge University Press), 89–106.
von Eye, A., and Bergman, L. R. (2003). Research strategies in developmental psychopathology: dimensional identity and the person-oriented approach. Dev. Psychopathol. 15, 553–580. doi: 10.1017/S0954579403000294
Wadsworth, M. E., Hudziak, J. J., Heath, A. C., and Achenbach, T. M. (2001). Latent class analysis of child behavior checklist anxiety/depression in children and adolescents. J. Am. Acad. Child Adolesc. Psychiatry 40, 106–114. doi: 10.1097/00004583-200101000-00023
Keywords: latent class analysis, latent profile analysis, LCA, LPA, mental health, child, systematic review
Citation: Petersen KJ, Qualter P and Humphrey N (2019) The Application of Latent Class Analysis for Investigating Population Child Mental Health: A Systematic Review. Front. Psychol. 10:1214. doi: 10.3389/fpsyg.2019.01214
Received: 18 January 2019; Accepted: 08 May 2019;
Published: 29 May 2019.
Edited by:
Stéphane Bouchard, Université du Québec en Outaouais, CanadaReviewed by:
Hugo Cogo-Moreira, Federal University of São Paulo, BrazilWasantha Jayawardene, Indiana University Bloomington, United States
Copyright © 2019 Petersen, Qualter and Humphrey. This is an open-access article distributed under the terms of the Creative Commons Attribution License (CC BY). The use, distribution or reproduction in other forums is permitted, provided the original author(s) and the copyright owner(s) are credited and that the original publication in this journal is cited, in accordance with accepted academic practice. No use, distribution or reproduction is permitted which does not comply with these terms.
*Correspondence: Kimberly J. Petersen, kimberly.petersen@manchester.ac.uk