- 1Research Coordination and Support Service, Istituto Superiore di Sanità, Rome, Italy
- 2Autism Research Unit, Villa Santa Maria Institute, Tavernerio, Italy
- 3IRCCS Fondazione Stella Maris, Pisa, Italy
- 4Department of Clinical and Experimental Medicine, University of Pisa, Pisa, Italy
Motor disturbances have been widely observed in children with autism spectrum disorder (ASD), and motor problems are currently reported as associated features supporting the diagnosis of ASD in the current Diagnostic and Statistical Manual of Mental Disorders (DSM-5). Studies on this issue reported disturbances in different motor domains, including both gross and fine motor areas as well as coordination, postural control, and standing balance. However, they failed to clearly state whether motor impairments are related to demographical and developmental features of ASD. Both the different methodological approaches assessing motor skills and the heterogeneity in clinical features of participants analyzed have been implicated as contributors to variance in findings. However, the non-linearity of the relationships between variables may account for the inability of the traditional analysis to grasp the core problem suggesting that the “single symptom approach analysis” should be overcome. Artificial neural networks (ANNs) are computational adaptive systems inspired by the functioning processes of the human brain particularly adapted to solving non-linear problems. This study aimed to apply the ANNs to reveal the entire spectrum of the relationship between motor skills and clinical variables. Thirty-two male children with ASD [mean age: 48.5 months (SD: 8.8); age range: 30–60 months] were recruited in a tertiary care university hospital. A multidisciplinary comprehensive diagnostic evaluation was associated with a standardized assessment battery for motor skills, the Peabody Developmental Motor Scale-Second Edition. Exploratory analyses were performed through the ANNs. The findings revealed that poor motor skills were a common clinical feature of preschoolers with ASD, relating both to the high level of repetitive behaviors and to the low level of expressive language. Moreover, unobvious trends among motor, cognitive and social skills have been detected. In conclusion, motor abnormalities in preschoolers with ASD were widespread, and the degree of impairment may inform clinicians about the severity of ASD core symptoms. Understanding motor disturbances in children with ASD may be relevant to clarify neurobiological basis and ultimately to guide the development of tailored treatments.
Introduction
According to the Diagnostic and Statistical Manual of Mental Disorders, fifth edition (DSM-5) (American Psychiatric Association [APA], 2013), autism spectrum disorders (ASD) are a heterogeneous set of neurodevelopmental disorders characterized by deficits in social communication and social interaction along with the presence of restricted and repetitive behaviors. Motor impairments have been widely reported in children with ASD (Fournier et al., 2010), but it is still unclear whether they are represented across the spectrum and whether they are related to the clinical specifiers (i.e., intelligence, language, comorbidity, and associated conditions). The development of reliable motor skills is considered an essential component to enhance communication and social engagement (Iverson, 2010; Soska et al., 2010; Bhat et al., 2011), and motor dysfunctions may influence social development interfering with opportunities for social experiences and social learning (Sacrey et al., 2014). Also, it has been suggested that the impairment in motor skills in children with ASD may also impact their others’ actions understanding (Gallese et al., 2013). Thus, investigating the nature of motor problems in ASD may bring a new perspective into diagnostic and treatment approaches. Indeed, motor impairments have been identified in children with ASD at an early stage of development (Esposito and Venuti, 2008; Phagava et al., 2008; Esposito et al., 2009, 2011; Bhat et al., 2011; Landa et al., 2013; Gima et al., 2018), and a growing research interest has been directed toward investigating the effects of motor intervention on both core and associated symptoms of ASD (Brand et al., 2015; Bremer et al., 2015; Ketcheson et al., 2017; Pan et al., 2017; Bishop and Pangelinan, 2018; Najafabadi et al., 2018).
The motor deficits occur more frequently in children with ASD than in children with typical development (TD) (Fournier et al., 2010; Moseley and Pulvermüller, 2018). Moreover, motor problems are currently reported as associated features supporting the diagnosis of ASD (American Psychiatric Association [APA], 2013). To date, impairments have been highlighted in various motor domains including both gross and fine motor areas, motor coordination, postural control and standing balance (Fournier et al., 2010; Esposito et al., 2011; Longuet et al., 2012; Travers et al., 2013; May et al., 2016). The high variance in findings may suggest a broad spectrum of motor impairments. However, it should be noted that various methodological approaches in different studies could play a role in the heterogeneity of results.
First, different studies have examined motor skills through various instruments (Wilson et al., 2018) including home-video analysis (Gernsbacher et al., 2008; Ozonoff et al., 2008; Phagava et al., 2008; Zappella et al., 2015), parent reports (Kopp et al., 2010; LeBarton and Iverson, 2013; Hedgecock et al., 2018), developmental tests (Landa and Garrett-Mayer, 2006; Landa et al., 2013; Libertus et al., 2014), specific motor batteries as the Movement Assessment Battery for Children (Green et al., 2009; Whyatt and Craig, 2012) and the Peabody Developmental test (Provost et al., 2007; Jasmin et al., 2009; Fulceri et al., 2015), kinematic analysis during walking or prehension movement (Glazebrook et al., 2006; Campione et al., 2016; Eggleston et al., 2017) and electronic balance board (Travers et al., 2013; Stins et al., 2015).
Secondly, differences observed in clinical and demographical features of ASD individuals between studies should be mentioned. In fact, some studies enrolled infants and young children (Landa and Garrett-Mayer, 2006; Provost et al., 2007; Phagava et al., 2008; Esposito et al., 2009; Jasmin et al., 2009), whereas others involved school-aged children, adolescents, and adults (Minshew et al., 2004; Green et al., 2009; Staples and Reid, 2010). Moreover, some studies enrolled children without intellectual disabilities (MacDonald et al., 2013; Miller et al., 2014) whereas others enlisted children with a wide range of cognitive functioning (Vanvuchelen et al., 2007; Green et al., 2009; Fulceri et al., 2015). In this regard, it has been reported that intellectual functioning may be related to motor skills, with low performances associated with reduced motor skills (Mari et al., 2003; Dewey et al., 2007; Dziuk et al., 2007; Vanvuchelen et al., 2007; Dowell et al., 2009; Green et al., 2009; Hilton et al., 2012; Fulceri et al., 2015). However, the largest meta-analysis on this issue to date (Fournier et al., 2010) was unable to unequivocally state the impact of intellectual functioning on motor skills in individuals. Analogously, the relationship between motor impairments and language abilities in children with ASD has not been yet clarified. The observation of motor impairments in children with poor language skills has been related to the coexistence of intellectual impairment (Moseley and Pulvermüller, 2018). However, it should be noted that studies on infants at high familial risk for ASD (infants who have an older sibling with an ASD diagnosis) revealed that the level of fine motor skills in the first years of life was able to predict the language development (LeBarton and Iverson, 2013; Garrido et al., 2017). The interconnection between motor and language skills during development has been clearly defined in children with TD (Libertus and Violi, 2016), and this evidence may be relevant also for children with ASD (Kim, 2008; Bedford et al., 2016; Srinivasan and Bhat, 2016). Finally, the degree of ASD severity has been found to be related to the severity of motor problems (Jasmin et al., 2009; Hilton et al., 2012; MacDonald et al., 2013; Purpura et al., 2016), even if contrasting data have been reported (Zachor et al., 2010; Fulceri et al., 2015).
Thirdly, the traditional statistical analysis approach performed in the studies mentioned above suffered from some criticisms. Indeed, due to the heterogeneity in clinical expression of ASD (i.e., different degree of symptoms severity along with various co-morbidities), it could be possible that traditional “single symptom approach analysis” did not provide comprehensive information. Artificial neural networks (ANNs) are computational adaptive systems inspired by the functioning processes of the human brain particularly adapted to solve non-linear problems (Krogh, 2008; Manning et al., 2014) and to discover unobvious trends and associations among variables. Based on their learning through an adaptive way (i.e., extracting from the available data the information needed to gather a specific task and to generalize the acquired knowledge), the ANNs appear to be a powerful tool for data analysis also in the presence of relatively small samples (Buscema et al., 2015).
In the last years, the ANNs have been increasingly used in medicine (Cocchi et al., 2008; Street et al., 2008; Ayer et al., 2013; Gironi et al., 2013; Peixoto et al., 2015; Toscano et al., 2017), and recently they have also been applied to the ASD field (Buscema et al., 2015; Narzisi et al., 2015; Djemal et al., 2017; Grossi et al., 2017, 2018). Overall, literature findings suggest that the ANNs method may be a strategic approach to grasp the core of the relationship between motor impairments and clinical and developmental features. Therefore, this study aimed to explore the associations between motor skills and clinical/developmental features in a sample of preschoolers with ASD through the ANNs. We hypothesized that the ANNs approach would be able to reveal latent connections among the full spectrum of clinical/developmental variables revealing their simultaneous connections with different domain or degrees of motor impairment.
Materials and Methods
Participants
Participants were a selected subset of a group children recruited at ASD unit at the IRCCS Fondazione Stella Maris, a tertiary care university hospital. Each child received a clinical diagnosis of autistic disorder (AD) or pervasive developmental disorder not otherwise specified (PDD-NOS), based on DSM-IV-TR criteria (American Psychiatric Association [APA], 2000) after several days of extensive and comprehensive evaluation. The diagnosis was confirmed by Autism Diagnostic Observation Schedule (ADOS-G; Lord et al., 2000) and all children satisfy criteria for a diagnosis of ASD according to DSM-5 (American Psychiatric Association [APA], 2013). Exclusion criteria were: (a) neurological syndromes or focal neurological signs; (b) significant sensory impairment (e.g., blindness, deafness); (c) anamnesis of severe birth asphyxia, head injury or epilepsy; (d) potential secondary causes of ASD revealed by high-resolution karyotyping, DNA analysis of Fragile-X, or screening tests for inborn errors of metabolism. The demographical and clinical features of the original sample have been previously published (Fulceri et al., 2015). For this study, incomplete data have been excluded, and analyses have been limited to data from children with non-verbal IQ ≥ 70. The final sample consisted of 32 male preschoolers with a diagnosis of ASD and with non-verbal IQ ≥ 70 (age range: 30–60 months) who were evaluated through a standardized assessment battery for the gross and fine motor skills: the Peabody Developmental Motor Scale-Second Edition (PDMS-2; Folio and Fewell, 2000).
It should be noted that the characteristics of this sample substantially overlapped with those of the sample considered in Fulceri et al. (2015). Descriptive statistics (box and whisker plot) of variables on the study are presented in Figure 1. Table 1 summarized the demographical and clinical features of the sample.
This study was carried out following the recommendations of the IRCCS Stella Maris Foundation. The Ethics Committee of IRCCS Stella Maris Foundation approved the study and the head of the ASD unit at the IRCCS Fondazione Stella Maris supervised the project activities. All parents/guardians gave written informed consent in accordance with the Declaration of Helsinki.
Instruments
Motor Assessment
The PDMS-2 is a standardized assessment battery for gross and fine motor skills. This test consists of six subscales: Reflexes, Stationary, Locomotion, Object Manipulation, Grasping, and Visual-Motor Integration. The combination of the results in these subscales provides three quotients: Gross Motor Quotient (Gross MQ), Fine Motor Quotient (Fine MQ), and Total Motor Quotient (Total MQ). Subscales and quotients scores are classified into seven categories: very superior, superior, above average, average, below average, poor, and very poor. At the time of the study, the Italian version of PDMS-2 has not been yet officially edited. Thus, members of the research team who individually translated the source text of the English version of the PDMS-2 and sought agreement between own translations have elaborated an experimental and un-official Italian version of PDMS-2 used in this study. To date, an official Italian version of this test has been provided by Biancotto et al. (2017).
Autism Diagnostic Measures
The ADOS-G is a semi-structured assessment to rate the child’s social-communicative impairment and restricted and repetitive behaviors. In this study, only Module 1 (pre-verbal/single words/simple phrases) and Module 2 (flexible phrase speech) were applied. The social affect score (ADOS-SA) and the restricted, repetitive behaviors score (ADOS-RRB) were calculated according to published algorithms (Gotham et al., 2009; Hus Bal and Lord, 2015). According to ADOS scoring, the low scores of ADOS are the expression of less impairment while high scores correspond to a higher impairment.
Language Abilities
The level of language abilities of children was evaluated according to the module of ADOS used to assess ASD symptoms. Indeed, the module of ADOS is selected according to the language level of the child. In detail, Module 1 is selected for children who do not use spontaneous phrase speech consistently (defined as non-echoed). Three-word utterances that sometimes involve a verb and that are spontaneous, meaningful word combinations. Module 2 is selected for children with some flexible phrase speech who are not verbally fluent. The operational definition of verbal fluency is the spontaneous, flexible use of sentences with multiple clauses that describe logical connections within a sentence. It requires the ability to talk about objects or events not immediately present (Lord et al., 2000).
Cognitive/Developmental Abilities
Several standardized tests (i.e., Leiter International Performance Scale-Revised, Roid and Miller, 1997; Griffiths Mental Development Scale-ER, Luiz et al., 2006; Wechsler Preschool and Primary Scale of Intelligence, Wechsler, 1974) were used to assess intellectual abilities according to clinical features (i.e., age, language level) of children. For this study, only non-verbal IQ scores were considered.
Motor Treatment History
Information about psychomotor treatment history were evaluated according to the following “yes/no” parent question: “Has your child ever received a psychomotor intervention?” The psychomotor intervention is an approach for child’s rehabilitation widely used in Italian Care Service for children with ASD. This approach focuses on child’s global development promoting awareness of personal space, sharing a common space during play situations, increasing of attention and development of social interaction. Body and movements are considered principal mediators between child and surrounding. University courses in Italy, train therapists in theoretical framework and techniques of psychomotor intervention.
Data Analysis
Linear Correlation Analysis
Pearson correlation analysis was performed between the motor variables (Stationary, Locomotion, Object Manipulation, Grasping and Visual-Motor Integration, Gross MQ, Fine MQ, Total MQ) and the demographical/clinical variables (chronological age, ADOS-SA, ADOS-RRB, ADOS Module 2; ADOS Module 1, Motor Intervention). A p-value of <0.05 was considered. Statistical analysis was performed with SPSS package.
Even if recommended in conducting multiple analyses on the same dependent variable, in this study the linear correlation analysis involved multiple comparisons has been performed without considering the Bonferroni correction. Indeed, being an exploratory analysis looking for important associations among many independent variables, there is no oblige to apply this type of adjustment. While the number of correlations among N independent variables and a dependent variable is equal to N - 1, in case of absence of a dependent variable the number of correlations among all independent variables tends to increase exponentially with their number (N∗N - 1/2) penalizing inevitably the significance of correlation indexes even when they are substantially high.
To limit the false discovery rate, the procedure described in Benjamini and Yekutieli (2001) has been applied obtaining that all r-values above 0.34 reported in the Table 2 remain significant.
Artificial Neural Networks Analysis
General Description
The detailed description of Auto-CM was provided in the Supplementary Material. The Auto Contractive Map (Auto-CM) system is a fourth generation unsupervised ANNs which has already been demonstrated to outperform several other unsupervised algorithms in a heterogeneous class of tasks (Buscema and Sacco, 2017).
Through a specific learning algorithm, the Auto-CM system detects a square matrix of “similarities” (named weights) among the variables considered. Once the Auto-CM weights matrix is obtained, it is then filtered by a minimum spanning tree algorithm (Kruskal, 1956; Fredman and Willard, 1990). The minimum spanning tree shows among the full spectrum of possible ways to connect the variables in a tree, the shortest combination. Based on the minimum spanning tree theory, the Auto-CM reveals the connections among variables providing a graph in which the distances among variables reflect their bonding strength (weights) (Buscema and Grossi, 2008; Buscema et al., 2008). A detailed description of the minimum spanning tree concept is provided in the Supplementary Material.
This data-mining model aims to detect hidden trends and unobvious associations among variables. Indeed, this algorithm provides a semantic connectivity map in which non-linear associations are preserved, and explicit connection schemes are described. This approach provides the map of relevant connections between and among variables and the principal hubs of the system. Hubs can be defined as variables with the maximum amount of connections in the map. The Auto-CM does not pose the initial weights randomly. Conversely, the Auto-CM starts with the same value. Thus, the resulting graph is reproducible along many runs. In other words, the Auto-CM ‘spatializes’ the correlation among the variables (‘closeness’) and the graph identifies only the relevant associations organizing them into a coherent picture. The “central node” is the inner node that remains after bottom-up recursively pruning away the “leaves” nodes.
Artificial Neural Networks Applied to Our Dataset
The 12 continuous variables (Total MQ, Gross MQ, Fine MQ, Stationary, Locomotion, Object Manipulation, Grasping, Visual-Motor Integration, Non-verbal IQ, ADOS-SA, ADOS-RRB, Age) and the two nominals (ADOS Module, Motor Intervention) were included.
In pre-processing, each continuous variable was transformed in two variables either named “high” and “low” based on the median score of each variable. This pre-processing was necessary to evidence the connections of each variable when their values were high or low (that is above or below the median score of each variable). Consequently, the projection of the original variable occupied two positions in the space: one related to high values and the other related to low values. In the map, these two conditions were named “high” and “low.” It should be noted that in non-linear systems, the position of a variable according to its high and low values is not necessarily symmetric in the map.
For the nominal variables, we merely provided the following three variables: Module 1, Module 2, and Motor Intervention.
Benchmarking Analysis
The Auto-CM and the self-organizing maps are systems for unsupervised data mining. However, it should be noted that the self-organizing maps are mainly directing the clustering individuals rather than variables. To handle a benchmarking analysis the principal component analysis (PCA) and hierarchical agglomerative clustering (HAC) were carried out with XLSTAT package 2018. In detail, PCA is mathematically defined as an orthogonal linear transformation that transforms the data to a new coordinate system such that the greatest variance by any projection of the data comes to lie on the first coordinate (called the first principal component), the second greatest variance on the second coordinate, and so on. PCA is theoretically the optimum transform for given data in least square terms. AHC is one of the most popular clustering methods which seeks to build a hierarchy of clusters with a “bottom-up” approach: each observation starts in its own cluster, and pairs of clusters are merged as one moves up the hierarchy. This method works from the dissimilarities between the objects to be grouped together producing the so-called “dendrogram,” which shows the progressive grouping of the data. It is then possible to gain an idea of a suitable number of classes into which the data can be grouped.
Results of this benchmarking analysis allowed us to compare findings from the Auto-CM approach with findings from the traditional statistical approach.
Results
Motor Skills According to the PDMS-2 (Figure 2)
Total QM, Gross QM, Fine QM mean scores were into the “Poor class”; any child had individual Total QM score in the Average class; one child and two children had, respectively, Gross QM and Fine QM in the Average class. Almost all subscales mean scores were into the “Below Average class” or the “Poor class.”
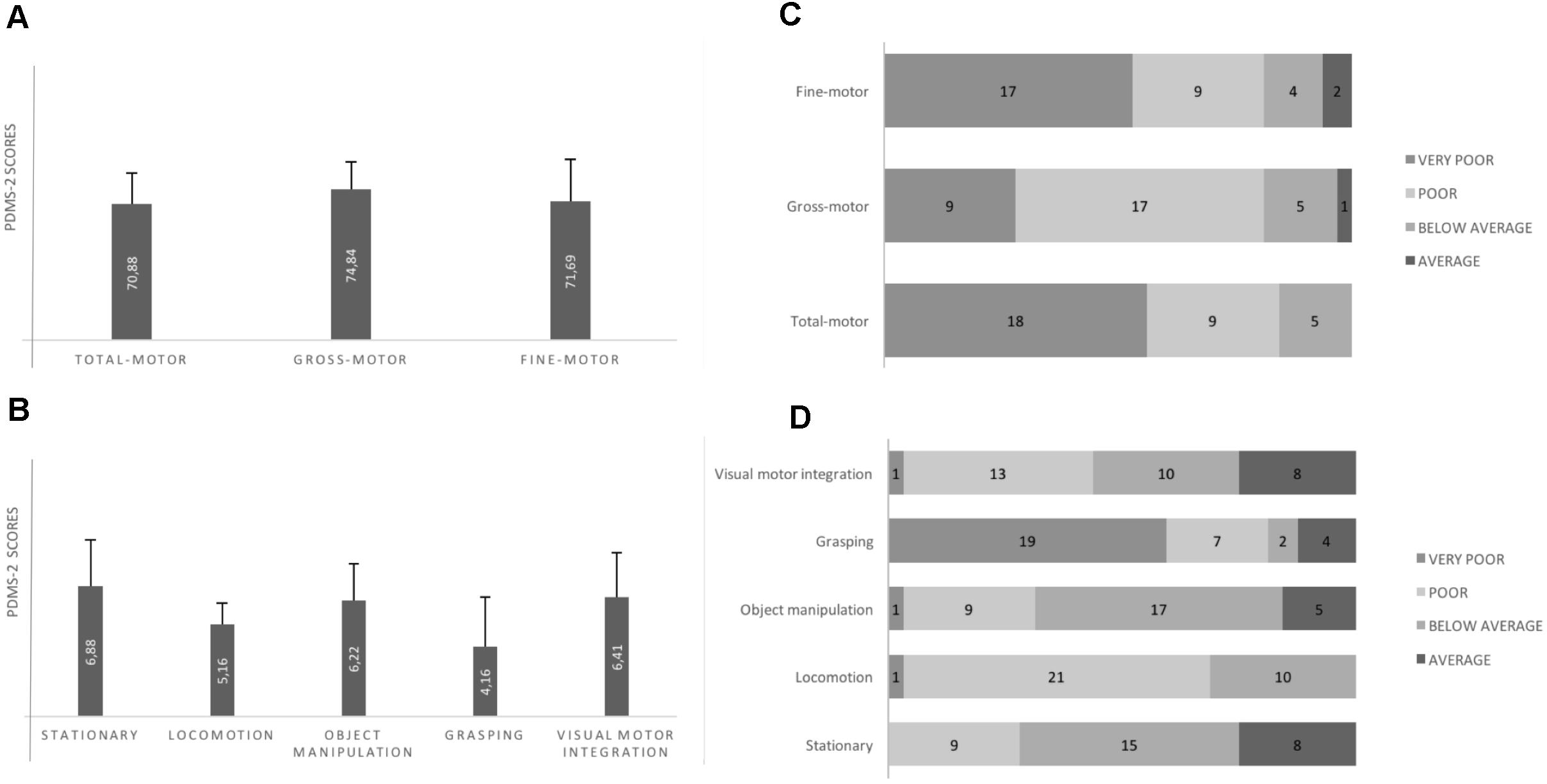
Figure 2. Motor performance according to PDMS-2. (A) All quotient mean scores are into the Poor class (standard scores for the Poor class range from 70 to 79). (B) Locomotion and Grasping subscales scores are into the Poor class (standard scores for the Poor class range from 4 to 5); Static, Object Manipulation and Visuo-Motor Integration are into the Below Average class (standard scores for Below Average class range from 6 to 7). (C) Individual motor quotients: any child shows total motor quotient in the Average class (standard scores for Average class range from 8 to 12. (D) Individual motor subscales: Grasping and Locomotion subscales (i.e., the more impaired subscales according to the mean scores of the sample) appear to be differently impaired. Any child shows Locomotion abilities into the Average class; many children show Grasping abilities into the Very Poor class (standard scores for the Very Poor class range from 1 to 3). Error bars are standard deviation.
Linear Correlation Analysis
Motor quotients (Total QM and Fine QM) were negatively correlated with “ADOS-RRB.” Motor quotients (Total QM and Fine QM) and motor subscales (Grasping and Visual-Motor Integration) were positively correlated with “Non-verbal IQ.” Stationary subscores were negatively correlated with chronological age. Results are summarized in Table 2.
Artificial Neural Networks Analysis (Figure 3)
The graph in Figure 3 described the central node of the network (ADOS Module 1) and two main areas. The upper area was characterized by values indicating a less impaired level of motor functioning (named “high”), where Total MQ high and Fine MQ high variables acted as hubs. Total MQ high coordinated a cluster of motor variables (locomotor high, stationary high, grasping high, object manipulation high) while Fine MQ high was related to following variables: visual-motor integration high, ADOS RRB low, and motor intervention.
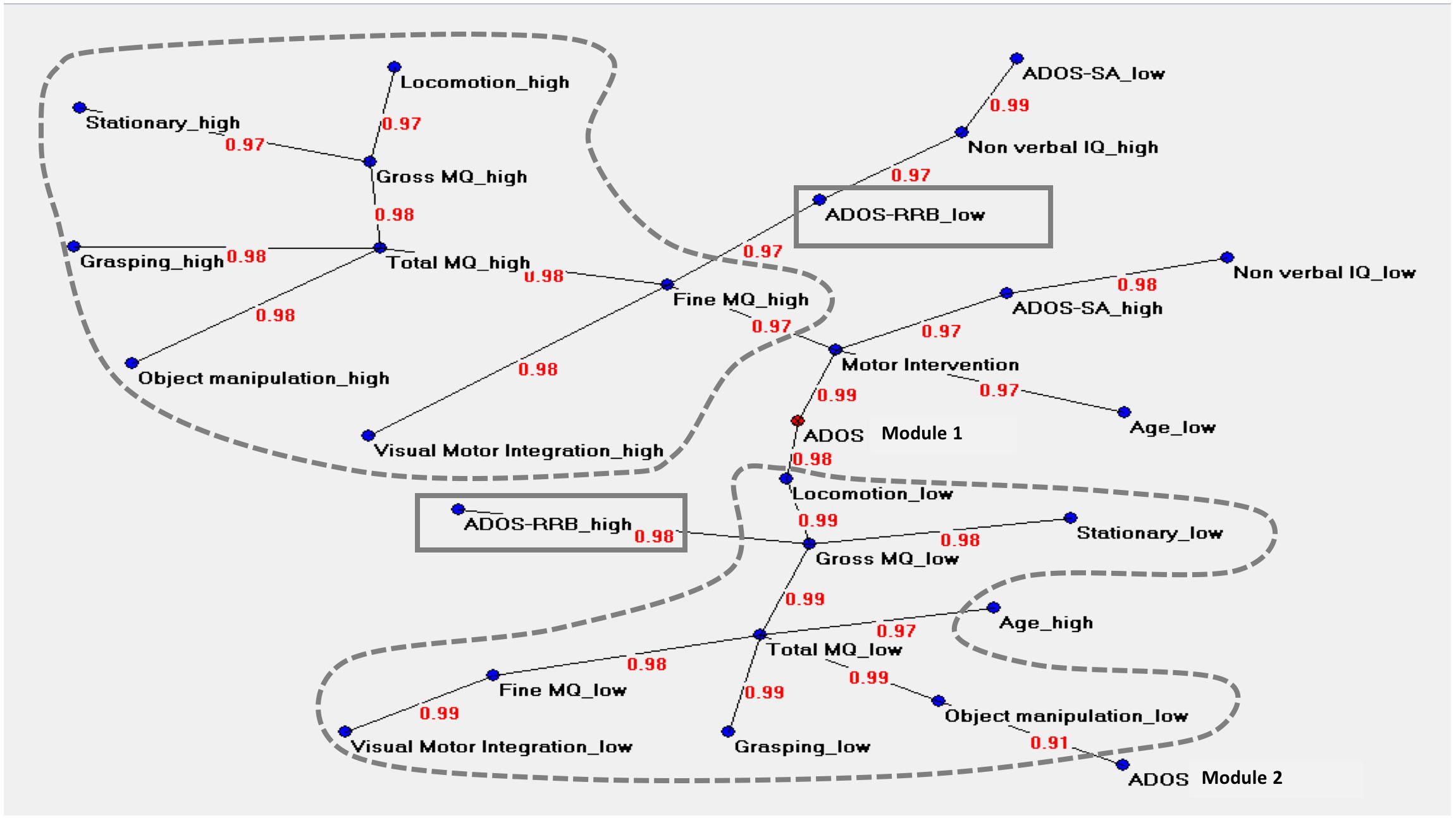
Figure 3. Auto neural networks map. The figure shows the relevant connections among the variables. Each variable is described by two different forms named “high” and “low,” i.e., above or below the median score of each variable. The variable ‘ADOS Module 1’ is the central node of the network. The motor variables indicating a very low level of motor functioning (named “low”) or a less impaired level of motor functioning (named “high”) are strongly connected between them; the two clusters are signed with dotted lines. The upper area is characterized by high values in motor functioning, where ‘Total MQ high’ and ‘Fine MQ high’ act as hubs. ‘Total MQ high’ coordinates a cluster of motor high variables (locomotor, stationary, grasping, object manipulation) while ‘Fine MQ’ is related to ‘Visual-Motor Integration high’ on one side and with ‘ADOS RRB low’ on the other side. This cluster is located close to the variables indicating a high level of cognitive skills and high social functioning. The lower area shows an analog structure of interconnections in opposite sense. ‘Total MQ low’ is the principal hub; ‘ADOS RRB high’ is directly connected with ‘Gross MQ low.’ ‘Total MQ low’ related to the high chronological age. The clinical variables ‘ADOS-SA’ (“high” and “low”) and ‘Non-verbal IQ’ (“high” and “low”) are not directly connected with any motor variables. The variable ‘Age low’ is directly connected with the variable ‘Motor Intervention.’ The variable ‘Motor Intervention’ is located at approximately the same distance between the two-motor clusters. The link strength’s values are presented in red.
The lower area of the graph showed an analog structure of interconnections in the opposite sense. The motor variables indicating a more impaired level of motor functioning (named “low”) were strongly connected between them. Total MQ low was the principal hub of this cluster of variables. This cluster was also directly related with the following clinical variables: ADOS Module 1, Age high and ADOS Module 2. The ADOS RRB high variable was directly connected with Gross MQ low variable. The direct associations between motor variables and ADOS RRB variable in both the two areas indicated a non-casual association between ASD severity and motor function.
The clinical variables ADOS-SA (“high” and “low”) and Non-verbal IQ (“high” and “low”) were not directly connected with any motor variables. The “Age low” was directly connected to the variable “Motor Intervention.”
Benchmarking Analysis (Figures 4, 5)
The PCA provided a map in which the two clusters containing ADOS RRB “high” and “low” present a perfect symmetry. Analogously, the AHC provided a dendrogram in the two clusters containing ADOS RRB high and low show a perfect symmetry.
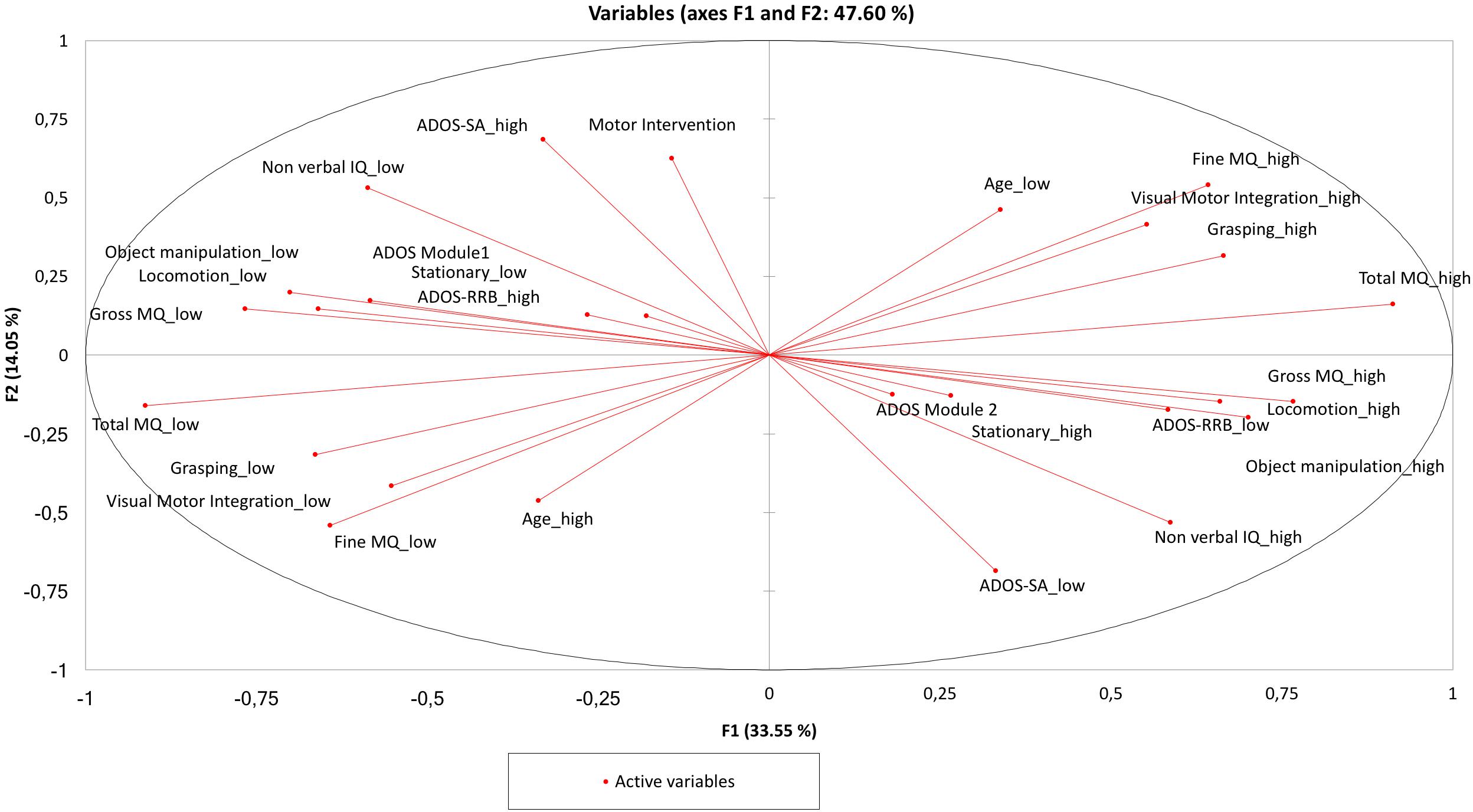
Figure 4. Principal component analysis (PCA). The two clusters containing ADOS RRB “high” and “low” are in a perfect symmetry. The cluster around ADOS RRB “high” indicates the following hierarchy: “ADOS Module 1,” “Stationary low,” “Object Manipulation low,” “Locomotion low,” “Gross MQ low,” and “Non-verbal IQ low,” and in the same way the cluster around ADOS RRB “low” shows the following hierarchy: “ADOS Module 2” “Stationary high,” “Object Manipulation high,” “Locomotion high,” “Gross MQ high,” and “Non-verbal IQ high.”
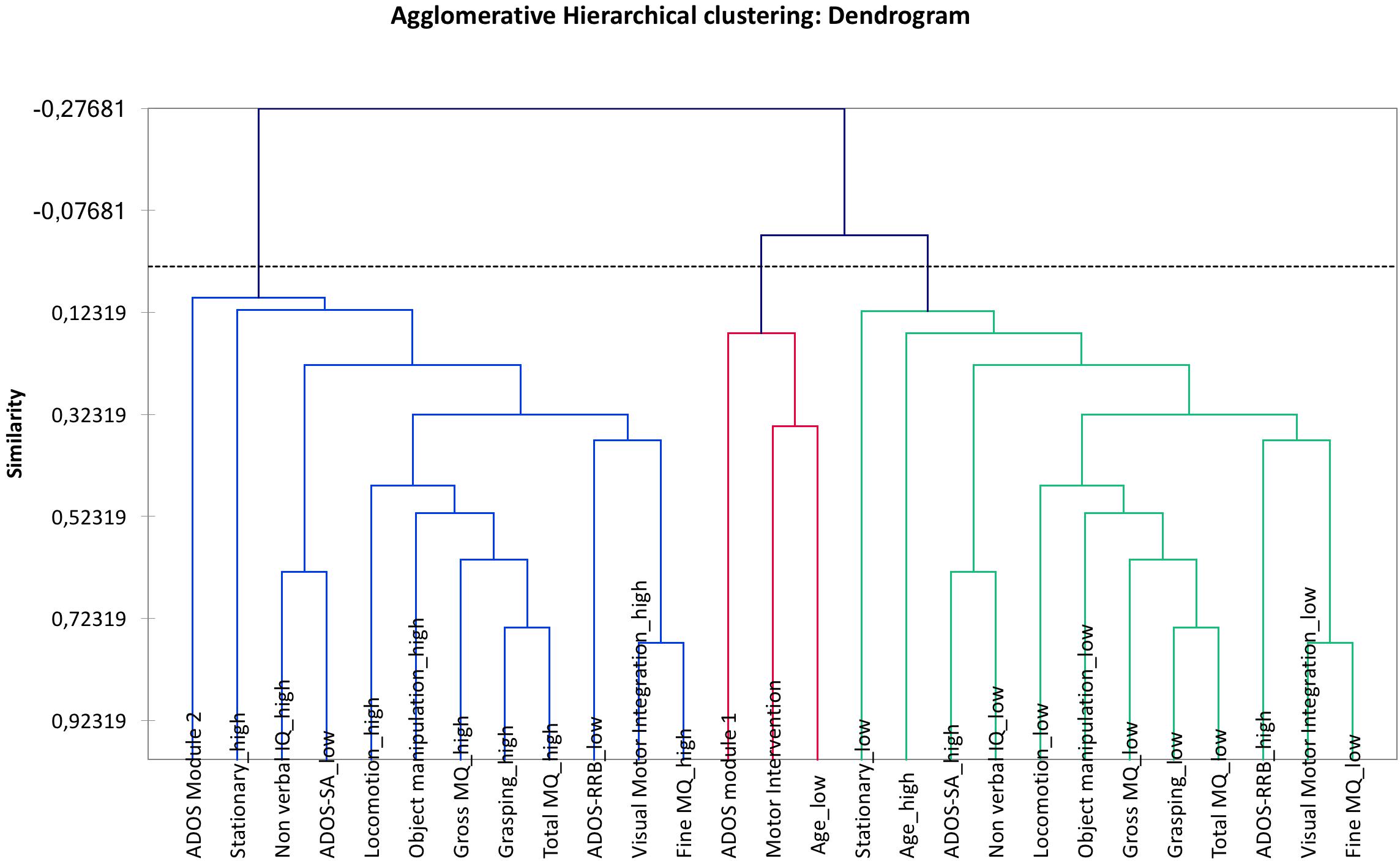
Figure 5. Agglomerative hierarchical clustering (AHC). The two clusters containing ADOS RRB “high” and “low” are in a perfect symmetry. The two clusters around ADOS RRB are: “visual-motor integration low” and “ADOS RRB high”; “visual-motor integration high” and “ADOS RRB low.”
Compared with PCA and HAC analysis, the Auto-CM analysis highlighted the asymmetry of the two clusters departing from ADOS RRB high and ADOS RRB low as one could expect from non-linear mathematics. The closest variable to ADOS RRB low is fine MQ high, while the closest variables to ADOS RRB high is gross MQ low. Asymmetry apart, Auto CM shows the relevance of motor component for other variables. This feature is missed with PCA and ACH.
Discussion
This study aimed to explore the associations between motor skills and clinical/developmental features in a sample of preschoolers with ASD through the application of ANNs analysis. We hypothesized that the ANNs could reveal simultaneous connections among the full spectrum of clinical/developmental variables.
First, the ANNs revealed that the Module 1 of the ADOS (designed for children whose spontaneous language is primarily in single words or preverbal) was the central node of the network: thus, the lower level of expressive language development was the most shared clinical feature among participants. This finding did not seem surprising since the Module 2 of the ADOS have been administrated to 25% of participants (see Table 2). However, the AANs revealed the direct connection between the lowest level of language skills of participants (i.e., Module 1) and the cluster of motor variables indicating the lowest motor functioning (see Figure 3). This finding acquired a greater relevance considering the growing literature suggesting a relationship between motor and language skills in children with ASD (Bhat et al., 2011; LeBarton and Iverson, 2013; Libertus and Violi, 2016). Motor impairments also emerged in children with some flexible phrase speech (i.e., Module 2 of the ADOS) suggesting that the relationship between motor and language skills may be not linear. It should be noted that our findings were limited by the lack of a direct and structured assessment of children’s language functioning. Thus, future studies aimed at investigating the interconnection between language and motor skills in individuals with ASD should provide a more detailed measure of language abilities, and they should also include children with several different degrees of language functioning.
Second, consistent with the results of the linear correlation analysis, the ANNs detected the association between the motor functioning and the repetitive behaviors in children with ASD. More specifically, the highest impaired motor skills were associated with the highest rate of RRB (see Figure 3). Our findings confirmed previous studies (Radonovich et al., 2013; Ravizza et al., 2013; Purpura et al., 2016; Uljarević et al., 2017) supporting the connection between motor and repetitive behaviors domains. Moreover, our results were consistent with some findings from neuroimaging studies according to which RRB and motor skills share common neural underpinnings as the basal ganglia, the cerebellum and the associated cortico-subcortical circuitries, including the striatum and thalamus (Lopez et al., 2005; Lam and Aman, 2007; Leekam et al., 2011).
Third, the AANs provided new insights into the simultaneous connections among motor skills and the full spectrum of variables including cognitive and social abilities. Consistent with previous data (Mari et al., 2003; Dewey et al., 2007; Green et al., 2009; Fulceri et al., 2015), linear correlation analysis revealed that the increase of motor skills, mainly fine motor skills, was associated with the increase of cognitive abilities. The ANNs approach did not disconfirm this finding but highlighted two different motor clusters differently connected with clinical and developmental variables (see Figure 3). Indeed, the motor variables indicating the lowest impaired motor skills were located close to the variables indicating a high level of cognitive skills and high social functioning. The variables indicating the highest impaired motor skills were located close (although to a lesser degree than the previous ones) to the variables indicating a low level of cognitive skills and low social functioning. According to this picture, children with the best motor functioning should be expected to present the lowest impairment in cognitive functioning and ASD severity. This latter picture seemed to emphasize the broad body of literature on this topic (Mari et al., 2003; Dewey et al., 2007; Green et al., 2009; Hilton et al., 2012; Fulceri et al., 2015) suggesting that motor skills may be accordingly influenced by different degree of impairment in clinical and developmental abilities.
There is a lack of strong closeness between the motor clusters and the variables indicating a different degree of social impairment. This finding led us to suppose that the relevance of motor development in the research field of ASD may be prevalent for RRB domain rather than in SA domain occurring regardless of the intellectual functioning. However, any interpretation of these preliminary data should be made with great caution given the methodology of the current work that may present limitations.
Fourth, the ANNs highlighted and expanded the relationship between motor skills and chronological age revealed from linear correlation analysis. Indeed, even if the ANNs approach did not detect a direct relationship between motor skills and chronological age, it should be noted that the cluster of highest impaired motor skills was located close to the variable “high chronological age,” supporting the hypothesis that motor abilities may worsen over time (Minshew et al., 2004; Lloyd et al., 2013). However, it is essential to consider that the cross-sectional nature of the current study implies caution in the interpretation of age-related phenomena, as they do not derive from intra-individual measures of change. Therefore, further longitudinal studies should be performed to strengthen these results.
Finally, the variable “motor intervention” was located at approximately the same distance between the two-motor clusters, and it was directly related to the lowest chronological age and the highest social affect impairment. This finding could be explained in the light of the Italian public care services context. Indeed, psychomotor intervention is among the early rehabilitative treatments offered to young children with a diagnosis of ASD, often regardless of their motor functioning. The lack of proximity between the variable “motor intervention” and “highest chronological age” (i.e., older children) was quite unexpected. Indeed, since this treatment is offered at an early age of development, the older children should also have practiced it. Our methodology limited further interpretations. Indeed, the question relating to psychomotor treatment was extremely vague and the answers of parents, including possible memory bias, were not investigated in more detail.
Several limitations should be discussed. The most relevant is undoubtedly the constrained data-set that strongly limits wide-ranging conclusions. The sample of participants was limited to a group of 32 male children with non-verbal IQ ≥ 70. It should be noted that in this study, only non-verbal IQ scores ≥ 70 were considered and that several standardized tests were used to assess intellectual abilities according to clinical features. Even if a numbers of exclusion criteria have been defined, it should be noted that it could be problematic to artificially define cutoff point of 70 of non-verbal IQ for ruling out co-morbidity of intellectual disability based on the age range (30–60 months) and the issues of testing non-verbal/verbal IQs for children (Bishop et al., 2015). However, the adaptive learning algorithms of inference, based on the principle of a functional estimation like ANN, has been reported as a strategical approach to overcome the problem of dimensionality (Buscema et al., 2015). Different from the classical statistics, the ANNs can manage complexity even with relatively small samples and to the subsequent unbalanced ratio between variables and records. Among other limitations, it should be noted that the PDMS-2 motor scores could reflect not only the motor impairment but also the child’s difficulties to attend the standardized context. However, the PDMS-2 has been widely and successfully used in children with ASD (Provost et al., 2007; Vanvuchelen et al., 2007; Jasmin et al., 2009; Zachor et al., 2010), due to the lack of time trials and the less cognitive demand compared to other motor assessment tools (Vanvuchelen et al., 2007).
Another substantial limitation of our findings relates to the lack of a typically developing control group. Indeed, even if the PDMS-2 is a standardized clinical tool providing a judgment of motor skills compared to a control sample, the lack of a typically developing group is a methodological criticism that should be underscored. Moreover, also the lack of a control group consisting of preschoolers with other neurodevelopmental disorders (e.g., intellectual disability, attention deficit hyperactivity disorder) should be mentioned since it prevents us to consider the motor abnormalities we detected as specific of ASD. Thus, further research needs to be performed to confirm our findings and to determine the value of motor impairments in ASD compared to those of children with other neurodevelopmental disorders. Also, this study is limited to male children with ASD. In the literature, the issue of gender differences in ASD has been widely discussed. However, even if it has been hypothesized an impaired motor functioning in females with ASD (Kopp et al., 2010), the results of this study are not able to contribute to this discussion.
Conclusion
In conclusion, this study revealed the pervasiveness of motor impairments in ASD and implemented a machine learning approach to highlight how it is related to repetitive behaviors and low level of expressive language as well as cognitive and social skills. Considering the above-mentioned limitations, this study provided a detailed delineation of the Auto-CM model for unsupervised ANN and its implementation for data mining to discover the “hidden” relationships among multidimensional variables with clusters on a map. This represents a novel approach to understanding the complexity of a key “impairment” measure in ASD such as motor skills and how it is related to other critical measures that define ASD. Findings suggests that motor skills may be a moderator of clinical or developmental features in young children with ASD and that the systematic observation of motor development in ASD may improve the knowledge about clinical and neurobiological involvement as well as guide development of tailored treatments.
Author Contributions
FF, EG, AC, SC, and FM contributed to the study conception and design, analysis and interpretation of data, to the writing of the manuscript, and to its critical revision. FF, AN, FA, RT, and IP contributed to the acquisition of data, and to the writing of the manuscript and its critical revision. All authors have approved the final version of the manuscript and are accountable for the work described.
Funding
This work has been partially supported by the European Community’s Horizon 2020 Program under the grant agreement n. 642996 (BRAINVIEW), by the Italian Ministry of Health Network Project ‘Italian Autism Spectrum Disorders Network: filling the gaps in the National Health System Care’ (NET 2013-02355263), and by grant from the IRCCS Fondazione Stella Maris (Ricerca Corrente, and the “5 × 1000” voluntary contributions, Italian Ministry of Health).
Conflict of Interest Statement
The authors declare that the research was conducted in the absence of any commercial or financial relationships that could be construed as a potential conflict of interest.
Supplementary Material
The Supplementary Material for this article can be found online at: https://www.frontiersin.org/articles/10.3389/fpsyg.2018.02683/full#supplementary-material
References
American Psychiatric Association [APA] (2000). Diagnostic and Statistical Manual of Mental Disorders, 4th Edn. Washington, DC: American Psychiatric Association.
American Psychiatric Association [APA] (2013). Diagnostic and Statistical Manual of Mental Disorders, 5th Edn. Washington, DC: American Psychiatric Association. doi: 10.1176/appi.books.9780890425596
Ayer, T., Chen, Q., and Burnside, E. S. (2013). Artificial neural networks in mammography interpretation and diagnostic decision making. Comput. Math. Methods Med. 2013:832509. doi: 10.1155/2013/832509
Bedford, R., Pickles, A., and Lord, C. (2016). Early gross motor skills predict the subsequent development of language in children with autism spectrum disorder. Autism Res. 9, 993–1001. doi: 10.1002/aur.1587
Benjamini, Y., and Yekutieli, D. (2001). The control of the false discovery rate in multiple testing under dependency. Ann. Statist. 29, 1165—-1188. doi: 10.1214/aos/1013699998
Bhat, A. N., Landa, R. J., and Galloway, J. C. (2011). Current perspectives on motor functioning in infants, children, and adults with autism spectrum disorders. Phys. Ther. 91, 1116–1129. doi: 10.2522/ptj.20100294
Biancotto, M., Girelli, L., Maggiore, P., Pelamatti, G. M., Rossi, G., Simonelli, A., et al. (2017). PDMS-2 – Peabody Developmental Motor Scales, 2ndEdn. Austin, TX: Hoghrefe.
Bishop, J. C., and Pangelinan, M. (2018). Motor skills intervention research of children with disabilities. Res. Dev. Disabil. 74, 14–30. doi: 10.1016/j.ridd.2017.11.002
Bishop, S. L., Farmer, C., and Thurm, A. (2015). Measurement of nonverbal IQ in autism spectrum disorder: scores in young adulthood compared to early childhood. J. Autism Dev. Disord. 45, 966–974. doi: 10.1007/s10803-014-2250-3
Brand, S., Jossen, S., Holsboer-Trachsler, E., Pühse, U., and Gerber, M. (2015). Impact of aerobic exercise on sleep and motor skills in children with autism spectrum disorders - a pilot study. Neuropsychiatr. Dis. Treat. 11, 1911–1920. doi: 10.2147/NDT.S85650
Bremer, E., Balogh, R., and Lloyd, M. (2015). Effectiveness of a fundamental motor skill intervention for 4-year-old children with autism spectrum disorder: a pilot study. Autism 19, 980–991. doi: 10.1177/1362361314557548
Buscema, M., and Grossi, E. (2008). The semantic connectivity map: an adapting self-organising knowledge discovery method in data bases experience in gastro-oesophageal reflux disease. Int. J. Data Min. Bioinform. 2, 362–404. doi: 10.1504/IJDMB.2008.022159
Buscema, M., Grossi, E., Montanini, L., and Street, M. E. (2015). Data mining of determinants of intrauterine growth retardation revisited using novel algorithms generating semantic maps and prototypical discriminating variable profiles. PLoS One 10:e0126020. doi: 10.1371/journal.pone.0126020
Buscema, M., Grossi, E., Snowdon, D., and Antuono, P. (2008). Auto-Contractive Maps: an artificial adaptive system for data mining. An application to Alzheimer disease. Curr. Alzheimer Res. 5, 481–498. doi: 10.2174/156720508785908928
Buscema, M., and Sacco, P. L. (2017). Digging deeper on “deep” learning: a computational ecology approach. Behav. Brain Sci. 40:e256. doi: 10.1017/S0140525X1700005X
Campione, G. C., Piazza, C., Villa, L., and Molteni, M. (2016). Three-Dimensional kinematic analysis of prehension movements in young children with autism spectrum disorder: new insights on motor impairment. J. Autism Dev. Disord. 46, 1985–1999. doi: 10.1007/s10803-016-2732-6
Cocchi, M., Tonello, L., Tsaluchidu, S., and Puri, B. K. (2008). The use of artificial neural networks to study fatty acids in neuropsychiatric disorders. BMC Psychiatry 8(Suppl. 1):S3. doi: 10.1186/1471-244X-8-S1-S3
Dewey, D., Cantell, M., and Crawford, S. G. (2007). Motor and gestural performance in children with autism spectrum disorders, developmental coordination disorder, and/or attention deficit hyperactivity disorder. J. Int. Neuropsychol. Soc. 13, 246–256. doi: 10.1017/S1355617707070270
Djemal, R., AlSharabi, K., Ibrahim, S., and Alsuwailem, A. (2017). EEG-based computer aided diagnosis of autism spectrum disorder using wavelet, entropy, and ANN. Biomed. Res. Int. 2017:9816591. doi: 10.1155/2017/9816591
Dowell, L. R., Mahone, E. M., and Mostofsky, S. H. (2009). Associations of postural knowledge and basic motor skill with dyspraxia in autism: implication for abnormalities in distributed connectivity and motor learning. Neuropsychology 23, 563–570. doi: 10.1037/a0015640
Dziuk, M. A., Gidley Larson, J. C., Apostu, A., Mahone, E. M., Denckla, M. B., and Mostofsky, S. H. (2007). Dyspraxia in autism: association with motor, social, and communicative deficits. Dev. Med. Child Neurol. 49, 734–739. doi: 10.1111/j.1469-8749.2007.00734.x
Eggleston, J. D., Harry, J. R., Hickman, R. A., and Dufek, J. S. (2017). Analysis of gait symmetry during over-ground walking in children with autism spectrum disorder. Gait Posture 55, 162–166. doi: 10.1016/j.gaitpost.2017.04.026
Esposito, G., and Venuti, P. (2008). Analysis of toddlers’ gait after six months of independent walking to identify autism: a preliminary study. Percept. Mot. Skills 106, 259–269. doi: 10.2466/pms.106.1.259-269
Esposito, G., Venuti, P., Apicella, F., and Muratori, F. (2011). Analysis of unsupported gait in toddlers with autism. Brain Dev. 33, 367–373. doi: 10.1016/j.braindev.2010.07.006
Esposito, G., Venuti, P., Maestro, S., and Muratori, F. (2009). An exploration of symmetry in early autism spectrum disorders: analysis of lying. Brain Dev. 31, 131–138. doi: 10.1016/j.braindev.2008.04.005
Folio, M. R., and Fewell, R. R. (2000). Peabody Developmental Motor Scales Examiner’s Manual, 2nd Edn. Austin,TX: Pro-Ed, Inc.
Fournier, K. A., Hass, C. J., Naik, S. K., Lodha, N., and Cauraugh, J. H. (2010). Motor coordination in autism spectrum disorders: a synthesis and meta-analysis. J. Autism Dev. Disord. 40, 1227–1240. doi: 10.1007/s10803-010-0981-3
Fredman, M. L., and Willard, D. E. (1990). “Trans-dichotomous algorithms for minimum spanning trees and shortest paths,” in Proceedings of the 1990 31st Annual Symposium on Foundations of Computer Science, Vol. 2, (St. Louis, MO: IEEE), 719–725. doi: 10.1109/FSCS.1990.89594
Fulceri, F., Contaldo, A., Parrini, I., Calderoni, S., Narzisi, A., Tancredi, R., et al. (2015). Locomotion and grasping impairment in preschoolers with autism spectrum disorder. Clin. Neuropsychiatry 12, 94–100.
Gallese, V., Rochat, M. J., and Berchio, C. (2013). The mirror mechanism and its potential role in autism spectrum disorder. Dev. Med. Child Neurol. 55, 15–22. doi: 10.1111/j.1469-8749.2012.04398.x
Garrido, D., Petrova, D., Watson, L. R., Garcia-Retamero, R., and Carballo, G. (2017). Language and motor skills in siblings of children with autism spectrum disorder: a meta-analytic review. Autism Res. 10, 1737–1750. doi: 10.1002/aur.1829
Gernsbacher, M. A., Sauer, E. A., Geye, H. M., Schweigert, E. K., and Hill Goldsmith, H. (2008). Infant and toddler oral- and manual-motor skills predict later speech fluency in autism. J. Child Psychol. Psychiatry 49, 43–50. doi: 10.1111/j.1469-7610.2007.01820.x
Gima, H., Kihara, H., Watanabe, H., Nakano, H., Nakano, J., Konishi, Y., et al. (2018). Early motor signs of autism spectrum disorder in spontaneous position and movement of the head. Exp. Brain Res. 236, 1139–1148. doi: 10.1007/s00221-018-5202-x
Gironi, M., Saresella, M., Rovaris, M., Vaghi, M., Nemni, R., Clerici, M., et al. (2013). A novel data mining system points out hidden relationships between immunological markers in multiple sclerosis. Immun. Ageing 10:1. doi: 10.1186/1742-4933-10-1
Glazebrook, C. M., Elliott, D., and Lyons, J. (2006). A kinematic analysis of how young adults with and without autism plan and control goal-directed movements. Motor Control 10, 244–264. doi: 10.1123/mcj.10.3.244
Gotham, K., Pickles, A., and Lord, C. (2009). Standardizing ADOS scores for a measure of severity in autism spectrum disorders. J. Autism Dev. Disord. 39, 693–705. doi: 10.1007/s10803-008-0674-3
Green, D., Charman, T., Pickles, A., Chandler, S., Loucas, T., Simonoff, E., et al. (2009). Impairment in movement skills of children with autistic spectrum disorders. Dev. Med. Child Neurol. 51, 311–316. doi: 10.1111/j.1469-8749.2008.03242.x
Grossi, E., Migliore, L., and Muratori, F. (2018). Pregnancy risk factors related to autism: an Italian case-control study in mothers of children with autism spectrum disorders (ASD), their siblings and of typically developing children. J. Dev. Orig. Health Dis. 9, 442–449. doi: 10.1017/S2040174418000211
Grossi, E., Olivieri, C., and Buscema, M. (2017). Diagnosis of autism through EEG processed by advanced computational algorithms: a pilot study. Comput. Methods Programs 142, 73–79. doi: 10.1016/j.cmpb.2017.02.002
Hedgecock, J. B., Dannemiller, L. A., Shui, A. M., Rapport, M. J., and Katz, T. (2018). Associations of gross motor delay, behavior, and quality of life in young children with autism spectrum disorder. Phys. Ther. 98, 251–259. doi: 10.1093/ptj/pzy006
Hilton, C. L., Zhang, Y., Whilte, M. R., Klohr, C. L., and Constantino, J. (2012). Motor impairment in sibling pairs concordant and discordant for autism spectrum disorders. Autism 16, 430–441. doi: 10.1177/1362361311423018
Hus Bal, V., and Lord, C. (2015). Replication of standardized ados domain scores in the simons simplex collection. Autism Res. 8, 583–592. doi: 10.1002/aur.1474
Iverson, J. M. (2010). Multimodality in infancy: vocal-motor and speech-gesture coordinations in typical and atypical development. Enfance 2010, 257–274. doi: 10.4074/S0013754510003046
Jasmin, E., Couture, M., McKinley, P., Reid, G., Fombonne, E., and Gisel, E. (2009). Sensori-motor and daily living skills of preschool children with autism spectrum disorders. J. Autism Dev. Disord. 39, 231–241. doi: 10.1007/s10803-008-0617-z
Ketcheson, L., Hauck, J., and Ulrich, D. (2017). The effects of an early motor skill intervention on motor skills, levels of physical activity, and socialization in young children with autism spectrum disorder: a pilot study. Autism 21, 481–492. doi: 10.1177/1362361316650611
Kim, H. U. (2008). Development of early language and motor skills in preschool children with autism. Percept. Mot. Skills 107, 403–406. doi: 10.2466/pms.107.2.403-406
Kopp, S., Beckung, E., and Gillberg, C. (2010). Developmental coordination disorder and other motor control problems in girls with autism spectrum disorder and/or attention-deficit/hyperactivity disorder. Res. Dev. Disabil. 31, 350–361. doi: 10.1016/j.ridd.2009.09.017
Krogh, A. (2008). What are artificial neural networks? Nat. Biotechnol. 26, 195–197. doi: 10.1038/nbt1386
Kruskal, J. B. (1956). On the shortest spanning subtree of a graph and the traveling salesman problem. Proc. Am. Math. Soc. 7, 48–50. doi: 10.1090/S0002-9939-1956-0078686-7
Lam, K. S. L., and Aman, M. G. (2007). The repetitive behavior scale-revised: independent validation in individuals with autism spectrum disorders. J. Autism Dev. Disord. 37, 855–866. doi: 10.1007/s10803-006-0213-z
Landa, R., and Garrett-Mayer, E. (2006). Development in infants with autism spectrum disorders: a prospective study. J. Child Psychol. Psychiatry 47, 629–638. doi: 10.1111/j.1469-7610.2006.01531.x
Landa, R. J., Gross, A. L., Stuart, E. A., and Faherty, A. (2013). Developmental trajectories in children with and without autism spectrum disorders: the first 3 years. Child Dev. 84, 429–442. doi: 10.1111/j.1467-8624.2012.01870.x
LeBarton, E. S., and Iverson, J. M. (2013). Fine motor skill predicts expressive language in infant siblings of children with autism. Dev. Sci. 16, 815–827. doi: 10.1111/desc.12069
Leekam, S. R., Prior, M. R., and Uljarevic, M. (2011). Restricted and repetitive behaviors in autism spectrum disorders: a review of research in the last decade. Psychol. Bull. 137, 562–593. doi: 10.1037/a0023341
Libertus, K., Sheperd, K. A., Ross, S. W., and Landa, R. J. (2014). Limited fine motor and grasping skills in 6-month-old infants at high risk for autism. Child Dev. 85, 2218–2231. doi: 10.1111/cdev.12262
Libertus, K., and Violi, D. A. (2016). Sit to talk: relation between motor skills and language development in infancy. Front. Psychol. 7:475. doi: 10.3389/fpsyg.2016.00475
Lloyd, M., MacDonald, M., and Lord, C. (2013). Motor skills of toddlers with autism spectrum disorders. Autism Int. J. Res. Pract. 17, 133–146. doi: 10.1177/1362361311402230
Longuet, S., Ferrel-Chapus, C., Orêve, M.-J., Chamot, J.-M., and Vernazza-Martin, S. (2012). Emotion, intent and voluntary movement in children with autism. An example: the goal directed locomotion. J. Autism Dev. Disord. 42, 1446–1458. doi: 10.1007/s10803-011-1383-x
Lopez, B. R., Lincoln, A. J., Ozonoff, S., and Lai, Z. (2005). Examining the relationship between executive functions and restricted, repetitive symptoms of Autistic Disorder. J. Autism Dev. Disord. 35, 445–460. doi: 10.1007/s10803-005-5035-x
Lord, C., Risi, S., Lambrecht, L., Cook, E. H., Leventhal, B. L., DiLavore, P. C., et al. (2000). The autism diagnostic observation schedule-generic: a standard measure of social and communication deficits associated with the spectrum of autism. J. Autism Dev. Disord. 30, 205–223. doi: 10.1023/A:1005592401947
Luiz, D. M., Barnard, A., Knoesen, N., Kotras, N., Horrocks, S., McAlinden, P., et al. (2006). Griffiths Mental Developmental Scales-Extended Revised: Two to Eight Years. Analysis Manual. Oxford, MS: Hogrefe.
MacDonald, M., Lord, C., and Ulrich, D. A. (2013). The relationship of motor skills and social communicative skills in school-aged children with autism spectrum disorder. Adapt. Phys. Activ. Q. 30, 271–282. doi: 10.1123/apaq.30.3.271
Manning, T., Sleator, R. D., and Walsh, P. (2014). Biologically inspired intelligent decision making: a commentary on the use of artificial neural networks in bioinformatics. Bioengineered 5, 80–95. doi: 10.4161/bioe.26997
Mari, M., Castiello, U., Marks, D., Marraffa, C., and Prior, M. (2003). The reach-to-grasp movement in children with autism spectrum disorder. Philos. Trans. R. Soc. Lond. B Biol. Sci. 358, 393–403. doi: 10.1098/rstb.2002.1205
May, T., McGinley, J., Murphy, A., Hinkley, T., Papadopoulos, N., Williams, K. J., et al. (2016). A multidisciplinary perspective on motor impairment as an early behavioural marker in children with autism spectrum disorder. Aust. Psychol. 51, 296–303. doi: 10.1111/ap.12225
Miller, M., Chukoskie, L., Zinni, M., Townsend, J., and Trauner, D. (2014). Dyspraxia, motor function and visual-motor integration in autism. Behav. Brain Res. 269, 95–102. doi: 10.1016/j.bbr.2014.04.011
Minshew, N. J., Sung, K., Jones, B. L., and Furman, J. M. (2004). Underdevelopment of the postural control system in autism. Neurology 63, 2056–2061. doi: 10.1212/01.WNL.0000145771.98657.62
Moseley, R. L., and Pulvermüller, F. (2018). What can autism teach us about the role of sensorimotor systems in higher cognition? New clues from studies on language, action semantics, and abstract emotional concept processing. Cortex 100, 149–190. doi: 10.1016/j.cortex.2017.11.019
Najafabadi, M. G., Sheikh, M., Hemayattalab, R., Amir, M., Rezaii, M., and Hafizi, S. (2018). The effect of spark on social and motor skills of children with autism. Pediatr. Neonatol. 59, 481–487. doi: 10.1016/j.pedneo.2017.12.005
Narzisi, A., Muratori, F., Buscema, M., Calderoni, S., and Grossi, E. (2015). Outcome predictors in autism spectrum disorders preschoolers undergoing treatment as usual: insights from an observational study using artificial neural networks. Neuropsychiatr. Dis. Treat. 11, 1587–1599. doi: 10.2147/NDT.S81233
Ozonoff, S., Young, G. S., Goldring, S., Greiss-Hess, L., Herrera, A. M., Steele, J., et al. (2008). Gross motor development, movement abnormalities, and early identification of autism. J. Autism Dev. Disord. 38, 644–656. doi: 10.1007/s10803-007-0430-0
Pan, C.-Y., Chu, C.-H., Tsai, C.-L., Sung, M.-C., Huang, C.-Y., and Ma, W.-Y. (2017). The impacts of physical activity intervention on physical and cognitive outcomes in children with autism spectrum disorder. Autism 21, 190–202. doi: 10.1177/1362361316633562
Peixoto, L. A., Bhering, L. L., and Cruz, C. D. (2015). Artificial neural networks reveal efficiency in genetic value prediction. Genet. Mol. Res. 14, 6796–6807. doi: 10.4238/2015.June.18.22
Phagava, H., Muratori, F., Einspieler, C., Maestro, S., Apicella, F., Guzzetta, A., et al. (2008). General movements in infants with autism spectrum disorders. Georgian Med. News 156, 100–105.
Provost, B., Lopez, B. R., and Heimerl, S. (2007). A comparison of motor delays in young children: autism spectrum disorder, developmental delay, and developmental concerns. J. Autism Dev. Disord. 37, 321–328. doi: 10.1007/s10803-006-0170-6
Purpura, G., Fulceri, F., Puglisi, V., Masoni, P., and Contaldo, A. (2016). Motor coordination impairment in children with autism spectrum disorder: a pilot study using movement assessment battery for children-2 checklist. Minerva Pediatr. 12:2016.
Radonovich, K. J., Fournier, K. A., and Hass, C. J. (2013). Relationship between postural control and restricted, repetitive behaviors in autism spectrum disorders. Front. Integr. Neurosci. 7:28. doi: 10.3389/fnint.2013.00028
Ravizza, S. M., Solomon, M., Ivry, R. B., and Carter, C. S. (2013). Restricted and repetitive behaviors in autism spectrum disorders: the relationship of attention and motor deficits. Dev. Psychopathol. 25, 773–784. doi: 10.1017/S0954579413000163
Roid, G. H., and Miller, L. J. (1997). Leiter International Performance Scale-Revised. Lutz, FL: Psicological Assessment Resources.
Sacrey, L.-A. R., Germani, T., Bryson, S. E., and Zwaigenbaum, L. (2014). Reaching and grasping in autism spectrum disorder: a review of recent literature. Front. Neurol. 5:6. doi: 10.3389/fneur.2014.00006
Soska, K. C., Adolph, K. E., and Johnson, S. P. (2010). Systems in development: motor skill acquisition facilitates 3d object completion. Dev. Psychol. 46, 129–138. doi: 10.1037/a0014618
Srinivasan, S. M., and Bhat, A. N. (2016). Differences in object sharing between infants at risk for autism and typically developing infants from 9 to 15 months of age. Infant Behav. Dev. 42, 128–141. doi: 10.1016/j.infbeh.2015.12.003
Staples, K. L., and Reid, G. (2010). Fundamental movement skills and autism spectrum disorders. J. Autism Dev. Disord. 40, 209–217. doi: 10.1007/s10803-009-0854-9
Stins, J. F., Emck, C., de Vries, E. M., Doop, S., and Beek, P. J. (2015). Attentional and sensory contributions to postural sway in children with autism spectrum disorder. Gait Posture 42, 199–203. doi: 10.1016/j.gaitpost.2015.05.010
Street, M. E., Grossi, E., Volta, C., Faleschini, E., and Bernasconi, S. (2008). Placental determinants of fetal growth: identification of key factors in the insulin-like growth factor and cytokine systems using artificial neural networks. BMC Pediatr. 8:24. doi: 10.1186/1471-2431-8-24
Toscano, M., De Grandi, R., Peroni, D. G., Grossi, E., Facchin, V., Comberiati, P., et al. (2017). Impact of delivery mode on the colostrum microbiota composition. BMC Microbiol. 17:205. doi: 10.1186/s12866-017-1109-0
Travers, B. G., Powell, P. S., Klinger, L. G., and Klinger, M. R. (2013). Motor difficulties in autism spectrum disorder: linking symptom severity and postural stability. J. Autism Dev. Disord. 43, 1568–1583. doi: 10.1007/s10803-012-1702-x
Uljarević, M., Hedley, D., Alvares, G. A., Varcin, K. J., and Whitehouse, A. J. O. (2017). Relationship between early motor milestones and severity of restricted and repetitive behaviors in children and adolescents with autism spectrum disorder. Autism Res. 10, 1163–1168. doi: 10.1002/aur.1763
Vanvuchelen, M., Roeyers, H., and De Weerdt, W. (2007). Nature of motor imitation problems in school-aged boys with autism: a motor or a cognitive problem? Autism 11, 225–240.
Wechsler, D. (1974). Manual for the Wechsler Scale for Children – Revised (WISC-R). New York, NY: The psychological corporation.
Whyatt, C. P., and Craig, C. M. (2012). Motor skills in children aged 7-10 years, diagnosed with autism spectrum disorder. J. Autism Dev. Disord. 42, 1799–1809. doi: 10.1007/s10803-011-1421-8
Wilson, R. B., Enticott, P. G., and Rinehart, N. J. (2018). Motor development and delay: advances in assessment of motor skills in autism spectrum disorders. Curr. Opin. Neurol. 31, 134–139. doi: 10.1097/WCO.0000000000000541
Zachor, D., Ilanit, T., and Ben-Itzchak, E. (2010). Autism severity and motor abilities correlates of imitation situations in children with autism spectrum disorders. Res. Autism Spectr. Disord. 4, 438–443. doi: 10.1016/j.rasd.2009.10.016
Zappella, M., Einspieler, C., Bartl-Pokorny, K. D., Krieber, M., Coleman, M., Bölte, S., et al. (2015). What do home videos tell us about early motor and socio-communicative behaviours in children with autistic features during the second year of life–An exploratory study. Early Hum. Dev. 91, 569–575. doi: 10.1016/j.earlhumdev.2015.07.006
Keywords: autism spectrum disorders, motor impairments, motor skills, repetitive behaviors, artificial neural network, Peabody Developmental Motor Scale, preschoolers
Citation: Fulceri F, Grossi E, Contaldo A, Narzisi A, Apicella F, Parrini I, Tancredi R, Calderoni S and Muratori F (2019) Motor Skills as Moderators of Core Symptoms in Autism Spectrum Disorders: Preliminary Data From an Exploratory Analysis With Artificial Neural Networks. Front. Psychol. 9:2683. doi: 10.3389/fpsyg.2018.02683
Received: 21 May 2018; Accepted: 13 December 2018;
Published: 09 January 2019.
Edited by:
Pamela Bryden, Wilfrid Laurier University, CanadaReviewed by:
Chris Lange-Küttner, London Metropolitan University, United KingdomYang Zhang, University of Minnesota Twin Cities, United States
Copyright © 2019 Fulceri, Grossi, Contaldo, Narzisi, Apicella, Parrini, Tancredi, Calderoni and Muratori. This is an open-access article distributed under the terms of the Creative Commons Attribution License (CC BY). The use, distribution or reproduction in other forums is permitted, provided the original author(s) and the copyright owner(s) are credited and that the original publication in this journal is cited, in accordance with accepted academic practice. No use, distribution or reproduction is permitted which does not comply with these terms.
*Correspondence: Sara Calderoni, sara.calderoni@fsm.unipi.it; sara.calderoni@inpe.unipi.it