Training the developing brain: a neurocognitive perspective
- 1Leiden Institute for Brain and Cognition (LIBC), Leiden University, Leiden, Netherlands
- 2Department of Radiology, Leiden University Medical Center, Leiden, Netherlands
- 3Institute of Psychology, Leiden University, Leiden, Netherlands
Developmental training studies are important to increase our understanding of the potential of the developing brain by providing answers to questions such as: “Which functions can and which functions cannot be improved as a result of practice?,” “Is there a specific period during which training has more impact?,” and “Is it always advantageous to train a particular function?”In addition, neuroimaging methods provide valuable information about the underlying mechanisms that drive cognitive plasticity. In this review, we describe how neuroscientific studies of training effects inform us about the possibilities of the developing brain, pointing out that childhood is a special period during which training may have different effects. We conclude that there is much complexity in interpreting training effects in children. Depending on the type of training and the level of maturation of the individual, training may influence developmental trajectories in different ways. We propose that the immature brain structure might set limits on how much can be achieved with training, but that the immaturity can also have advantages, in terms of flexibility for learning.
Introduction
The human brain is highly plastic and adapts quickly to new experiences. Several examples are at hand that highlight the plasticity of the brain in adults. For instance, a famous set of studies with London taxi drivers suggested that the gray matter volume in the hippocampus, a region important for memory, can be modulated by training. Moreover, these studies showed that hippocampal gray matter volume corresponded with the level of driving experience (Maguire et al., 2000, 2006) (see e.g., Elbert et al., 1995; Gaser and Schlaug, 2003 for similar results in musicians). Besides brain structure, also the function of the brain can be influenced by training. There is evidence from studies showing altered brain activation in limbic and/or frontoparietal regions for long-term meditation practitioners (Brefczynski-Lewis et al., 2007; Lutz et al., 2008) and after training with working memory tasks (Olesen et al., 2004; Jolles et al., 2010; Klingberg, 2010). It is well known that much of our learning takes place in childhood. But what do we know about the plasticity and flexibility of the developing brain? How can neuroscientific studies increase our insight of training effects during development?
In this article, we suggest that childhood might be special period during which training has specific effects. Currently, relatively little is known about how training-related plasticity differs between children and adults, but this direction of research has great potential for tailoring optimal learning situations. On the one hand, there are great changes in neural efficiency during development, which could make this period well suited for training interventions. On the other hand, there might also be limitations on the effects of training in childhood. That is, the maximum achievable performance could be constrained by the current level of structural brain development and cognitive functioning. Neuroimaging studies can provide a deeper level of insight in the underlying cognitive and neural processes that are involved during training (cf. Lustig et al., 2009). In this review, we mainly focus on (neuroscientific) training studies in the domain of cognitive control and working memory. In adults, these functions are associated with activation in a common set of regions in prefrontal and parietal cortex (Duncan and Owen, 2000; Wager and Smith, 2003; Owen et al., 2005). Several behavioral studies have demonstrated improved performance after cognitive training in children, and there is now a growing interest in the changes in frontoparietal brain regions that accompany these behavioral changes.
In the following sections, we first give a general introduction about the aims and methods of cognitive training studies, based on the child and adult behavioral literature. Then, we provide background on the interplay between brain maturation and training effects. Finally, we discuss the results of the first neuroscientific training studies in children. We conclude with some critical considerations and directions for future research.
Cognitive Training: Purpose and Approach
Training Paradigms
In this article, cognitive training is defined as the process of improving cognitive functioning by means of practice and/or intentional instruction. For alternative approaches to improve cognitive functions, including ecological interventions, physical exercise, and social interaction, we refer to previous reviews of cognitive interventions in children (Diamond and Lee, 2011; Bryck and Fisher, 2012) and adults (Hertzog et al., 2009; Lustig et al., 2009; Noack et al., 2009; Buschkuehl and Jaeggi, 2010). In general, cognitive training studies have focused on two goals: application (i.e., designing a training intervention that is effective in practice), and theory (i.e., answering empirical questions about the functions that are being trained and the processes responsible for the desired change) (Willis and Schaie, 2009). While determining the efficacy of a training program is a key objective in most training studies, it is equally important that training studies provide new insights into the processes of cognitive plasticity and the underlying neural mechanisms. For example, theory-based training studies may help to determine which aspects of the training program are driving training effects, and why some individuals gain more from training than others. In addition, theory-based training studies can improve our understanding of the specific functions that are being trained and why these functions are sometimes compromised (Willis and Schaie, 2009).
Depending on the goals of the study, a variety of different training paradigms can be used. The major approaches of cognitive training can roughly be classified as process-based and strategy-based training paradigms (cf. Lustig et al., 2009; Noack et al., 2009; Morrison and Chein, 2010). The process-based approach involves repeated performance (i.e., practice) of demanding executive function tasks. Most process-based studies in children have focused on training of working memory (e.g., Klingberg et al., 2005; Holmes et al., 2009a; Van der Molen et al., 2010; Jaeggi et al., 2011; Jolles et al., 2012), but other functions have been studied as well, including (executive) attention (e.g., Rueda et al., 2005; Shalev et al., 2007), inhibition (e.g., Thorell et al., 2009; Johnstone et al., 2010), and task switching (e.g., Karbach and Kray, 2009). The strategy-based approach on the other hand uses more explicit task instructions. For instance, in the domain of working memory, strategy training studies have promoted the use of rehearsal, chunking, mental imagery, and/or story-formation strategies to increase the number of items that are held in mind (e.g., Ford et al., 1984; Conners et al., 2008; St. Clair-Thompson et al., 2010; Swanson et al., 2010). Other strategy-based studies have used a more general approach, providing metacognitive knowledge about controlling and regulating task procedures and strategies (e.g., Ghatala et al., 1985; Kramarski and Mevarech, 2003). While it has been argued that process-based training of core executive functions will show a broader generalization because it is more domain-general in nature (cf. Lustig et al., 2009; Noack et al., 2009; Klingberg, 2010; Morrison and Chein, 2010), the strategy-based approach might be specifically effective in studies that aim to improve a particular skill (e.g., in arithmetic or language). Interestingly, in a study of children with attention difficulties, both typical process-based attention training and training of academic skills (which involved strategy-based elements) reduced attention problems. However, only the children who took part in the academic training improved significantly on (some) academic skills (Rabiner et al., 2010). Finally, a number of studies have explored the combination of process-based training and strategy instructions (van't Hooft et al., 2003, 2005; Chenault et al., 2006). One of these studies demonstrated that children with dyslexia benefit more from writing instruction when this is preceded by process-based training of attention, than when it is preceded by a control training (reading fluency). Notably, the attention training itself did not directly improve writing skills; it was the combination of training programs that yielded the best results (Chenault et al., 2006). These findings indicate that the process-based attention training facilitated learning during the writing lessons, demonstrating the potential benefit of combining process-based and strategy-based training procedures.
Except from the process-based versus strategy-based distinction, there are several other factors that should be considered when designing a training study, including the length of the training, the complexity of the task that is trained (i.e., does the task train one specific function or several different processes at once), the variability in stimuli and tasks (both within and between cognitive domains), and whether or not the difficulty level of the trained task(s) is adapted to the participants' level of performance. These factors depend strongly on the goal of the study (e.g., theory versus application). For instance, a study that examines theoretical questions about training-related changes in cognitive processes will benefit most from a simple training paradigm that controls for confounding variables (cf. Luna et al., 2010; Morrison and Chein, 2010). However, a study that aims to develop a cognitive intervention that is effective in practice might benefit more from a complex training paradigm. It has been suggested that training with complex and variable tasks will lead to greater generalization to real-life situations (Green and Bavelier, 2008; Lustig et al., 2009; Buschkuehl and Jaeggi, 2010). In addition, changing stimuli and adapting the difficulty level of the task are considered important methods to keep the participant motivated and to prevent automaticity (Green and Bavelier, 2008; Buschkuehl and Jaeggi, 2010; Klingberg, 2010; Morrison and Chein, 2010). There have only been a small number of studies in children that directly examined the influence of these factors and definitive conclusions have not yet been reached. For example, a number of studies have demonstrated that adaptive training led to greater training effects than non-adaptive training (Klingberg et al., 2002, 2005; Holmes et al., 2009a; Bergman Nutley et al., 2011; but see also Van der Molen et al., 2010), yet most of these studies used non-adaptive training with a very low difficulty. It is unclear whether adaptive training is still more successful than non-adaptive training if the latter is more challenging, and if so, what would be the optimal level of task difficulty to facilitate learning. In addition, the few studies that directly examined the effects of task variability did not find clear evidence that training with variable tasks will lead to greater generalization. For example, Karbach and Kray (2009) demonstrated that children who trained with different versions of the same task showed less transfer of training gain than children who trained with only one version. These findings were opposite of the findings in adults, who showed larger transfer effects in the variable training condition (Karbach and Kray, 2009). Furthermore, to examine whether generalizability would be larger for a training program that encompasses several cognitive domains than for training that is focused on one domain, Bergman Nutley et al. (2011) studied the effects of training both working memory and non-verbal reasoning relative to training only one of these functions. They demonstrated that the improvement on the specific functions was roughly proportionate to the amount of training in that particular domain, and there was no evidence of enhanced generalization if training was divided between cognitive domains. Future studies should further examine “success factors” (i.e., characteristics of the training paradigm that promote training gain and generalizability) and determine to which extent these factors are age-dependent.
Assessing Training Effectiveness: Dependent Variables
There are several ways to determine the effectiveness of the training, the most obvious being performance improvements (e.g., in accuracy or response times) on the trained task. Additional variables that could be studied include the frequency of a particular strategy that is employed, as well as the speed or proficiency with which that strategy is used (Willis and Schaie, 2009). If performance is measured throughout the training period, it is also possible to estimate a learning curve, which shows how the learning rate changes over time. Typically, the learning curve is steep at the beginning of training, but gradually becomes more flat when learning progresses (e.g., Jolles et al., 2010; Van der Molen et al., 2010; Loosli et al., 2011). The decreasing slope of performance improvements can partly be explained by the different aspects of the task that are being trained. For example, in the beginning of the training, participants might adopt a new strategy that improves performance dramatically. Later in training, performance improvements often slow down because participants are simply practicing with the same strategy over and over again. Moreover, in the beginning of training, a number of additional factors are introduced that are not directly related to the trained function of interest, including the equipment, the experimenter, and other aspects of the training context. Getting used to these extraneous factors contributes to the steep learning curve in the beginning of training. It is important to note that the learning curves of individual participants do not necessarily take the same form as the average curve of the group (Heathcote et al., 2000). Especially if there is a large variability in learning rate, the average learning curve of the group can be distorted, which suggests that individual curves should always be taken into account. Moreover, when comparing performance improvements between groups (e.g., children versus adults or children with developmental disabilities versus typically developing children), it is important to pay attention to performance differences before and after the training, as well as the room for improvement. Because it seems that performance improvements slow down when there is less room for improvement, the group that is closest to asymptotic performance will show less performance gains. In addition, it is possible that one group shows a larger improvement, while their maximal performance is still below that of the other group.
Besides performance improvements during the training, it is informative to examine the long-term effects of training, using a follow-up measurement several months after the training is completed (e.g., Klingberg et al., 2005; Holmes et al., 2009b; Beck et al., 2010; Jolles et al., 2010; Jaeggi et al., 2011). This follow-up test does not only examine the durability of training effects, but also tests for cumulative effects. That is, training gains may be enhanced during the follow-up test as a result of the secondary effects of training, including increased motivation or ability to learn. Some of these secondary effects (such as better school performance) require some time to establish (Holmes et al., 2009a; Van der Molen et al., 2010).
To rule out test-retest effects (e.g., Bors and Vigneau, 2001; Goodyear and Douglas, 2009; Jolles et al., 2010), it is important to compare the performance of the trained participants to that of a control group who did not participate in the training. Several studies have used a passive control group, which only participated in the pre- and posttraining sessions. Although a passive control group is useful to rule out the effects of familiarity, it does not take into account expectancy effects and motivation (see Box 1). To control for these effects, an active control group should be included, which receives a “placebo treatment”. Several placebo interventions have been proposed, including training the same task at a low difficulty (e.g., Klingberg et al., 2005; Holmes et al., 2009a; Bergman Nutley et al., 2011), watching videos (Rueda et al., 2005), and playing computer games (Shalev et al., 2007; Thorell et al., 2009). Yet, a control treatment is difficult to design because it should be very similar to the training program, but it must not be effective. Therefore, an alternative approach is to compare the effects of two training programs that focus on different cognitive functions (Thorell et al., 2009; Mackey et al., 2011).
A critical aspect to assess the generalizability of training benefits is the transfer of training effects to untrained tasks and real-life situations. Several studies have demonstrated near transfer of training effects to tasks within the same domain (e.g., Holmes et al., 2009b; Bergman Nutley et al., 2011; Mackey et al., 2011), and a number of studies have even found transfer to other domains, academic performance measures, or symptoms of inattention and hyperactivity (e.g., Klingberg et al., 2005; Rueda et al., 2005; Karbach and Kray, 2009; Dahlin, 2011; Loosli et al., 2011). However, transfer effects are highly inconsistent across studies, and the exact variables that lead to the transfer effects are still unclear. Perhaps this is due to the majority of studies focusing on the efficacy of the training, rather than why the training is effective, and what exactly is being transferred (Willis and Schaie, 2009). Yet, transfer effects are not only important from an intervention perspective. They can inform us about the underlying cognitive processes that change as a result of training. This is even important if one well-described task is being trained. Because of the “impurity” of executive function tasks (Miyake et al., 2000; Huizinga et al., 2006), there are many processes that can be influenced by training. For instance, if participants practice with a working memory task, training may lead to a general increase in processing efficiency (e.g., an increase of working memory capacity), a strategy change (e.g., the use of rehearsal to memorize items in working memory), or a task-specific skill (e.g., familiarity with the memory items). These processes can be disentangled if the participants also perform a number of transfer tasks that have one or more elements in common with the trained task. The use of a latent-variable approach can be particularly fruitful in this respect (Noack et al., 2009; Schmiedek et al., 2010; Bergman Nutley et al., 2011).
Training Effects in the Context of the Developing Brain
Children can improve their performance on cognitive control tasks as a result of training. This has been demonstrated both in healthy children (e.g., Karbach and Kray, 2009; Thorell et al., 2009; St. Clair-Thompson et al., 2010; Bergman Nutley et al., 2011; Loosli et al., 2011), and in children with cognitive or attentional impairments (e.g., Klingberg et al., 2005; Shalev et al., 2007; Bangirana et al., 2009; Holmes et al., 2009a; Mezzacappa and Buckner, 2010; Rabiner et al., 2010; Van der Molen et al., 2010). However, what does it mean if children reach more “mature” levels of performance, or if children with a developmental disability show “normalized” performance after training (cf. Karmiloff-Smith, 2009)? There are a few factors that should be taken into account, including the sensitivity and the ecological validity of the test, and the underlying processes that might be involved. That is, comparable test scores between groups do not necessarily mean that the groups use the same underlying cognitive processes and brain networks. Neuroscientific methods may add to this discussion by giving insight in the underlying mechanisms of cognitive plasticity and the relation between training effects and brain development.
According to Johnson (2001, 2011), there are three different viewpoints within the field of developmental cognitive neuroscience. First, the maturational viewpoint suggests that cognitive functions develop when the underlying brain regions reach maturity. In contrast, the second viewpoint, the interactive specialization account, suggests that the specialization of a particular brain region is a consequence of its interaction and competition with other brain regions over the course of development. This viewpoint has probably received the most support, as it takes into account the role of experience in brain maturation, suggesting that general rules of structural development might be genetically programmed, but specific details are the result of activity-dependent processes influenced by the environment (Changeux and Danchin, 1976; Greenough et al., 1987; Huttenlocher, 2002; Uylings, 2006). This account also points out that brain regions should always be viewed in relation to the functional networks in which they are involved. The third viewpoint is the skill-learning account, which emphasizes that the patterns of change observed during development are sometimes similar to those involved in skill acquisition in adults (Johnson, 2001; Casey et al., 2005; Johnson, 2011). This account argues that it is important to distinguish between the effects of age and performance in driving differences in brain activation between children and adults. Together, these viewpoints may be used to describe the effects of training in the developing brain.
In the following paragraphs, we describe three questions that are of particular importance when studying the effects of training in children and how these relate to the different viewpoints.
1. How are training effects influenced by the current stage of development?
Over the course of development, the human brain undergoes dramatic changes, driven by a series of progressive (e.g., myelination and strengthening of synapses) and regressive events (e.g., selective pruning of neurons and synaptic connections; e.g., Uylings, 2006; Stiles, 2008; Giedd and Rapoport, 2010). It is expected that the same training will have different outcomes in children and adults, depending on the nature of the function that is trained, and the brain structures and neuronal networks in which the changes take place (cf. Galvan, 2010; Kolb et al., 2010). While training in adults mainly modifies the existing neural architecture, in young children it may still influence the construction of neural networks (cf. Galvan, 2010), suggesting that there are both quantitatively and qualitatively different effects of training in children and adults.
On the one hand, an immature brain structure might set limits on how much can be achieved with practice. For example, the speed and efficiency of information processing are determined by the degree of myelination, and the pattern of synaptic connectivity (Goldman-Rakic, 1987; Chechik et al., 1998; Fields, 2008; Paus, 2010). This could, for instance, constrain practice-related gains on speeded control tasks or working memory (e.g., Case et al., 1982). Besides, training gains are limited by the stage of cognitive development (and thus by age and earlier experience). That is, a child cannot learn new skills if these skills build upon more primitive processes that are not yet mature (Zelazo, 2004). Thus, it is likely that there are particular cognitive processes that cannot be accelerated with training interventions. Therefore, it is expected that some age differences are actually magnified rather than reduced after training, which has also been demonstrated in training studies examining younger versus older adults (Baltes and Kliegl, 1992; Nyberg et al., 2003).
On the other hand, it has been suggested that in some cases, immaturity is actually advantageous (Ramscar and Gitcho, 2007; Bjorklund et al., 2009). For example, it has been argued that increasing specialization and integration in brain networks over the course of development goes at the expense of plasticity (Huttenlocher, 2003; Johnson, 2011). Or, as Thompson-Schill et al. (2009) put it: “a system optimized for performance may not be optimal for learning, and vice versa” (p. 260). Moreover, it has been suggested that there are “sensitive periods” in brain development during which specific experiences have their largest effects. Sensitive periods are most pronounced for basic sensory processes that occur during the first years of life, and they are expected to coincide with periods in which there is an abundance of neurons, axonal projections, and synaptic connections (Greenough et al., 1987; Huttenlocher, 2002; Uylings, 2006). With respect to higher cognitive functions, there is still a debate about the existence of sensitive periods. Because of the flexible nature of higher cognitive functions, these functions probably rely on neural mechanisms with life-long plasticity. Nevertheless, it is possible that the capacity for plasticity becomes smaller with age because of the increasing specificity of brain function (cf. Huttenlocher, 2003; Uylings, 2006; Johnson, 2011).
Finally, without denying the possible influence of time-specific biological processes, it is important to note that even (the onset and duration of) sensitive periods are largely influenced by experience (cf. Hensch, 2004). For example, it has been demonstrated that once a neural network is shaped by a particular environmental input, it is difficult to alter the neuronal connections by subsequent experience. These effects are independent of the age of the system (Munakata et al., 2004; Munakata and Pfaffly, 2004). At the same time, if the expected input is not yet received, the network may remain sensitive to new experience for a longer period (Hensch, 2004). Taken together, it seems that the periods of increased sensitivity to training effects are not simply guided by age, but rather by experience-related maturation (Hensch, 2004; Munakata et al., 2004; Munakata and Pfaffly, 2004).
2. Do training effects reflect long-lasting changes of brain structure or flexibility of brain function?
Besides the neural changes associated with memory of the trained material and the training itself, training-related changes in information processing are not necessarily caused by long-lasting alterations of the underlying brain structure. Performance improvements can also reflect flexibility of brain function that takes place within the limits of the current structural constraints of the brain (cf. Posner and Rothbart, 2005; Noack et al., 2009; Lövdén et al., 2010a). For instance, it has been suggested that the failure of young children to rehearse the items that are to be remembered during a working memory task often reflects a “production deficiency” (e.g., Flavell et al., 1966; Keeney et al., 1967). This indicates that children are able to apply the rehearsal strategy, but they do not always use it. Therefore, training may improve performance by encouraging children to use the strategy (e.g., Keeney et al., 1967; Ford et al., 1984), without inducing structural changes of the brain that increase working memory capacity directly.
Lövdén et al. (2010a) suggested that structural changes only take place when there is a mismatch between the environmental demands and the possibilities of the current structural system. For example, if children practice with a working memory task that requires them to hold more items in mind than they are able to (despite their use of rehearsal strategies), there is a mismatch between the demands of the training paradigm and the supply of the system (i.e., the working memory capacity). As a result, the training may increase working memory capacity by inducing plastic changes within the frontoparietal network that is involved in working memory (cf. Klingberg, 2010). The mismatch hypothesis might therefore explain why adaptive training can be more successful than non-adaptive training (Klingberg et al., 2002, 2005; Holmes et al., 2009a; Bergman Nutley et al., 2011). Noteworthy, it has been emphasized that a mismatch is a necessary, but not a sufficient condition for inducing long-term structural changes (Lövdén et al., 2010a). That is, some structural changes are not possible (e.g., working memory capacity cannot be increased infinitely). Moreover, it is important that the training is long enough for the specific structural changes to occur and that the training is not too difficult (Lövdén et al., 2010a). Finally, the degree to which plasticity is possible differs between individuals, depending on genetic factors and prior environmental influences.
3. How does training influence developmental trajectories?
It is important to consider the effect of training on the continuing developmental trajectory of the individual. First of all, training may simply “speed-up” development, such that cognitive processing/brain structure after training is more similar to that of older children (Figure 1, arrow A). This is in line with the idea that development is driven by an interaction between prespecified biological maturation and experience (Stiles, 2008) and the suggestion that development and learning can be regarded as two ends of the same continuum (Galvan, 2010). Yet, training and development do not necessarily involve the exact same underlying mechanisms. It has been argued that (early) development relies to a large extent on experience-expectant neural mechanisms, while training is more influenced by experience-dependent processes (cf. Galvan, 2010). As described by Greenough et al. (1987), experience-expectant mechanisms involve neural processes that occur during particular phases of development (such as the overproduction and subsequent pruning of neurons or synaptic connections), and are driven by environmental input that is common to all members of a species. Experience-dependent mechanisms on the other hand are driven by input that is more specific to an individual and involve neural processes that are available throughout lifetime (including the formation of new synapses and changes in the efficiency of synaptic contacts). The potential difference between developmental and training-related mechanisms suggests that training could influence cognitive processing/brain structure in a way that deviates from the typical developmental trajectory (Figure 1, arrow B).
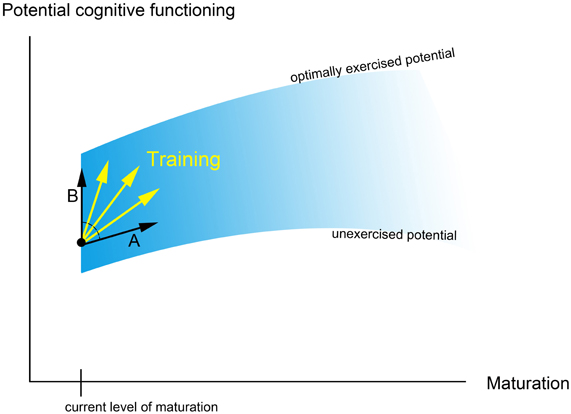
Figure 1. This figure shows a simplified, metaphorical description of how training might influence developmental trajectories [based on Denney (1984); see also Hertzog et al. (2009)]. The blue curve shows the potential of cognitive functioning, which increases with age due to maturational changes and common environmental experience. In addition, optimal environmental input and training determine whether the “optimally exercised potential” (i.e., the upper limit of cognitive functioning at a certain age; Denney, 1984) can be reached. Arrow A shows how training may improve cognitive functioning by speeding-up development; arrow B shows how training might improve functioning in a way that deviates from the typical developmental trajectory.
Neuroimaging methods might give insight in the different mechanisms that underlie typical development and training-related changes. For example, it has repeatedly been demonstrated that gray matter volume decreases during late childhood and adolescence (Sowell et al., 2001, 2003; Giedd, 2004; Gogtay et al., 2004). In contrast, adults who were learning to juggle (Draganski et al., 2004; Scholz et al., 2009), studied for exams (Draganski et al., 2006; Ceccarelli et al., 2009), or practiced mirror-reading (Ilg et al., 2008) showed increased gray matter volume in several of these areas (but see also Takeuchi et al., 2011). This suggests that on the one hand training in children may speed-up development and lead to decreased gray matter volume. On the other hand training may increase gray matter volume, like it often does in adults. Developmental training studies are needed to investigate the potential differences between typical development and training-related changes across a wide range of domains, and examine what are the long-term effects of training in terms of later developmental trajectories.
Finally, it has been argued that the “immature” brain structure actually has some important evolutionary benefits, and that speeding-up the development of cognitive control abilities in children might even have some disadvantages (cf. Bjorklund et al., 2009). For example, it has been suggested that language learning is only successful in neural networks with limited cognitive control and working memory (Newport, 1990; Elman, 1993; Ramscar and Gitcho, 2007; Thompson-Schill et al., 2009). Moreover, with advancing levels of expertise and knowledge, individuals usually develop certain routines, which might impair attentiveness and creativity (cf. Hertzog et al., 2009; Thompson-Schill et al., 2009). Yet, these findings do not necessarily mean that we should be reluctant to use training studies in childhood. It is expected that at each developmental stage there will be gains and losses (Willis and Schaie, 2009), and during childhood the gains of training will probably outweigh the losses. Nevertheless, the hypothesized disadvantages of training require further attention in the future.
Neuroimaging Studies of Cognitive Training
Neuroimaging methods provide a promising approach to increase our insight in the underlying mechanisms that drive training effects, and they can be used to make predictions about transfer effects (Dahlin et al., 2008). An additional advantage of neuroimaging data is that they can be analyzed along several dimensions (e.g., magnitude, location, or dynamics of activation and connectivity), which may result in increased sensitivity compared with behavioral measures (cf. Lustig et al., 2009). To describe the range of possible training outcomes irrespective of development, we start with a brief description of neuroimaging effects of training in adults, with a particular focus on the domain of working memory and cognitive control. For an extensive overview of training effects in the adult brain, we refer to prior reviews (Kelly and Garavan, 2005; Lustig et al., 2009; Buschkuehl et al., 2012).
Changes of brain activation
Depending on the cognitive and neural processes involved, cognitive training may lead to increased activation, reduced activation, and/or a change in the spatial pattern of activation (Poldrack, 2000; Jonides, 2004; Kelly and Garavan, 2005). It has been argued that simple process-based training often changes the level of activation within the functional network that was already recruited before practice (Chein and Schneider, 2005; Kelly and Garavan, 2005). The majority of cognitive training studies have demonstrated frontoparietal activation decreases in this respect, particularly if the training was very short (e.g., Garavan et al., 2000; Jansma et al., 2001; Landau et al., 2004; Tomasi et al., 2004; Sayala et al., 2006). Nevertheless, decreases have also been observed after longer training periods (Hempel et al., 2004; Schneiders et al., 2011). There are several possible explanations for these activation decreases, including reduced reliance on executive control and error monitoring, increased speed of processing, repetition priming (i.e., implicit memory for task stimuli leading to faster identification), and/or increased specificity of neuronal responses in the underlying neural network (cf. Poldrack, 2000). Yet, the magnitude and direction of training-related activation changes probably depend on specific task demands and the difficulty level of the task (Jolles et al., 2010). It has been hypothesized that cognitive training should only result in reduced activation if the task is within capacity limits (cf. Nyberg et al., 2009). This might explain why young adults showed frontoparietal activation decreases after training in working memory updating (in addition to increased activation in the striatum), while older adults–who likely had a lower working memory capacity–showed activation increases (Dahlin et al., 2008). Moreover, when task load was dynamically adapted to the ability of participants (i.e., by increasing the number of items to be held in working memory), increased frontoparietal activation has also been found in young adults (Olesen et al., 2004; but see also Schneiders et al., 2011). More specifically, the authors found training-related activation increases in middle frontal gyrus and superior and inferior parietal cortices (along with decreases in the cingulate cortex), which they attributed to an increase of working memory capacity (Olesen et al., 2004; Klingberg, 2010).
When participants learn to employ a new strategy, a change in the spatial pattern of functional activation is often observed (cf. Poldrack, 2000; Chein and Schneider, 2005; Kelly and Garavan, 2005). Furthermore, it has been suggested that the use of new strategies may lead to increased activation in frontoparietal control regions, even when these strategies lessen task demands (Bor and Owen, 2007b). For example, in a series of experiments Bor et al. (2004; Bor and Owen, 2007a) showed that when participants used chunking strategies to maintain information in working memory, frontoparietal activation increased, although task difficulty decreased. In addition, it has been demonstrated that when participants were trained in using semantic or visuospatial strategies for the encoding of word lists, they showed improved recall and increased activation in frontal and/or occipitoparietal cortex (Nyberg et al., 2003; Miotto et al., 2006). Finally, a strategy change may also induce a shift in the dynamics of activation. For example, using a short strategy training in a group of older adults, Braver et al. (2009) demonstrated a shift from probe-based to cue-based activation in prefrontal cortex regions. This shift was interpreted as a change from a reactive toward a more proactive control mode.
Changes of functional connectivity
In addition to changes in the level of activation within regions, training can also induce changes in the interaction between regions. Such interactions can be studied using functional connectivity (i.e., temporal correlations of blood oxygen level dependent (BOLD) signal fluctuations between brain regions) and effective connectivity (i.e., the influence that one region exerts over another) (for a detailed discussion of these concepts, see Friston, 1994). For example, connectivity changes have been observed during artificial grammar learning (Fletcher et al., 1999), repetition suppression (Buchel et al., 1999), visual categorization learning (DeGutis and D'Esposito, 2009), and in experts versus non-experts during a creativity task (Kowatari et al., 2009). Moreover, training-related changes of functional connectivity have been observed during resting-state (Albert et al., 2009; Lewis et al., 2009; Jolles et al., 2011), suggesting that changes of interregional interactions are not necessarily specific to task conditions. For example, Jolles et al. (2011) showed that practice with a working memory task changed functional connectivity during a rest period preceding the task. More specifically, regions of the frontoparietal task network showed increased resting-state functional connectivity after training, whereas regions of the default mode network showed reduced functional connectivity after training. Future studies should examine whether these changes were associated with repeated co-activation during the practice period or with preparatory processes regarding the upcoming task.
Changes of brain structure
It remains to be determined to which extent changes of brain activation or functional connectivity are directly related to changes of the underlying brain structure. Functional changes could be associated with a multitude of different structural changes, including changes in the number or efficacy of synapses, myelination, and changes of hormone or neurotransmitter systems. However, only a subset of structural changes can be observed using neuroimaging methods (cf. Poldrack, 2000). For example, a number of studies have demonstrated changes in gray- and/or white matter structure (Draganski et al., 2006; Ceccarelli et al., 2009; Lövdén et al., 2010b; Takeuchi et al., 2010; Garavan et al., 2000), and in the density of dopamine receptors (McNab et al., 2009). Interestingly, one study demonstrated a correspondence between regions that were activated during the trained task (i.e., mirror reading), regions that showed practice-related activation increases, and regions that showed changes of gray matter volume (Ilg et al., 2008). However, it is important to note that these results do not automatically imply causality, and further studies are necessary to specify the interaction between functional and structural changes as a result of training.
Training the Developing Brain
In general, practice may induce similar changes of brain function (or structure) in children as are seen in adults, including reduced activation with increasing automaticity, and a reorganization of neural activation after a strategy change. Yet, it is important to acknowledge that the child brain is not just a simplified, less efficient version of the adult brain (cf. Poldrack, 2010). As described in the section about Training effects in the context of the developing brain, training in children may speed-up developmental change, such that brain function is more similar to adult brain function after training. Yet, training could also have qualitatively different effects in children and adults.
There are only a few neuroscientific studies that examined activation changes after cognitive training in children. The first set of studies has demonstrated that training may speed-up developmental changes, such that neural activation in children is more similar to that of older children or adults. For instance, it has been suggested that children show a more “mature” pattern of frontoparietal brain activation after working memory practice (Jolles et al., 2012). Previously, it had been demonstrated that 8–12-year-old children did not show increased activation for manipulation of information in working memory above and beyond the regions they used for pure maintenance (Crone et al., 2006). However, after six weeks of practice, children showed increased activation in the frontoparietal network for manipulation relative to maintenance, arguing against the hypothesis that these regions were “inaccessible” due to immature neural circuitry (Jolles et al., 2012). A similar effect has been described for 6-year-old children who participated in training of executive attention (Rueda et al., 2005). After training, the children showed a more adult-like scalp distribution of event-related potentials (ERPs) than children of a control group. Notably, this study also pointed out that there might be limits on the effects of practice in childhood, as 4-year-olds did not show this effect (Rueda et al., 2005). These findings suggest that training of a particular brain function requires a certain stage of cognitive and/or structural brain development.
There are also studies indicating that children and adults process practiced information differently than adults. For example, after practicing for several days with algebra, children showed reduced activation in prefrontal and parietal cortex and increased activation in left putamen (Qin et al., 2004). In contrast, adults who practiced with a similar task only showed reduced prefrontal activation (Qin et al., 2003). It remains to be determined whether these results indicate increased plasticity, or whether they are related to immature processing in children (Luna, 2004). One study specifically examined the link between activation and changes of the underlying brain structure (Haier et al., 2009). In this study, adolescent girls practiced for three months with a visuospatial computer game (tetris). After practice, they showed increased cortical thickness in superior frontal and temporal areas, as well as decreased activation in frontal and parietal areas. Training-related activation changes did not overlap with changes of cortical thickness, suggesting that changes of activation are not necessarily the result of structural changes in the same location.
Finally, a number of studies have examined the malleability of brain function in children with developmental disabilities, such as attention deficit hyperactivity disorder (ADHD), developmental dyscalculia (i.e., a specific deficit in learning mathematics), and dyslexia. For instance, it has been demonstrated that cognitive training changes task performance and brain activation in children diagnosed with ADHD (Hoekzema et al., 2010). The authors emphasized that the training-related activation changes were found in syndrome-associated brain regions in frontal lobe and cerebellum, which are also target of psychostimulant medication. These findings point out the potential benefit of cognitive training as part of ADHD-treatment (cf. Hoekzema et al., 2010). Another study examined how children with and without developmental dyscalculia responded to mental number line training (Kucian et al., 2011). After training, both groups showed improved performance, as well as decreased activation in task-related areas. The decrease was stronger in children with developmental dyscalculia. This seems contradictive with the group differences before training, when children with developmental dyscalculia showed less activation compared to typically developing children. Yet, follow-up results in a subgroup of the dyscalculics indicated that there might be a normalization of brain function after a few weeks. However, it should be noted that these results were based on only seven children and require validation in future research. Neural activation changes have also been observed in children with language disorders, including reading disability, dyslexia, and specific language impairment (Simos et al., 2002; Aylward et al., 2003; Temple et al., 2003; Shaywitz et al., 2004; Stevens et al., 2008). Interestingly, Stevens et al. (2008) showed that language training did not only improve standardized measures of receptive language, it also influenced neural mechanisms related to auditory attention. That is, children with specific language impairment showed an increase in the ERP component associated with selective auditory attention after training. These findings are in line with the idea that language interventions might improve language skills in part by training domain-general systems such as attention or memory, which provides an interesting direction for future research (Stevens et al., 2008). Furthermore, future studies in children with developmental disabilities should examine the extent to which early interventions can change or even normalize developmental trajectories in later childhood or adolescence. Long-term effects are one of the most important measures to determine the effectiveness of training programs that are developed for intervention purposes.
Critical Considerations and Future Directions
In the present article, we suggested that training effects are better understood in the context of the developing brain, because they emerge from a dynamic interaction between learning and brain maturation (cf. Galvan, 2010). In addition, by providing a short overview of the effects of neurocognitive training studies, we illustrated how neuroimaging methods can contribute to our understanding of the underlying cognitive and neural processes that are involved during training. In this paragraph, we point out the issues that warrant further attention in future research.
Neuroimaging Methods: Confounds and Considerations
We have described how neuroimaging tools can be valuable in providing additive insights in the underlying cognitive and neural processes that are involved in training. In addition, neuroimaging data may be more sensitive than behavioral measures (cf. Lustig et al., 2009). However, a serious challenge is the complexity of the results. There are multiple cognitive and neural mechanisms that can drive changes in activation or brain structure, and these mechanisms might be different for children and adults. Thus, even if developmental and experience-related changes are similar, they are not necessarily caused by the same cognitive or neural processes (cf. Klingberg, 2006). Moreover, there is a number of confounding factors that further complicate the interpretation of activation changes after practice, including changes in task performance, scanner instability, or reduced anxiety (Box 1). Therefore, it is important to perform theory-driven experiments with well-described tasks and to control for variables that are not of interest (Poldrack, 2000; Luna et al., 2010; Crone and Ridderinkhof, 2011). In addition, human training studies might be conducted in parallel with animal studies and/or with neural network modeling to create hypotheses about the underlying anatomical, histological, and neurochemical processes that are involved during training. Prior studies have already demonstrated the value of computational modeling in describing how plasticity and learning may differ between children adults (e.g., Elman, 1993; Thomas and Karmiloff-Smith, 2002). In the future, it will be of great value to combine computational modeling with neuroimaging methods to create predictions about training-related changes in the BOLD signal (Macoveanu et al., 2006; Edin et al., 2007, 2009).
Individual Differences and Environmental Factors
We pointed out that inter- and intraindividual differences in training outcome depend on an interaction between genetic differences and prior experience. Individual differences might be evident in the ability to learn from training, the rate of learning, and the maximum level of cognitive functioning that can be achieved (cf. Mercado, 2008; Willis and Schaie, 2009). Moreover, individual differences in training gain have been shown to moderate transfer effects (Jaeggi et al., 2011). One important focus for future research involves the characterization of individual and environmental factors that define differences in training gain, and to determine how these factors are related to differences in brain function and structural brain maturation. Studies in adults have already demonstrated that individual differences in internalized beliefs and goals can influence learning success and that these differences are related to differences in the ERP response (e.g., Mangels et al., 2006). Moreover, there are indications that individual differences in brain structure predict performance improvements (Golestani et al., 2002; Erickson et al., 2010). In children, these mechanisms might even be more complex. Shaw et al. (2006) demonstrated that there are differences between children in the trajectory of cortical development, with more intelligent children showing a prolonged phase of structural brain maturation compared with less intelligent children. These findings indicate that individual differences in training gain could be influenced by the “maturity” of the underlying brain structure, regardless of the child's age.
Another factor that should be considered when examining training gain is the input from the environment that an individual receives (both in terms of schooling and positive or negative reinforcement). For example, it has been argued that children who receive optimal education and stimulation have a large “actualized genetic potential” (Bronfenbrenner and Ceci, 1994), which suggests that extra training will have less additional value. This may explain why cognitive intervention programs are particularly effective in children from a low socioeconomic background (Brooks-Gunn et al., 1992; Mezzacappa and Buckner, 2010; Mackey et al., 2011). In a similar vein, it has been argued that functions that are frequently practiced in every-day situations might be more difficult to train than less practiced functions (Denney, 1984). Moreover, according to the time displacement hypothesis (e.g., Bavelier et al., 2010), training may even lead to negative effects if the activities it displaces are more beneficial than the training itself.
Summary and Conclusion
We aimed to show in this review that training studies provide important tools in studying the possibilities and limitations of cognitive functioning over the course of childhood. We described that training effects in the developing brain are driven by a complex interaction between learning, brain development, genetic differences and prior experience. Depending on the type of training and the level of maturation of the individual, training may speed-up development; improve the individual's actualized genetic potential; or both. The immature brain structure can set limits on how much can be achieved with training, but in some cases these same limitations could be an advantage. We argued that neuroimaging methods have a great potential to improve our understanding of the interaction between learning and brain development. Rather than examining whether training studies are effective, neuroimaging studies may provide insight into how training interventions are effective. Yet, there is a still number of challenges and confounds to overcome.
Although we must be careful when translating scientific research to practical applications (Bruer, 1997; Goswami, 2006), neurocognitive training studies have potential for application in practice. Eventually, they might aid in designing education programs and interventions for normally developing children or children with developmental disabilities (Posner and Rothbart, 2005; Goswami, 2006; Carew and Magsamen, 2010). For example, to optimize education programs, it is valuable to know more about how children at different ages learn a particular skill, how the underlying neural circuitry supports different kinds of learning, and whether the learning-related changes reflect flexibility in brain function or more permanent changes of the underlying brain structure (Posner and Rothbart, 2005; Goswami, 2006; Carew and Magsamen, 2010). In addition, knowledge about children's abilities to learn might yield insights about specific learning problems, as seen for example in children with dyslexia, or ADHD. When the underlying cause of children's learning difficulties is better understood, it might be possible to target intervention to remediate these difficulties (Goswami, 2006).
Conflict of Interest Statement
The authors declare that the research was conducted in the absence of any commercial or financial relationships that could be construed as a potential conflict of interest.
Acknowledgments
This work was supported by a VIDI grant from the Netherlands Organization for Scientific Research (NWO 452-07-011 VIDI, Eveline A. Crone). The authors would like to thank Jelle Jolles for his valuable feedback on the manuscript. In addition, they would like to thank the reviewers for their useful comments and suggestions.
References
Albert, N. B., Robertson, E. M., and Miall, R. C. (2009). The resting human brain and motor learning. Curr. Biol. 19, 1023–1027.
Aylward, E. H., Richards, T. L., Berninger, V. W., Nagy, W. E., Field, K. M., Grimme, A. C., Richards, A. L., Thomson, J. B., and Cramer, S. C. (2003). Instructional treatment associated with changes in brain activation in children with dyslexia. Neurology 61, 212–219.
Baltes, P. B., and Kliegl, R. (1992). Further testing of limits of cognitive plasticity: negative age differences in a mnemonic skill are robust. Dev. Psychol. 28, 121–125.
Bangirana, P., Giordani, B., John, C. C., Page, C., Opoka, R. O., and Boivin, M. J. (2009). Immediate neuropsychological and behavioral benefits of computerized cognitive rehabilitation in Ugandan pediatric cerebral malaria survivors. J. Dev. Behav. Pediatr. 30, 310–318.
Bavelier, D., Green, C. S., and Dye, M. W. (2010). Children, wired: for better and for worse. Neuron 67, 692–701.
Beck, S. J., Hanson, C. A., Puffenberger, S. S., Benninger, K. L., and Benninger, W. B. (2010). A controlled trial of working memory training for children and adolescents with ADHD. J. Clin. Child Adolesc. Psychol. 39, 825–836.
Bergman Nutley, S., Soderqvist, S., Bryde, S., Thorell, L. B., Humphreys, K., and Klingberg, T. (2011). Gains in fluid intelligence after training non-verbal reasoning in 4-year-old children: a controlled, randomized study. Dev. Sci. 14, 591–601.
Bjorklund, D. F., Periss, V., and Causey, K. (2009). The benefits of youth. Eur. J. Dev. Psychol. 6, 120–137.
Bor, D., Cumming, N., Scott, C. E. L., and Owen, A. M. (2004). Prefrontal cortical involvement in verbal encoding strategies. Eur. J. Neurosci. 19, 3365–3370.
Bor, D., Duncan, J., Wiseman, R. J., and Owen, A. M. (2003). Encoding strategies dissociate prefrontal activity from working memory demand. Neuron 37, 361–367.
Bor, D., and Owen, A. M. (2007a). A common prefrontal-parietal network for mnemonic and mathematical recoding strategies within working memory. Cereb. Cortex. 17, 778–786.
Bor, D., and Owen, A. M. (2007b). Cognitive training: neural correlates of expert skills. Curr. Biol. 17, R95–R97.
Bors, D. A., and Vigneau, F. (2001). The effect of practice on Raven's advanced progressive matrices. Learn. Individ. Differ. 13, 291–312.
Braver, T. S., Paxton, J. L., Locke, H. S., and Barch, D. M. (2009). Flexible neural mechanisms of cognitive control within human prefrontal cortex. Proc. Natl. Acad. Sci. U.S.A. 106, 7351–7356.
Brefczynski-Lewis, J. A., Lutz, A., Schaefer, H. S., Levinson, D. B., and Davidson, R. J. (2007). Neural correlates of attentional expertise in long-term meditation practitioners. Proc. Natl. Acad. Sci. U.S.A. 104, 11483–11488.
Bronfenbrenner, U., and Ceci, S. J. (1994). Nature-nurture reconceptualized in developmental perspective: a bioecological model. Psychol. Rev. 101, 568–586.
Brooks-Gunn, J., Gross, R. T., Kraemer, H. C., Spiker, D., and Shapiro, S. (1992). Enhancing the cognitive outcomes of low birth weight, premature infants: for whom is the intervention most effective? Pediatrics 89, 1209.
Bryck, R. L., and Fisher, P. A. (2012). Training the brain: practical applications of neural plasticity from the intersection of cognitive neuroscience, developmental psychology, and prevention science. Am. Psychol. 67, 87–100.
Buchel, C., Coull, J. T., and Friston, K. J. (1999). The predictive value of changes in effective connectivity for human learning. Science 283, 1538–1541.
Buckner, R. L., Ndrews-Hanna, J. R., and Schacter, D. L. (2008). The brain's default network: anatomy, function, and relevance to disease. Ann. N.Y. Acad. Sci. 1124, 1–38.
Buschkuehl, M., and Jaeggi, S. M. (2010). Improving intelligence: a literature review. Swiss Med. Wkly. 140, 266–272.
Buschkuehl, M., Jaeggi, S. M., and Jonides, J. (2012). Neuronal effects following working memory training. Dev. Cogn. Neurosci. 2, S167–S179.
Carew, T. J., and Magsamen, S. H. (2010). Neuroscience and education: an ideal partnership for producing evidence-based solutions to Guide 21(st) Century Learning. Neuron 67, 685–688.
Case, R., Kurland, D. M., and Goldberg, J. (1982). Operational efficiency and the growth of short-term memory span. J. Exp. Child Psychol. 33, 386–404.
Casey, B. J., Tottenham, N., Liston, C., and Durston, S. (2005). Imaging the developing brain: what have we learned about cognitive development? Trends Cogn. Sci. 9, 104–110.
Ceccarelli, A., Rocca, M. A., Pagani, E., Falini, A., Comi, G., and Filippi, M. (2009). Cognitive learning is associated with gray matter changes in healthy human individuals: a tensor-based morphometry study. Neuroimage 48, 585–589.
Changeux, J. P., and Danchin, A. (1976). Selective stabilisation of developing synapses as a mechanism for the specification of neuronal networks. Nature 264, 705–712.
Chechik, G., Meilijson, I., and Ruppin, E. (1998). Synaptic pruning in development: a computational account. Neural Comput. 10, 1759–1777.
Chein, J. M., and Schneider, W. (2005). Neuroimaging studies of practice-related change: fMRI and meta-analytic evidence of a domain-general control network for learning. Brain Res. Cogn. Brain Res. 25, 607–623.
Chenault, B., Thomson, J., Abbott, R. D., and Berninger, V. W. (2006). Effects of prior attention training on child dyslexics' response to composition instruction. Dev. Neuropsychol. 29, 243–260.
Church, J. A., Petersen, S. E., and Schlaggar, B. L. (2010). The “Task B problem” and other considerations in developmental functional neuroimaging. Hum. Brain Mapp. 31, 852–862.
Conners, F. A., Rosenquist, C. J., Arnett, L., Moore, M. S., and Hume, L. E. (2008). Improving memory span in children with Down syndrome. J. Intellect. Disabil. Res. 52, 244–255.
Crone, E. A., and Ridderinkhof, R. K. (2011). The developing brain: from theory to neuroimaging and back. Dev. Cogn. Neurosci. 1, 101–109.
Crone, E. A., Wendelken, C., Donohue, S., van Leijenhorst, L., and Bunge, S. A. (2006). Neurocognitive development of the ability to manipulate information in working memory. Proc. Natl. Acad. Sci. U.S.A. 103, 9315–9320.
Dahlin, K. I. E. (2011). Effects of working memory training on reading in children with special needs. Reading Writ. 24, 479–491.
Dahlin, E., Neely, A. S., Larsson, A., Backman, L., and Nyberg, L. (2008). Transfer of learning after updating training mediated by the striatum. Science 320, 1510–1512.
DeGutis, J., and D'Esposito, M. (2009). Network changes in the transition from initial learning to well-practiced visual categorization. Front. Hum. Neurosci. 3:44. doi: 10.3389/neuro.09.044.2009
Diamond, A., and Lee, K. (2011). Interventions shown to aid executive function development in children 4 to 12 years old. Science 333, 959–964.
Draganski, B., Gaser, C., Busch, V., Schuierer, G., Bogdahn, U., and May, A. (2004). Neuroplasticity: changes in grey matter induced by training. Nature 427, 311–312.
Draganski, B., Gaser, C., Kempermann, G., Kuhn, H. G., Winkler, J., Buchel, C., and May, A. (2006). Temporal and spatial dynamics of brain structure changes during extensive learning. J. Neurosci. 26, 6314–6317.
Duncan, J., and Owen, A. M. (2000). Common regions of the human frontal lobe recruited by diverse cognitive demands. Trends Neurosci. 23, 475–483.
Edin, F., Klingberg, T., Johansson, P., McNab, F., Tegner, J., and Compte, A. (2009). Mechanism for top-down control of working memory capacity. Proc. Natl. Acad. Sci. U.S.A. 106, 6802–6807.
Edin, F., Macoveanu, J., Olesen, P., Tegner, J., and Klingberg, T. (2007). Stronger synaptic connectivity as a mechanism behind development of working memory-related brain activity during childhood. J. Cogn. Neurosci. 19, 750–760.
Elbert, T., Pantev, C., Wienbruch, C., Rockstroh, B., and Taub, E. (1995). Increased cortical representation of the fingers of the left hand in string players. Science 270, 305–307.
Elman, J. L. (1993). Learning and development in neural networks: the importance of starting small. Cognition 48, 71–99.
Erickson, K. I., Boot, W. R., Basak, C., Neider, M. B., Prakash, R. S., Voss, M. W., Graybiel, A. M., Simons, D. J., Fabiani, M., and Gratton, G. (2010). Striatal volume predicts level of video game skill acquisition. Cereb. Cortex 20, 2522.
Fields, R. D. (2008). White matter in learning, cognition and psychiatric disorders. Trends Neurosci. 31, 361–370.
Flavell, J. H., Beach, D. R., and Chinsky, J. M. (1966). Spontaneous verbal rehearsal in a memory task as a function of age. Child Dev. 37, 283–299.
Fletcher, P., Buchel, C., Josephs, O., Friston, K., and Dolan, R. (1999). Learning-related neuronal responses in prefrontal cortex studied with functional neuroimaging. Cereb. Cortex 9, 168–178.
Ford, C. E., Pelham, W. E., and Ross, A. O. (1984). Selective attention and rehearsal in the auditory short-term memory task performance of poor and normal readers. J. abnorm. child psychol. 12, 127–141.
Friston, K. (1994). Functional and effective connectivity in neuroimaging: a synthesis. Hum. Brain Mapp. 2, 56–78.
Garavan, H., Kelley, D., Rosen, A., Rao, S. M., and Stein, E. A. (2000). Practice-related functional activation changes in a working memory task. Microsc. Res. Tech. 51, 54–63.
Gaser, C., and Schlaug, G. (2003). Brain structures differ between musicians and non-musicians. J. Neurosci. 23, 9240–9245.
Ghatala, E. S., Levin, J. R., Pressley, M., and Lodico, M. G. (1985). Training cognitive strategy-monitoring in children. Am. Educ. Res. J. 22, 199.
Giedd, J. N. (2004). Structural magnetic resonance imaging of the adolescent brain. Ann. N.Y. Acad. Sci. 1021, 77–85.
Giedd, J. N., and Rapoport, J. L. (2010). Structural MRI of pediatric brain development: what have we learned and where are we going? Neuron 67, 728–734.
Gogtay, N., Giedd, J. N., Lusk, L., Hayashi, K. M., Greenstein, D., Vaituzis, A. C., Nugent, T. F., Herman, D. H., Clasen, L. S., Toga, A. W., Rapoport, J. L., and Thompson, P. M. (2004). Dynamic mapping of human cortical development during childhood through early adulthood. Proc. Natl. Acad. Sci. U.S.A. 101, 8174–8179.
Goldman-Rakic, P. S. (1987). Development of cortical circuitry and cognitive function. Child Dev. 58, 601–622.
Golestani, N., Paus, T., and Zatorre, R. J. (2002). Anatomical correlates of learning novel speech sounds. Neuron 35, 997–1010.
Goodyear, B. G., and Douglas, E. A. (2009). Decreasing task-related brain activity over repeated functional MRI scans and sessions with no change in performance: implications for serial investigations. Exp. Brain Res. 192, 231–239.
Goswami, U. (2006). Neuroscience and education: from research to practice? Nat. Rev. Neurosci. 7, 406–411.
Green, C. S., and Bavelier, D. (2008). Exercising your brain: a review of human brain plasticity and training-induced learning. Psychol. Aging 23, 692–701.
Greenough, W. T., Black, J. E., and Wallace, C. S. (1987). Experience and brain development. Child Dev. 58, 539–559.
Haier, R. J., Karama, S., Leyba, L., and Jung, R. E. (2009). MRI assessment of cortical thickness and functional activity changes in adolescent girls following three months of practice on a visual-spatial task. BMC Res. Notes 2, 174.
Heathcote, A., Brown, S., and Mewhort, D. J. (2000). The power law repealed: the case for an exponential law of practice. Psychon. Bull. Rev. 7, 185–207.
Hempel, A., Giesel, F. L., Garcia Caraballo, N. M., Amann, M., Meyer, H., Wustenberg, T., Essig, M., and Schroder, J. (2004). Plasticity of cortical activation related to working memory during training. Am. J. Psychiatry 161, 745–747.
Hertzog, C., Kramer, A. F., Wilson, R. S., and Lindenberger, U. (2009). Enrichment effects on adult cognitive development. Can the functional capacity of older adults be preserved and enhanced? Psychol. Sci. Public Interest 9, 1–65.
Hoekzema, E., Carmona, S., Tremols, V., Gispert, J. D., Guitart, M., Fauquet, J., Rovira, M., Bielsa, A., Soliva, J. C., Tomas, X., Bulbena, A., Ramos-Quiroga, A., Casas, M., Tobena, A., and Vilarroya, O. (2010). Enhanced neural activity in frontal and cerebellar circuits after cognitive training in children with attention-deficit/hyperactivity disorder. Hum. Brain Mapp. 31, 1942–1950.
Holmes, J., Gathercole, S. E., and Dunning, D. L. (2009a). Adaptive training leads to sustained enhancement of poor working memory in children. Dev. Sci. 12, F9–F15.
Holmes, J., Gathercole, S. E., Dunning, D. L., Hilton, K. A., and Elliott, J. G. (2009b). Working memory deficits can be overcome: impacts of training and medication on working memory in children with ADHD. Appl. Cogn. Psychol. 24, 827–836.
Huizinga, M., Dolan, C. V., and Van der Molen, M. W. (2006). Age-related change in executive function: developmental trends and a latent variable analysis. Neuropsychologia 44, 2017–2036.
Huttenlocher, P. R. (2002). Neural Plasticity: The Effects of Environment on the Development of the Cerebral Cortex (Perspectives in Cognitive Neuroscience). Cambridge, MA: Harvard University Press.
Huttenlocher, P. R. (2003). Basic neuroscience research has important implications for child development. Nat. Neurosci. 6, 541–541.
Ilg, R., Wohlschlager, A. M., Gaser, C., Liebau, Y., Dauner, R., Woller, A., Zimmer, C., Zihl, J., and Muhlau, M. (2008). Gray matter increase induced by practice correlates with task-specific activation: a combined functional and morphometric magnetic resonance imaging study. J. Neurosci. 28, 4210–4215.
Jaeggi, S. M., Buschkuehl, M., Jonides, J., and Shah, P. (2011). Short- and long-term benefits of cognitive training. Proc. Natl. Acad. Sci. U.S.A. 108, 10081–10086.
Jansma, J. M., Ramsey, N. F., Slagter, H. A., and Kahn, R. S. (2001). Functional anatomical correlates of controlled and automatic processing. J. Cogn. Neurosci. 13, 730–743.
Johnson, M. H. (2011). Interactive specialization: a domain-general framework for human functional brain development? Dev. Cogn. Neurosci. 1, 7–21.
Johnstone, S. J., Roodenrys, S., Phillips, E., Watt, A. J., and Mantz, S. (2010). A pilot study of combined working memory and inhibition training for children with AD/HD. Atten. Defic. Hyperact. Disord. 2, 31–42.
Jolles, D. D., Grol, M. J., van Buchem, M. A., Rombouts, S. A., and Crone, E. A. (2010). Practice effects in the brain: changes in cerebral activation after working memory practice depend on task demands. Neuroimage 52, 658–668.
Jolles, D. D., van Buchem, M. A., Crone, E. A., and Rombouts, S. A. (2011). Functional brain connectivity at rest changes after working memory training. Hum. Brain Mapp. doi: 10.1002/hbm.21444. [Epub ahead of print]
Jolles, D. D., van Buchem, M. A., Rombouts, S. A. R. B., and Crone, E. A. (2012). Practice effects in the developing brain: a pilot study. Dev. Cogn. Neurosci. 2S, S180–S191.
Karbach, J., and Kray, J. (2009). How useful is executive control training? Age differences in near and far transfer of task-switching training. Dev. Sci. 12, 978–990.
Karmiloff-Smith, A. (2009). Nativism versus neuroconstructivism: rethinking the study of developmental disorders. Dev. Psychol. 45, 56–63.
Keeney, T. J., Cannizzo, S. R., and Flavell, J. H. (1967). Spontaneous and induced verbal rehearsal in a recall task. Child Dev. 38, 953–966.
Kelly, A. M., and Garavan, H. (2005). Human functional neuroimaging of brain changes associated with practice. Cereb. Cortex 15, 1089–1102.
Klingberg, T. (2006). Development of a superior frontal-intraparietal network for visuo-spatial working memory. Neuropsychologia 44, 2171–2177.
Klingberg, T., Fernell, E., Olesen, P. J., Johnson, M., Gustafsson, P., Dahlstrom, K., Gillberg, C. G., Forssberg, H., and Westerberg, H. (2005). Computerized training of working memory in children with ADHD–a randomized, controlled trial. J. Am. Acad. Child Adolesc. Psychiatry 44, 177–186.
Klingberg, T., Forssberg, H., and Westerberg, H. (2002). Training of working memory in children with ADHD. J. Clin. Exp. Neuropsychol. 24, 781–791.
Kolb, B., Teskey, G. C., and Gibb, R. (2010). Factors influencing cerebral plasticity in the normal and injured brain. Front. Hum. Neurosci. 4:204. doi: 10.3389/fnhum.2010.00204
Kowatari, Y., Lee, S. H., Yamamura, H., Nagamori, Y., Levy, P., Yamane, S., and Yamamoto, M. (2009). Neural networks involved in artistic creativity. Hum. Brain Mapp. 30, 1678–1690.
Kramarski, B., and Mevarech, Z. R. (2003). Enhancing mathematical reasoning in the classroom: the effects of cooperative learning and metacognitive training. Am. Educ. Res. J. 40, 281.
Kucian, K., Grond, U., Rotzer, S., Henzi, B., Schonmann, C., Plangger, F., Galli, M., Martin, E., and von Aster, M. (2011). Mental number line training in children with developmental dyscalculia. Neuroimage 57, 782–795.
Landau, S. M., Schumacher, E. H., Garavan, H., Druzgal, T. J., and D'esposito, M. (2004). A functional MRI study of the influence of practice on component processes of working memory. Neuroimage 22, 211–221.
Lewis, C. M., Baldassarre, A., Committeri, G., Romani, G. L., and Corbetta, M. (2009). Learning sculpts the spontaneous activity of the resting human brain. Proc. Natl. Acad. Sci. U.S.A. 106, 17558–17563.
Loosli, S. V., Buschkuehl, M., Perrig, W. J., and Jaeggi, S. M. (2011). Working memory training improves reading processes in typically developing children. Child Neuropsychol. 1–17.
Lövdén, M., Backman, L., Lindenberger, U., Schaefer, S., and Schmiedek, F. (2010a). A theoretical framework for the study of adult cognitive plasticity. Psychol. Bull. 136, 659–676.
Lövdén, M., Bodammer, N. C., Kuhn, S., Kaufmann, J., Schutze, H., Tempelmann, C., Heinze, H. J., Duzel, E., Schmiedek, F., and Lindenberger, U. (2010b). Experience-dependent plasticity of white-matter microstructure extends into old age. Neuropsychologia 48, 3878–3883.
Luna, B., Velanova, K., and Geier, C. F. (2010). Methodological approaches in developmental neuroimaging studies. Hum. Brain Mapp. 31, 863–871.
Lustig, C., Shah, P., Seidler, R., and Reuter-Lorenz, P. A. (2009). Aging, training, and the brain: a review and future directions. Neuropsychol. Rev. 19, 504–522.
Lutz, A., Brefczynski-Lewis, J., Johnstone, T., and Davidson, R. J. (2008). Regulation of the neural circuitry of emotion by compassion meditation: effects of meditative expertise. PLoS One 3:e1897. doi: 10.1371/journal.pone.0001897
Mackey, A. P., Hill, S. S., Stone, S. I., and Bunge, S. A. (2011). Dissociable effects of reasoning and speed training in children. Dev. Sci. 14, 582–590.
Macoveanu, J., Klingberg, T., and Tegner, J. (2006). A biophysical model of multiple-item working memory: a computational and neuroimaging study. Neuroscience 141, 1611–1618.
Maguire, E. A., Gadian, D. G., Johnsrude, I. S., Good, C. D., Ashburner, J., Frackowiak, R. S., and Frith, C. D. (2000). Navigation-related structural change in the hippocampi of taxi drivers. Proc. Natl. Acad. Sci. U.S.A. 97, 4398–4403.
Maguire, E. A., Woollett, K., and Spiers, H. J. (2006). London taxi drivers and bus drivers: a structural MRI and neuropsychological analysis. Hippocampus 16, 1091–1101.
Mangels, J. A., Butterfield, B., Lamb, J., Good, C., and Dweck, C. S. (2006). Why do beliefs about intelligence influence learning success? A social cognitive neuroscience model. Soc. Cogn. Affect. Neurosci. 1, 75–86.
McNab, F., Varrone, A., Farde, L., Jucaite, A., Bystritsky, P., Forssberg, H., and Klingberg, T. (2009). Changes in cortical dopamine D1 receptor binding associated with cognitive training. Science 323, 800–802.
Mezzacappa, E., and Buckner, J. C. (2010). Working memory training for children with attention problems or hyperactivity: a school-based pilot study. Sch. Ment. Health 2, 202–208.
Miotto, E. C., Savage, C. R., Evans, J. J., Wilson, B. A., Martins, M. G., Iaki, S., and Amaro, E. Jr. (2006). Bilateral activation of the prefrontal cortex after strategic semantic cognitive training. Hum. Brain Mapp. 27, 288–295.
Miyake, A., Friedman, N. P., Emerson, M. J., Witzki, A. H., Howerter, A., and Wager, T. D. (2000). The unity and diversity of executive functions and their contributions to complex “Frontal Lobe” tasks: a latent variable analysis. Cogn. Psychol. 41, 49–100.
Morrison, A. B., and Chein, J. M. (2010). Does working memory training work? The promise and challenges of enhancing cognition by training working memory. Psychon. Bull. Rev. 18, 46–60.
Munakata, Y., Casey, B. J., and Diamond, A. (2004). Developmental cognitive neuroscience: progress and potential. Trends Cogn. Sci. 8, 122–128.
Noack, H., Lövdén, M., Schmiedek, F., and Lindenberger, U. (2009). Cognitive plasticity in adulthood and old age: gauging the generality of cognitive intervention effects. Restor. Neurol. Neurosci. 27, 435–453.
Nyberg, L., Dahlin, E., Stigsdotter, N. A., and Backman, L. (2009). Neural correlates of variable working memory load across adult age and skill: dissociative patterns within the fronto-parietal network. Scand. J. Psychol. 50, 41–46.
Nyberg, L., Sandblom, J., Jones, S., Neely, A. S., Petersson, K. M., Ingvar, M., and Backman, L. (2003). Neural correlates of training-related memory improvement in adulthood and aging. Proc. Natl. Acad. Sci. U.S.A. 100, 13728–13733.
Olesen, P. J., Westerberg, H., and Klingberg, T. (2004). Increased prefrontal and parietal activity after training of working memory. Nat. Neurosci. 7, 75–79.
Owen, A. M., Mcmillan, K. M., Laird, A. R., and Bullmore, E. (2005). N-back working memory paradigm: a meta-analysis of normative functional neuroimaging studies. Hum. Brain. Mapp. 25, 46–59.
Paus, T. (2010). Growth of white matter in the adolescent brain: myelin or axon? Brain Cogn. 72, 26–35.
Poldrack, R. A. (2000). Imaging brain plasticity: conceptual and methodological issues–a theoretical review. Neuroimage 12, 1–13.
Poldrack, R. A. (2010). Interpreting developmental changes in neuroimaging signals. Hum. Brain Mapp. 31, 872–878.
Posner, M. I., and Rothbart, M. K. (2005). Influencing brain networks: implications for education. Trends Cogn. Sci. 9, 99–103.
Qin, Y., Carter, C. S., Silk, E. M., Stenger, V. A., Fissell, K., Goode, A., and Anderson, J. R. (2004). The change of the brain activation patterns as children learn algebra equation solving. Proc. Natl. Acad. Sci. U.S.A. 101, 5686–5691.
Qin, Y., Sohn, M. H., Anderson, J. R., Stenger, V. A., Fissell, K., Goode, A., and Carter, C. S. (2003). Predicting the practice effects on the blood oxygenation level-dependent (BOLD) function of fMRI in a symbolic manipulation task. Proc. Natl. Acad. Sci. U.S.A. 100, 4951–4956.
Rabiner, D. L., Murray, D. W., Skinner, A. T., and Malone, P. S. (2010). A randomized trial of two promising computer-based interventions for students with attention difficulties. J. Abnorm. Child Psychol. 38, 131–142.
Raichle, M. E., Macleod, A. M., Snyder, A. Z., Powers, W. J., Gusnard, D. A., and Shulman, G. L. (2001). A default mode of brain function. Proc. Natl. Acad. Sci. U.S.A. 98, 676–682.
Ramscar, M., and Gitcho, N. (2007). Developmental change and the nature of learning in childhood. Trends Cogn. Sci. 11, 274–279.
Rueda, M. R., Rothbart, M. K., Mccandliss, B. D., Saccomanno, L., and Posner, M. I. (2005). Training, maturation, and genetic influences on the development of executive attention. Proc. Natl. Acad. Sci. U.S.A. 102, 14931–14936.
Sayala, S., Sala, J. B., and Courtney, S. M. (2006). Increased neural efficiency with repeated performance of a working memory task is information-type dependent. Cereb. Cortex 16, 609–617.
Schmiedek, F., Lövdén, M., and Lindenberger, U. (2010). Hundred days of cognitive training enhance broad cognitive abilities in adulthood: findings from the COGITO study. Front. Aging Neurosci. 2:27. doi: 10.3389/fnagi.2010.00027
Schneiders, J. A., Opitz, B., Krick, C. M., and Mecklinger, A. (2011). Separating intra-modal and across-modal training effects in visual working memory: an fMRI investigation. Cereb. Cortex 21, 2555–2564.
Scholz, J., Klein, M. C., Behrens, T. E., and Johansen-Berg, H. (2009). Training induces changes in white-matter architecture. Nat. Neurosci. 12, 1370–1371.
Shalev, L., Tsal, Y., and Mevorach, C. (2007). Computerized progressive attentional training (CPAT) program: effective direct intervention for children with ADHD. Child Neuropsychol. 13, 382–388.
Shaw, P., Greenstein, D., Lerch, J., Clasen, L., Lenroot, R., Gogtay, N., Evans, A., Rapoport, J., and Giedd, J. (2006). Intellectual ability and cortical development in children and adolescents. Nature 440, 676–679.
Shaywitz, B. A., Shaywitz, S. E., Blachman, B. A., Pugh, K. R., Fulbright, R. K., Skudlarski, P., Mencl, W. E., Constable, R. T., Holahan, J. M., Marchione, K. E., Fletcher, J. M., Lyon, G. R., and Gore, J. C. (2004). Development of left occipitotemporal systems for skilled reading in children after a phonologically- based intervention. Biol. Psychiatry 55, 926–933.
Simos, P. G., Fletcher, J. M., Bergman, E., Breier, J. I., Foorman, B. R., Castillo, E. M., Davis, R. N., Fitzgerald, M., and Papanicolaou, A. C. (2002). Dyslexia-specific brain activation profile becomes normal following successful remedial training. Neurology 58, 1203–1213.
Sowell, E. R., Peterson, B. S., Thompson, P. M., Welcome, S. E., Henkenius, A. L., and Toga, A. W. (2003). Mapping cortical change across the human life span. Nat. Neurosci. 6, 309–315.
Sowell, E. R., Thompson, P. M., Tessner, K. D., and Toga, A. W. (2001). Mapping continued brain growth and gray matter density reduction in dorsal frontal cortex: inverse relationships during postadolescent brain maturation. J. Neurosci. 21, 8819–8829.
St. Clair-Thompson, H., Stevens, R., Hunt, A., and Bolder, E. (2010). Improving children's working memory and classroom performance. Educ. Psychol. 30, 203–219.
Stevens, C., Fanning, J., Coch, D., Sanders, L., and Neville, H. (2008). Neural mechanisms of selective auditory attention are enhanced by computerized training: electrophysiological evidence from language-impaired and typically developing children. Brain Res. 1205, 55–69.
Stiles, J. (2008). The Fundamentals of Brain Development; Integrating Nature and Nurture. Cambridge, MA: Harvard University Press.
Swanson, H. L., Kehler, P., and Jerman, O. (2010). Working memory, strategy knowledge, and strategy instruction in children with reading disabilities. J. learn. Disabil. 43, 24.
Takeuchi, H., Sekiguchi, A., Taki, Y., Yokoyama, S., Yomogida, Y., Komuro, N., Yamanouchi, T., Suzuki, S., and Kawashima, R. (2010). Training of working memory impacts structural connectivity. J. Neurosci. 30, 3297–3303.
Takeuchi, H., Taki, Y., Sassa, Y., Hashizume, H., Sekiguchi, A., Fukushima, A., and Kawashima, R. (2011). Working memory training using mental calculation impacts regional gray matter of the frontal and parietal regions. PLoS One 6:e23175. doi: 10.1371/journal.pone.0023175
Temple, E., Deutsch, G. K., Poldrack, R. A., Miller, S. L., Tallal, P., Merzenich, M. M., and Gabrieli, J. D. (2003). Neural deficits in children with dyslexia ameliorated by behavioral remediation: evidence from functional MRI. Proc. Natl. Acad. Sci. U.S.A. 100, 2860–2865.
Thomas, M., and Karmiloff-Smith, A. (2002). Are developmental disorders like cases of adult brain damage? Implications from connectionist modelling. Behav. Brain Sci. 25, 727–750; discussion 750–787.
Tomasi, D., Ernst, T., Caparelli, E. C., and Chang, L. (2004). Practice-induced changes of brain function during visual attention: a parametric fMRI study at 4 Tesla. Neuroimage 23, 1414–1421.
Thompson-Schill, S. L., Ramscar, M., and Chrysikou, E. G. (2009). Cognition without control: when a little frontal lobe goes a long way. Curr. Dir. Psychol. Sci. 18, 259–263.
Thorell, L. B., Lindqvist, S., Bergman Nutley, S., Bohlin, G., and Klingberg, T. (2009). Training and transfer effects of executive functions in preschool children. Dev. Sci. 12, 106–113.
Uylings, H. B. M. (2006). Development of the human cortex and the concept of “critical” or “sensitive” periods. Lang. Learn. 56, 59–90.
Van der Molen, M. J., van Luit, J. E., Van der Molen, M. W., Klugkist, I., and Jongmans, M. J. (2010). Effectiveness of a computerised working memory training in adolescents with mild to borderline intellectual disabilities. J. Intellect. Disabil. Res. 54, 433–447.
van't Hooft, I., Andersson, K., Bergman, B., Sejersen, T., von Wendt, L., and Bartfai, A. (2005). Beneficial effect from a cognitive training programme on children with acquired brain injuries demonstrated in a controlled study. Brain Inj. 19, 511–518.
van't Hooft, I., Andersson, K., Sejersen, T., Bartfai, A., and von Wendt, L. (2003). Attention and memory training in children with acquired brain injuries. Acta Paediatr. 92, 935–940.
Wager, T. D., and Smith, E. E. (2003). Neuroimaging studies of working memory: a meta-analysis. Cogn. Affect. Behav. Neurosci. 3, 255–274.
Willis, S. L., and Schaie, K. W. (2009). Cognitive training and plasticity: theoretical perspective and methodological consequences. Restor. Neurol Neurosci. 27, 375–389.
Keywords: training, development, executive functions, cognitive control, plasticity, neuroimaging, brain maturation
Citation: Jolles DD and Crone EA (2012) Training the developing brain: a neurocognitive perspective. Front. Hum. Neurosci. 6:76. doi: 10.3389/fnhum.2012.00076
Received: 13 December 2011; Paper pending published: 06 January 2012;
Accepted: 19 March 2012; Published online: 09 April 2012.
Edited by:
Julia Karbach, Saarland University, GermanyReviewed by:
Torkel Klingberg, Karolinska Institutet, SwedenMartin Buschkuehl, The University of Michigan, USA
Copyright: © 2012 Jolles and Crone. This is an open-access article distributed under the terms of the Creative Commons Attribution Non Commercial License, which permits non-commercial use, distribution, and reproduction in other forums, provided the original authors and source are credited.
*Correspondence: Dietsje D. Jolles, Department of Psychiatry and Behavioral Sciences, Stanford University School of Medicine, Stanford Cognitive and Systems Neuroscience Laboratory, 1070 Arastradero Rd. Suite 220, Palo Alto, CA 94304, USA. e-mail: ddjolles@stanford.edu