Older Women, Deeper Learning: Age and Gender Interact to Predict Learning Approach and Academic Achievement at University
- 1School of Psychology, The University of Newcastle, Newcastle, NSW, Australia
- 2School of Psychology and Exercise Science, Murdoch University, Singapore, Singapore
- 3School of Education, The University of Newcastle, Newcastle, NSW, Australia
- 4Institute of Educational Technology, The Open University, Milton Keynes, United Kingdom
Older students have reported a series of barriers to starting their higher education experience. However, quantitative evidence suggests that older students, particularly older women, have unique approaches to learning that enhance their satisfaction with their higher education studies. The current study sought to extend these findings by quantitatively examining an interaction between age and gender in predicting approaches to learning and subsequent academic achievement. The research provided an original analysis from two previous studies. Participants consisted of Australian and United Kingdom undergraduates. The Australian samples were 367 undergraduates at an Australian public regional university. The United Kingdom samples were 2,163 undergraduates enrolled with a distance higher education provider. Participants completed a research survey either online or on paper. Consistent with previous research, age moderated the effect of gender on deep learning, such that gender predicted deep learning more strongly among older students than younger students in both samples. Furthermore, gender predicted achievement in both samples, such that women out-performed men. Finally, deep learning only explained the relationship between gender and academic achievement when students were older. Based on this evidence, higher education institutions should consider and address the barriers that adult students, particularly older women, experience in order to enhance the social mobility benefits from a university degree that this non-traditional higher education group accrues.
Introduction
Educational researchers have identified different approaches to learning (Marton and Säljö, 1976; Richardson, 2005). Deep learning approaches represent students’ attempts to understand the meaning of course materials, and are marked by engagement with the content, the relation of what is learned to previous knowledge and everyday experience, and attempts to understand the logic of arguments (Entwistle, 1987). Surface approaches indicate the use of memorization to retain course materials for assessment, without reflecting on the purpose of the tasks or the principles that can be derived from teaching examples (Entwistle, 1987). These different learning approaches have different implications for academic achievement (Robbins et al., 2004). The impact of age and gender on the employment of these learning approaches has been established (Richardson, 2013), but limited attention has been paid to how age and gender interact to predict learning approach. Rubin et al. (2018) found that older women demonstrated deeper learning than other demographic groups at university, and that this deeper learning had a positive impact on their degree satisfaction. These findings accord with a qualitative literature that identifies older students as having a clear understanding of the personal meaning and relevance of their studies (McCune et al., 2010). However, qualitative studies also indicate that older students hesitate to attend higher education due to a series of outside commitments (Fragoso et al., 2013), and they feel stigmatised when they do participate (Mallman and Lee, 2016, 2017). Hence, higher education institutions should pay closer attention to the barriers that older students, particularly older women, experience in their higher education transition (Andreou et al., 2006).
The current study presents an original analysis of two previous studies on the learning approach of tertiary education students (Richardson, 2005; Rubin et al., 2018). Rubin et al. (2018) examined the interaction between age and gender in predicting learning approaches among on-campus university students at an Australian public university. The present analyses represent a subset of participants from the sample reported in Rubin et al. (2018), who gave permission for their academic records to be accessed. We explicitly review the findings of Rubin et al. (2018) in this literature review as it is directly relevant to the interactive effect of age and gender on approach to learning. Richardson (2005) reported the relationships between approaches to learning and their perceptions of their academic contexts in students enrolled via distance learning at the Open University in the United Kingdom. Because the findings of Richardson (2005) did not report on any demographic effects associated with learning approach, or examine academic achievement, we do not address it in further detail in our literature review.
This research considers the interaction of age and gender on learning approaches and tertiary education achievement. Academic achievement data was not reported in Richardson (2005), and data on academic achievement was not available at the time Rubin et al. (2018) conducted their original analyses. We tested the hypothesis that age and gender interact to predict deep learning, such that older women are most likely to employ deep learning strategies. We also explored the possibility that deep learning explains (mediates) the association between gender and university achievement only among older students. Our conceptual model is a moderated mediation model, and it can be found in Figure 1, along with the corresponding statistical model that forms the foundation of our analyses. The following literature review explores each of the links in our model in further detail.
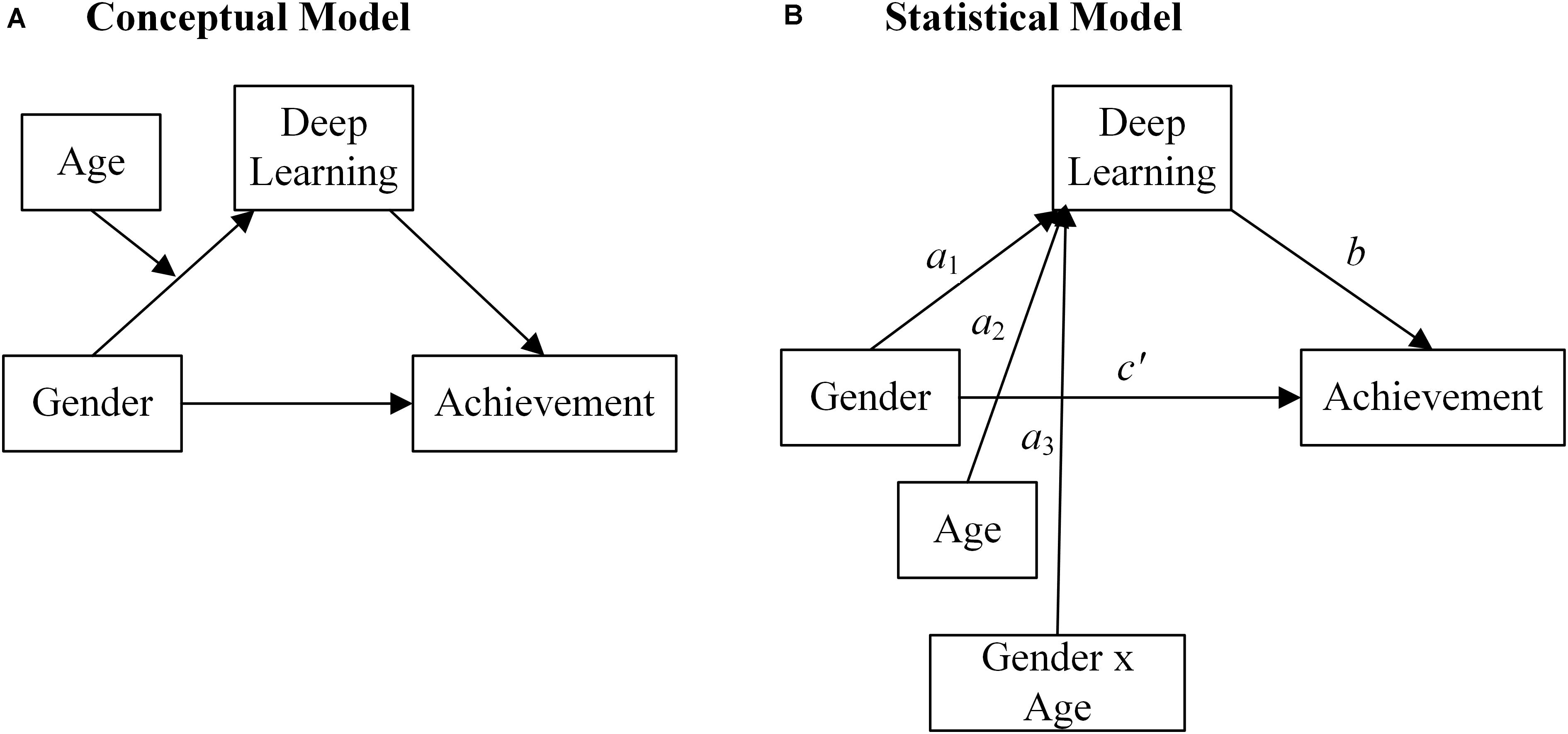
Figure 1. Hypothesized first-stage moderated mediation model including; (A) the conceptual model; and (B) the statistical model specifically tested. a_1 = effect of gender on deep learning; a_2 = effect of age on deep learning; a_3 = effect of the interaction between age and gender on deep learning; b = effect of deep learning on achievement; c′ = direct effect of gender on achievement (with gender, age, and deep learning controlled).
Women outperform men in academic achievement at all levels of schooling and in all subjects (Voyer and Voyer, 2014). These differences in achievement are difficult to account for because the differences between men and women on cognitive tasks are negligible (Caplan et al., 1997). Consistent with previous research findings, we hypothesised that women exhibit higher academic achievement than men (pathway c′ in Figure 1).
The evidence that approaches to learning differ by gender is mixed. Some studies have found that men report a greater surface learning approach than women (Salamonson et al., 2013; Rubin et al., 2018), and others have found that women report a greater surface learning approach (Paver and Gammie, 2005). Other studies have identified that women report a deeper learning approach than men (Tarabashkina and Lietz, 2011). Finally, many studies conclude that there are no identifiable gender differences in learning approach (Zeegers, 2001; Andreou et al., 2006; Heikkilä and Lonka, 2006; May et al., 2012).
This lack of agreement on the precise association between learning approach and gender might be due to the small effect sizes evident in gender differences in learning approach. These small effect sizes will be difficult to detect in studies that have small samples (Zeegers, 2001; Paver and Gammie, 2005; Andreou et al., 2006; Heikkilä and Lonka, 2006; Tarabashkina and Lietz, 2011; May et al., 2012). Applying Cohen’s (1988) benchmarks for an appropriate sample size to detect a small relationship at a significance level of 0.05, we identified three studies that had a sufficient sample size to appropriately test gender differences in learning approach (Richardson, 2013; Salamonson et al., 2013; Rubin et al., 2018). Both Salamonson et al. (2013) and Rubin et al. (2018) found that men reported a greater surface learning approach than women. Further, Rubin et al. (2018) found no significant main effect of gender on deep learning. The third study identified no gender effects for either surface or deep learning (Richardson, 2013). Therefore, the question of precisely whether and how gender influences learning approaches is still open for debate (pathway a_1 in Figure 1).
Older students report fewer surface and more deep learning approaches than younger students (Richardson, 1995, 2013; Justice and Dornan, 2001; Fragoso et al., 2013; Salamonson et al., 2013; Rubin et al., 2018). Older students might be more motivated by intrinsic goals such as improving their knowledge, rather than by extrinsic goals related to their career progression (Richardson, 2013).
Researchers have often categorised students into older, non-school leaver groups and younger, school-leaver populations, generally based on a threshold age that ranges from 21 to 30 years and above (Kasworm, 2018). Defining students using a specific age category might not capture the heterogeneity of life situations experienced by students at all life stages (Kasworm, 2018). Our aim in this study was to understand how age influenced learning approach in conjunction with gender (pathway a_2 in Figure 1). Rather than define students as either older or younger than a specific threshold, we choose to refer to younger versus older students in relative terms (Rubin et al., 2018). We achieved this by using age as a continuous variable. This approach substantially increased the statistical power of our analyses (Royston et al., 2006).
Three previous studies have investigated the interactive effect of age and gender on approaches to learning. Andreou et al. (2006) sampled 452 students from a university in Greece and measured approaches to learning using Entwistle and Tait’s (1995) revised approaches to studying inventory (RASI). They found that older students of both genders reported a deeper learning approach than younger students. This effect varied depending on the degree program in which students were enrolled. Sadler-Smith and Tsang (1998) examined the cross-cultural validity of the RASI in Hong Kong (N = 183) and the United Kingdom (N = 225) among second-year business undergraduates. Age and gender interacted to predict learning approach in the Hong Kong sample only. Contrary to Andreou et al. (2006), older male students in Hong Kong reported more deep learning than younger male students. Older female students reported less deep learning than younger female students. Both of these studies had limited power to detect these interaction effects, which could account for the different findings (Blake and Gangestad, 2020). To overcome these power issues, Rubin et al. (2018) sampled N = 983 undergraduate students at an Australian university. Their study found that age and gender interacted to predict deep learning approach, such that older women reported a deeper learning approach than younger women and all men (pathway a_3 in Figure 1). This study had a large sample size, thus overcoming the power issues in previous research. However, given concerns about replicability in science (Zwaan et al., 2018), there is a need to replicate this finding in a similarly large sample in order to show that it is not specific to the particular university sample used by Rubin et al. (2018).
The present studies extended previous research by exploring whether age and gender differences in learning approach had an influence on academic achievement. This part of the research was somewhat exploratory in nature. However, as indicated below, the hypotheses that were tested were established in prior literature.
Deep learning is preferable to surface learning, because it reflects a commitment to understand the meaning of material and to integrate new ideas with previous knowledge (Salamonson et al., 2013). Deep learning predicts better academic achievement (Zeegers, 2001; Paver and Gammie, 2005; Heikkilä and Lonka, 2006; Tarabashkina and Lietz, 2011; May et al., 2012; Salamonson et al., 2013). A surface learning approach sometimes predicts worse academic achievement (Zeegers, 2001; Tarabashkina and Lietz, 2011; Salamonson et al., 2013) and sometimes does not predict achievement at all (Paver and Gammie, 2005; Heikkilä and Lonka, 2006; Richardson, 2007). We expected that deep learning would be positively associated with academic achievement, and we investigated the possibility that surface learning was associated with negative academic achievement (pathway b in Figure 1).
If there are demographic differences in learning approach, then we might also expect learning approaches to explain differences in academic achievement for particular demographic groups. If older women show deeper learning, then we might also expect deep learning to explain (mediate) achievement for this group.
The present study sought to examine the conditions under which learning approaches explain academic achievement. The interaction between age and gender can influence approaches to learning, such that older women are deeper learners than both younger women and all men (Rubin et al., 2018). Given the established association between achievement and learning approaches (Salamonson et al., 2013) an interaction between age and gender might explain academic achievement for these demographic groups through learning approach.
Further, it is widely acknowledged that approaches to learning change depending on the context (Richardson, 2007, 2013), particularly the mode of study (e.g., traditional on-campus attendance), the type of degree program, and the student’s country. We examined the literature and determined that with few exceptions (i.e., Harper and Kember, 1986; Duff, 2003), the research on distance education students was solely conducted by Richardson and colleagues (Richardson et al., 1999; Richardson and Price, 2003; Richardson, 2005, 2006, 2007, 2013). All other examinations of our key effects were conducted on students studying in on-campus modes. In one study, we were unable to identify where students were studying (Smith and Miller, 2005). In many studies, the classroom mode of students was not explicitly stated, thus we drew conclusions about the location of students from the description of the study procedures (Gow and Kember, 1990; Wilson et al., 1996; Justice and Dornan, 2001; Paver and Gammie, 2005; Heikkilä and Lonka, 2006; Heijne-Penninga et al., 2008, 2010; Tarabashkina and Lietz, 2011; May et al., 2012). Further, in an attempt to explicitly compare the literature on approaches to learning in campus-based and distance education students, Richardson (2000) has suggested that the age of students, rather than the learning context, might better account for differences in learning approach. Given the broad assumption that the learning approaches of students in higher education are context dependent, an explicit comparison of the learning approaches of on-campus versus distance education students is both warranted and timely. We therefore sought to compare the findings from an Australian on-campus sample who completed the Study Process Questionnaire (Rubin et al., 2018) with students studying via distance education in the United Kingdom using the RASI (Richardson, 2005). This represents a conceptual replication study, because we are explicitly testing whether age and gender influence a deep learning approach and subsequent achievement in a similar way across different learning contexts, with two alternative measures of learning approaches (Zwaan et al., 2018).
We expected that older students would report deeper learning and less surface learning. Given that learning approach is associated with motivation, and women generally have more intrinsic motivation than men (Bonneville-Roussy et al., 2017), we further predicted that female students would report less surface learning and more deep learning than male students in both the Australian and United Kingdom samples. In addition, we predicted an interactive effect of age and gender in both samples, such that gender differences in learning approach would be larger among older students than among younger students.
The current study further sought to establish whether deep learning explained gender differences in university achievement. Consistent with Voyer and Voyer (2014), we predicted that women in both samples would have higher university achievement scores than men (pathway c′ in Figure 1). We further predicted, based on Robbins et al. (2004), that deeper learning would be associated with higher academic achievement (pathway b in Figure 1). Finally, because older women were more likely to employ deep learning approaches than other students at university, we expected that their deep learning approach might explain their achievement at university.
The analyses reported in this study represent an original re-analysis of two previously published papers (Richardson, 2005; Rubin et al., 2018). The data reported in Richardson (2005) was not collected to test the specific model tested in the current manuscript. In addition, the academic achievement data that was analyzed in this study was not available for analysis in Rubin et al. (2018).
Study 1: Australian On-Campus Students
Method
Participants
Students were 361 undergraduates from a previous study who gave permission for their academic records to be accessed (Rubin et al., 2018). Students in Australia usually study for 3 years to obtain an undergraduate degree, with selected students studying for 4 years to achieve an honours degree. Students came from all 4 years of undergraduate study (first year N = 192 [53.2%]; second year N = 97 [26.9%]; third year N = 47 [13.0%]; fourth year N = 24 [6.6%]). Participants were from four broad degree types: allied health (N = 139, 38.5%), science (N = 97, 26.9%), business (N = 76, 21.1%), and engineering (N = 30, 8.3%). Participants were 207 women (57.3%) and 154 men (42.7%). The age of the sample ranged between 18 and 66 years, with a mean age of 23.15 (SD = 7.29). Women were more likely to be enrolled in allied health (74.1%) programs, while men were more likely to be enrolled in engineering (93.3%), χ2 (3) = 56.20, p < 0.001. Students enrolled in allied health were older (M = 24.58, SD = 8.40) than those in business (M = 22.04, SD = 6.29, p = 0.016) and engineering (M = 21.30, SD = 2.32, p = 0.028). Consequently, degree type was controlled in our subsequent models.
Procedure and Measures
Students volunteered to complete a 15-min online or paper survey in response to advertisements in online course systems, emails, and visits by researchers to lectures and tutorials. All data were collected in October and November of 2013 (Rubin et al., 2018). This research was approved by the institutional Human Research Ethics Committee.
Deep and surface approaches to learning were measured using the 20-item, Revised Two-Factor Study Process Questionnaire (Biggs et al., 2001). An example item from the deep approach scale is “I work hard at my studies because I find the material interesting.” In contrast, an example item from the surface approach scale was “I see no point in learning material which is not likely to be in the examination.” Both subscale scores had good internal reliability in the current sample (α = 0.81 for deep learning; α = 0.74 for surface learning).
Academic achievement was derived from students’ academic transcripts. A cumulative weighted average mark (WAM) was computed from the courses present on student transcripts at the time of coding, accounting for the number of credit points associated with each course. University policy at this institution was to record course marks of 44 and below as 44, and so the scores ranged between 44 and 100, with 50 the mark required to pass. The course marks were averaged to form an overall cumulative achievement score for each student ranging between 44 and 100. Despite the cut-off at 44 marks, this variable was normally distributed with no evidence of floor effects.
Data Analysis
Missing data represented less than 6% of the total dataset. Mean substitution was used to deal with this missing data, which is appropriate when the missing data represents less than 20% within each scale (Downey and King, 1998). If participants responded to 75% or more of the items included within each scale, then their missing data was replaced with the sample mean for each item. If they did not respond to more than 25% of the items within a scale, then all their data for that scale was removed. Age was substantially positively skewed (skew = 3.01). To produce a more normal distribution, we transformed this variable by computing its inverse function (skewness = −1.20). Because of the inverse transformation, decreasing values for this variable corresponded to increasing age. We coded gender as 1 for men and 2 for women in both samples. All variables were mean-centred (z-score transformed) prior to analyses.
We used Hayes (2013) PROCESS macro for SPSS to test the moderating effect of age on the association between gender and the dependent variables of learning approach, satisfaction, and academic achievement. We also calculated moderated mediation models on university achievement. Here, gender was the predictor and age the moderator of the mediated effect through deep learning.
Results
Table 1 reports descriptive statistics and correlations between age, surface learning, deep learning, and achievement. Age was positively associated with deep learning and negatively associated with surface learning. Deep learning was positively associated with academic achievement, and surface learning was negatively associated with achievement.
The Effects of Age and Gender on Learning Approach
Moderator analyses can be found in Table 2. Older students reported less of a surface learning approach than younger students, with female students reporting less surface learning than male students. There was no significant interaction between age and gender for surface learning. Only gender was a significant and positive predictor of academic achievement, such that women consistently outperformed men.
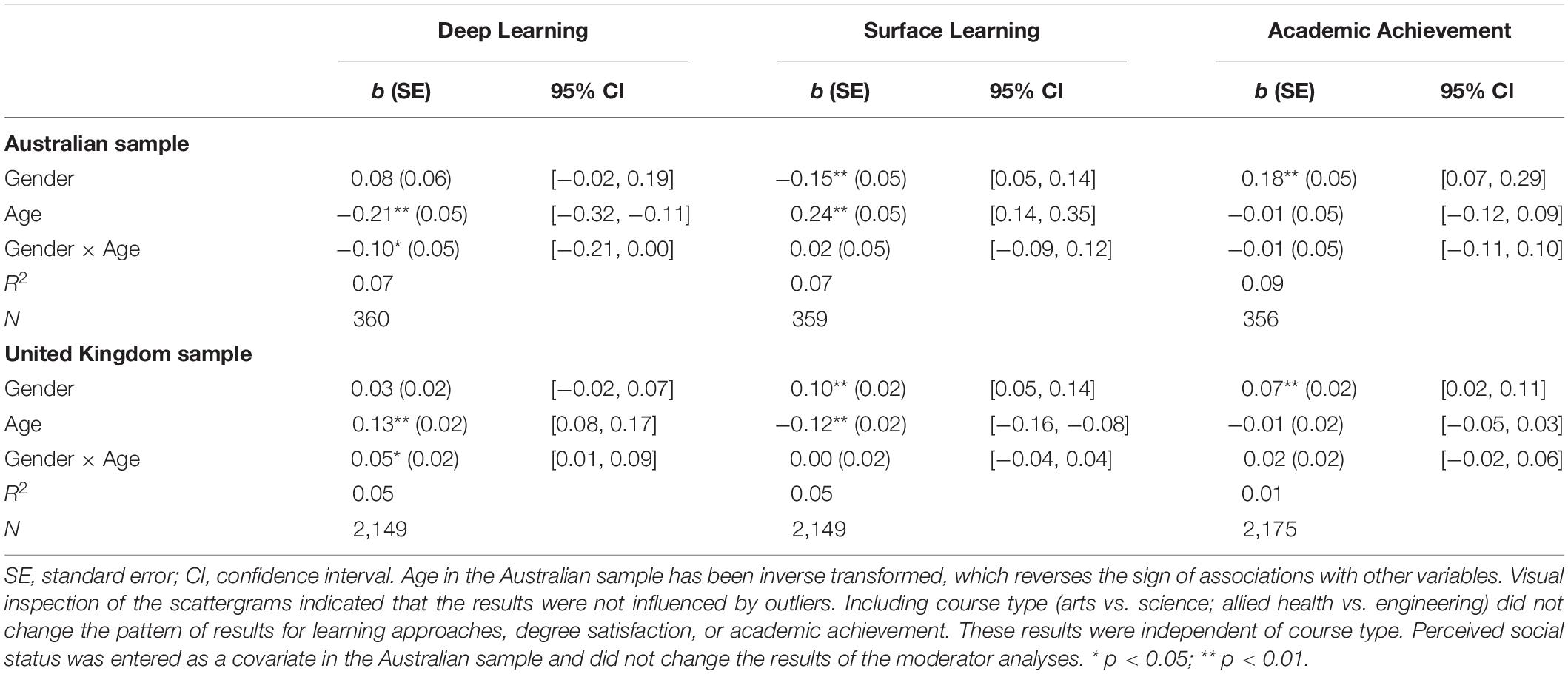
Table 2. Gender, age, and their interaction as predictors of learning approach, degree satisfaction, and academic achievement.
Gender was not significantly associated with deep learning. However, age was a significant and positive predictor of deep learning. This main effect was qualified by a significant interaction. We thus compared the effects of age on deep learning among men and women separately in Figure 2A. Older women were deeper learners than younger women, b = −0.30, SE = 0.07, t (203) = −4.19, p < 0.001. Among men, the relationship between age and deep learning was much weaker and non-significant, b = −0.10, SE = 0.08, t (150) = −1.30, p = 0.194.
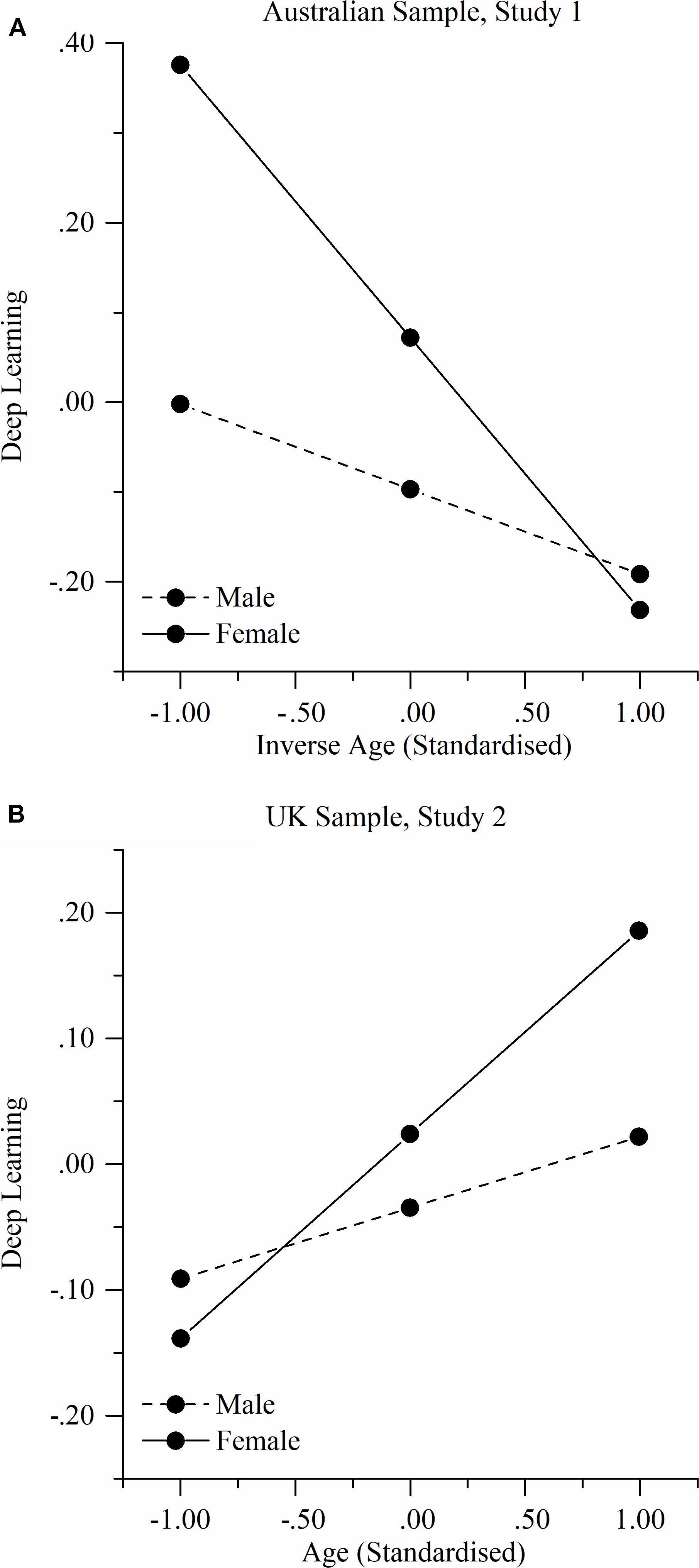
Figure 2. The interactive effect of age and gender on deep learning. (A) Displays the results for the Australian sample, and (B) illustrates the results for the United Kingdom sample.
Regions of Significance
Using the Johnson-Neyman technique (Hayes, 2013), we probed the interaction at different values of the moderator to determine at what point of age the effect of gender on deep learning became significant. Figure 3A shows the Johnson-Neyman plot for the Australian sample. The figure shows that the effect of gender on deep learning was positive and significant at z-score ages above −0.27, with 75.56% of the sample falling above this value. This value corresponded to a real age of 23.05 years. Above the age of 23.05, gender had a significant and positive effect on deep learning, indicating that women reported more deep learning approaches than men. Below this age, the effect of gender on deep learning was non-significant.
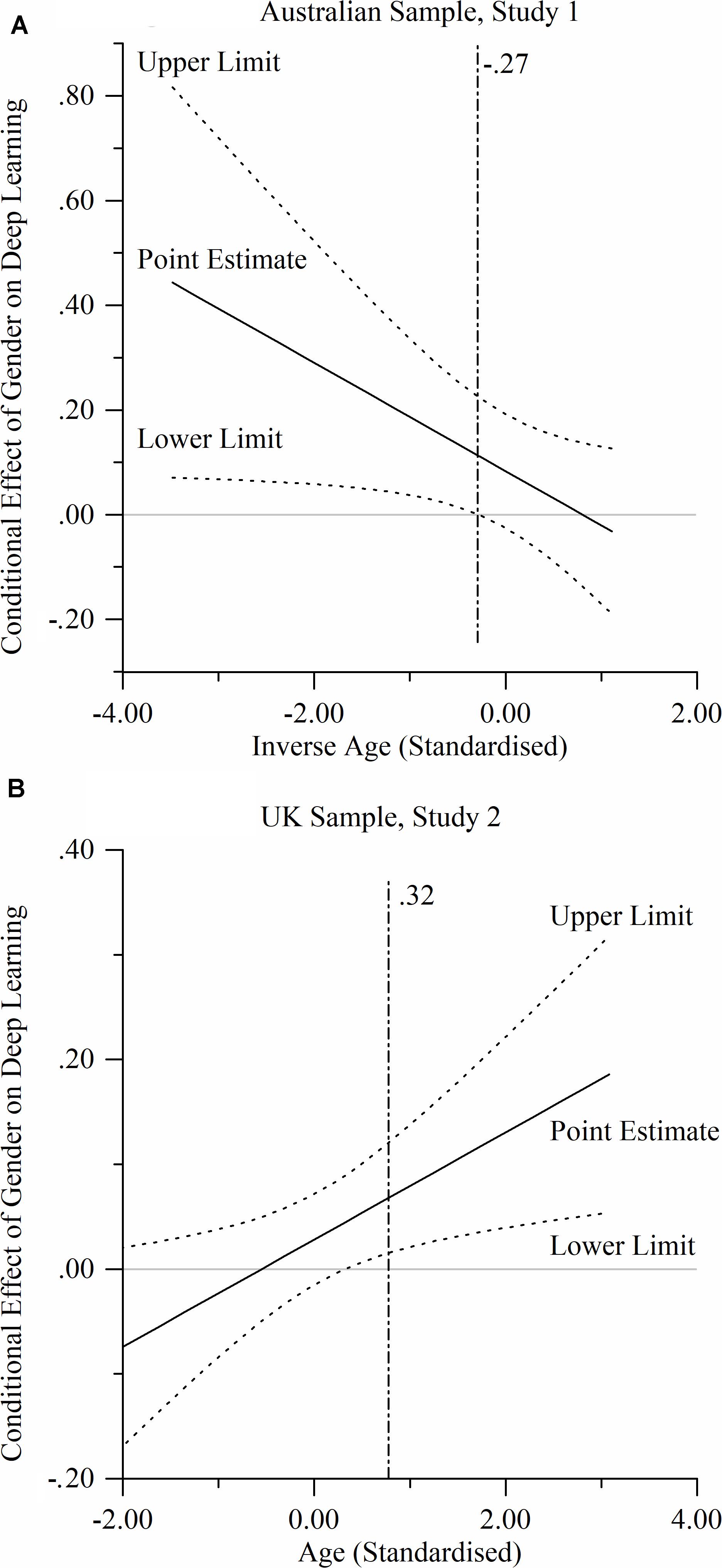
Figure 3. Johnson-Neyman plot displaying the point at which gender had a significant effect on deep learning relative to age. (A) Shows the results for the Australian sample, and (B) demonstrates the results for the United Kingdom sample. Upper and lower limits refer to 95% confidence interval (CI) bounds at values of the moderator (age).
Conditional Process Analysis
We tested a conditional process model (Model 7; Hayes, 2013) that examined the impact of gender (the predictor) on achievement (the outcome), with age as a moderator of the pathway from gender to deep learning (the mediator). The analysis is reported in Table 3. Examination of the bootstrapped 95% confidence intervals (CIs) indicated that deep learning mediated the relationship between gender and academic achievement at older ages only, as indicated by 95% CIs that did not cross zero. The index of moderated mediation was significant in the Australian sample, a3b1 = −0.02, SE = 0.01, 95% CI (−0.0466, −0.0023). Figure 4A contains the results of this analysis for the Australian sample.
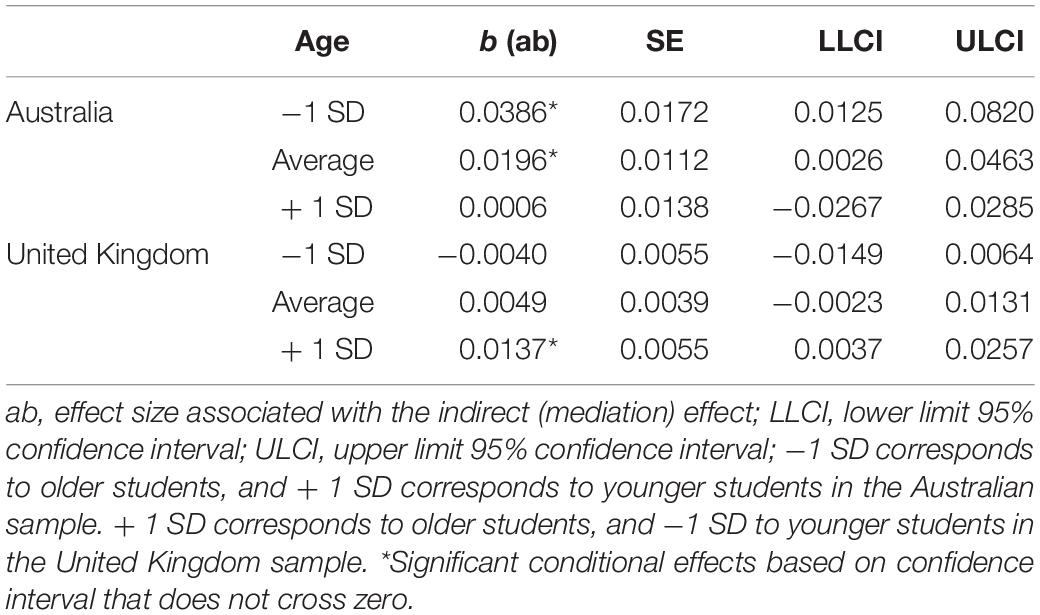
Table 3. The conditional indirect effect of gender on achievement through deep learning at values of age.
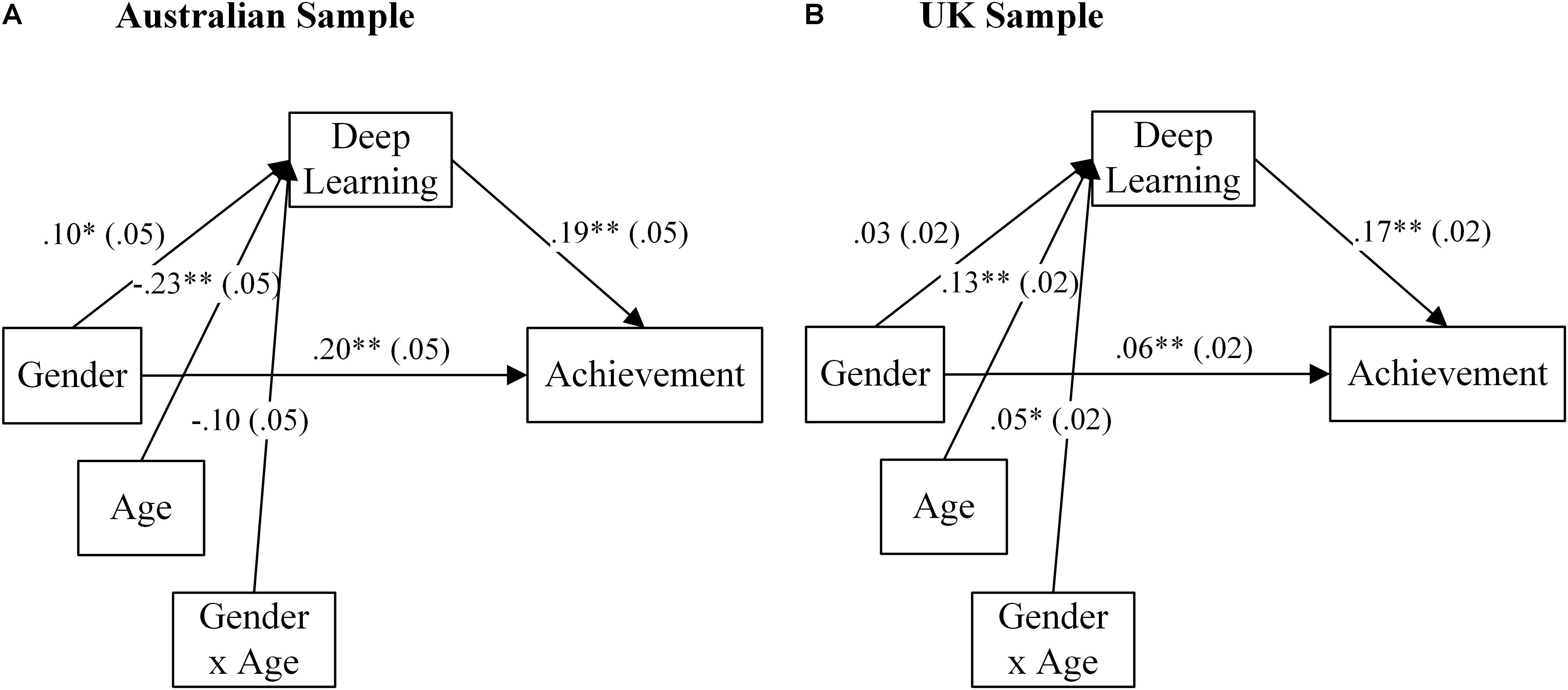
Figure 4. Conditional process models for (A) Australia and (B) the United Kingdom, including standardized regression weights for each pathway (standard errors). * p < 0.05; ** p < 0.001.
Study 2: United Kingdom Distance Education Students
Method
Participants
The United Kingdom sample consisted of 2,176 undergraduates who were studying their university courses by distance education at one institution in the United Kingdom (Richardson, 2005). Participants were selected from three broad course types: arts (N = 1,482, 68.1%), science (N = 414, 19.0%), and mixed cross-faculty courses (N = 281, 12.9%). Based on the total student enrolments in the selected courses (N = 3,953), the response rate for the United Kingdom sample was 60.6%. At the time this data was collected, students were enrolled in individual course units and were only awarded their degrees when they had accumulated a sufficient number of credit points. This contrasts with the Australian sample, in which students were recruited from degree programs rather than the specific course units within degree programs.
The sample contained 1,400 women (64.3%) and 776 men (35.6%). Participants ranged in age from 21 to 87 years, with a mean age of 48.02 (SD = 12.65). One student did not report their age or gender. Women were more likely to be enrolled in arts (66.8%) and cross-faculty course types (86.1%), and men were more likely to be enrolled in science courses (59.3%), χ2 (2) = 162.80, p < 0.001. Men had a mean age of 49.10 (SD = 13.14), and women had a mean age of 47.42 (SD = 12.33). There was a significant gender difference in age, t (2,174) = 2.97, p = 0.003. Older students were more likely to be enrolled in the arts courses (M = 50.09, SD = 12.82) as opposed to science and mixed cross-faculty courses (ps < 0.001). There was no difference in age between students enrolled in science (M = 44.28, SD = 11.61) and cross-faculty (M = 42.60, SD = 10.13) course types. Because age and gender were associated with course type, we controlled for course type in all subsequent models.
Students were enrolled in intermediate or honours level courses in this sample. Courses at honours level were restricted to the Arts Faculty (Richardson, 2005). We examined whether course level had an influence on the substantive findings for the N = 1,482 students enrolled in art courses. We did not find any effect of course type that changed the moderation or moderated mediation findings.
Procedure and Measures
The procedure was the same as that reported in Richardson (2005). Questionnaires were mailed directly to students in January 2002, followed by a reminder later in the same month. Participants returned completed copies of the questionnaire via mail. Student responses were identified by a special barcode attached to the questionnaire. Only the measures pertinent to the current study are reported here. The re-analysis of the data from this study was approved by the lead author’s (HD) University Human Research Ethics Committee.
Deep and surface approaches to learning were measured using the RASI (Entwistle et al., 2000). Surface and deep approaches were each assessed using 16 items rated on a five-point Likert scale ranging from disagree (1) to agree (5). An example item from the deep approach subscale was “I usually set out to understand for myself the meaning of what we have to learn.” A surface approach item was “I find I have to concentrate on memorising a good deal of what I have to learn.” The internal reliabilities of these subscales from Richardson (2005) were both moderate: α = 0.66 for the deep approach subscale, and α = 0.63 for the surface approach subscale.
Demographic variables, including age and gender, and final course grades were derived directly from student records. An overall mark for the course was derived by averaging the two assessment components, consisting of coursework and a traditional examination. Potential scores for achievement ranged from 0 to 100, with a pass mark of 40.
Data Analysis
For the United Kingdom participants on the RASI, 89 students had not provided a response to one or more of the 52 items. In most cases, these were regarded as items that did not apply to the student, and so they were coded as “doesn’t apply to me.” Students who had missed four or more items in this scale were dropped from further analysis. There were 2,144 students with complete data on age, gender, learning approach, and course satisfaction.
Again, we used Hayes (2013) PROCESS macro to investigate our hypothesised model for the United Kingdom sample. All variables were checked for normality and transformed if necessary. All variables were mean centred using a z-transformation before being entered into the PROCESS models.
Results
Descriptive statistics and correlations for the United Kingdom sample are shown in Table 1. All associations found in the Australian sample were replicated in the United Kingdom sample.
The Effects of Age and Gender on Learning Approach
The moderator models for the United Kingdom sample are shown in Table 2. In contrast to the Australian sample, gender was a significant and positive predictor of surface learning, indicating that women were more likely to report a surface approach to their studies. Once again, there was no interaction between age and gender in predicting surface approach. Neither age nor the interaction between age and gender predicted academic achievement.
Comparison of the conditional effects of age on deep learning among men and women separately indicated a similar pattern of results to those found in Study 1: For males, age did not predict deep learning, b = 0.05, SE = 0.03, t (772) = 1.53, p = 0.127. In contrast, the effect for women was positive, with older women reporting deeper learning approaches than younger women, b = 0.17, SE = 0.03, t (1396) = 5.96, p < 0.001. The interaction effect is illustrated in Figure 2B.
Regions of Significance
Figure 3B shows the Johnson-Neyman plot for the United Kingdom sample. The figure demonstrates that the effect of gender on deep learning transitioned to positive as age increased. The effect of gender on deep learning was significant at z-score ages above 0.32, with 36.81% of the sample falling at this age or above. This value corresponded to an actual age of 52.07. Above the age of 52.07 years, gender had a significant and positive effect on deep learning, such that women reported more deep learning approaches than men. Below this age, the effect of gender on deep learning was not significant.
Conditional Process Analysis
Results of the conditional process analysis for the United Kingdom sample are shown in Figure 4B. Examination of the bootstrapped 95% CIs once again indicated that deep learning only mediated the association between gender and achievement when students were older. The index of moderated mediation supported this finding in the United Kingdom sample, a3b1 = 0.01, SE = 0.00, 95% CI (0.0019, 0.0173).
Discussion
This study investigated the effects of age, gender, and their interaction on approaches to learning and academic achievement in higher education. Consistent with the findings of previous research, older students in both of the current samples reported deeper learning and less surface learning (Zeegers, 2001; Salamonson et al., 2013). The findings for the main effect of gender were clear: No overall effect of gender was found in either sample for deep learning. Women in the Australian sample were less likely to report surface learning strategies; however, women in the United Kingdom sample were more likely to report a surface approach to learning.
The Australian sample was collected from students studying via on-campus mode (Rubin et al., 2018), while the United Kingdom dataset was derived from students in a distance learning environment (Richardson, 2005). Evidence suggests that learning approach changes depending on the delivery context. For example, Richardson et al. (1999) found that female students enrolled in distance education were more likely to report a reproducing orientation to their studies, a learning strategy that is related to the surface learning approach. Richardson et al. (1999) suggested that older female students in this sample might be driven to adopt a reproducing orientation because of their competing occupational and domestic responsibilities. Hence, the difference between our two samples on surface learning might simply reflect the difference between on-campus and distance education groups of students.
In these studies, age predicted deep learning more strongly among women than men. This finding replicated and extended Rubin et al.’s (2018) results to a sample of distance education students and a different measure of learning approach (the RASI). Rubin et al. (2018) was the first that we are aware of with a sufficiently powered study to identify that older women might exhibit deeper learning than either younger women or men. The quantitative findings further accord with qualitative studies documenting the reasons given by women for attending higher education (McCune et al., 2010). Future studies should test for not only age differences in deep learning, but interaction effects between age and gender. In contrast to the findings for deep learning, there was no interaction between age and gender for surface learning in either sample of the current study; only main effects of age and gender.
The current study extended previous work by demonstrating that the mediation of the relationship between gender and academic achievement by deep learning was only significant among older students. The present research provided some insight into the demographic influences on higher education achievement, and the mechanisms underlying discrepancies in achievement between genders, at least for older students (Voyer and Voyer, 2014).
Strengths of the Current Studies
Both studies retained the original continuous structure of the age variable in both samples. Previous research has tended to dichotomise age to split students into school leavers and non-school leaver groups (Fragoso et al., 2013). Using this approach can increase the risk of both false positive (Type I) and false negative (Type II) errors (MacCallum et al., 2002; DeCoster et al., 2009). The present study achieved an increase in statistical power by treating age as a continuous variable in both samples (Royston et al., 2006).
The current research forms a conceptual replication of the findings on learning approach (Zwaan et al., 2018). Zwaan et al. (2018) described a conceptual replication as a study where there are changes to the original procedures that might change the observed pattern of results. The current study provides a conceptual replication because it varies the context in which students are studying (on-campus traditional university delivery versus distance education), the country they are studying in (Australia versus the United Kingdom), and the measurement of the dependent variable with two prominent measures of learning approach (the RASI and the SPQ). We found some evidence of contextual effects for surface learning, where women were more likely to report surface approaches in distance education, and less likely to report them in an on-campus, younger student sample. Regardless of the context or the learning approach questionnaire used, the substantive pattern of results for deep learning and academic achievement remained the same, lending further support to our findings.
Limitations and Directions for Future Research
Only 367 of the 983 Australian students provided permission for their academic transcripts to be utilised. This represents only 37.3% of the entire sample reported in Rubin et al. (2018). This introduces the possibility of attrition bias, which is unlikely because the demographics of the subset reported in this paper did not differ from the larger sample. Further, simulation of attrition in longitudinal studies suggests that estimates of associations between variables only become biased when attrition is dependent on both baseline and follow-up variables (Gustavson et al., 2012). This was not the case in the current study, where only the follow-up variables were subject to the attrition effect. We further did not collect information about ethnicity in either study. The Australian study was originally designed to examine the experiences of students who were first in family to attend university (Rubin et al., 2018), while the United Kingdom sample was used to examine whether course experiences were associated with learning approaches (Richardson, 2005). Evaluating the influence of ethnic background can be challenging, because the ethnic categories do not readily transfer from one country or culture to another. Second, there is limited research thus far on the effects of ethnic background on Australian tertiary education attainment. Researchers in Australia instead prefer to focus on the major categories of non-traditional university students, including Aboriginal and Torres Strait Islander status and non-English speaking background (Li and Carroll, 2017). Finally, the under-attainment of ethnic minority students in the United Kingdom does not appear to be explained by differences in learning approaches (Richardson, 2010). This does not exclude the possibility that ethnic minority status might influence university attainment in Australian students. Future research might wish to examine the influence of ethnic background on learning approaches and subsequent achievement.
Although this research represents the first that we are aware of to compare the learning approaches of distance learners versus on-campus students, neither study was designed to do so. Students who are distance learners tend to be older and are more likely to be female, a factor we have explicitly modelled in both datasets (Ke and Xie, 2009; Quinn, 2009). These student groups also differ in ways that we did not measure. In a recent Australian study explicitly designed to compare the two groups of students, Johnson (2015) found that students studying on campus had higher extrinsic achievement motivation and self-reported greater need for peer and teacher support in their learning. Students who choose to study online also display different personality traits, such that they are lower on trait extraversion, independence, and higher in self-control than their on-campus peers (MacGregor, 2002). A recent comparison of online versus blended learning students found that online students had more adaptive self-regulated learning strategies (Broadbent and Fuller-Tyszkiewicz, 2018). However, a meta-analysis found no differences in satisfaction between distance education and traditional education formats (Allen et al., 2002). In an examination of students studying a first year biology course in on-campus versus distance modes, those studying on campus make less use of deep learning approaches, and used more surface approaches (Quinn, 2009). However, the group studying on campus in this study were also younger than the distance mode students, making the effect of context difficult to tease apart from the effects of demographics. Future research might wish to compare the learning approaches of students studying via online versus on-campus modes using a purposeful research design, to verify the current findings.
The present study extends the applicability of Rubin et al.’s (2018) findings to a sample of distance education students who responded to a different learning approach questionnaire and who were primarily studying from within the United Kingdom (Richardson, 2005). While the convergence in results suggests stronger evidence for the older women, deeper learning effect found in the original Rubin et al. (2018) paper, it does not extend to different cultural contexts. For example, evidence suggests that Chinese students have qualitatively different beliefs about learning (Li, 2003, 2005). These findings might explain the recent evidence that international students from this region are more likely than Australian students to adopt a deep learning approach (Bowden et al., 2015). However, Bowden et al.’s (2015) research only examines the learning approach of these groups of students when they are studying as international students. This is a problem because learning approaches are supposed to change with the context of study. Hence, these same students might adopt completely different approaches to learning if they are studying for their degree in their country of origin. Further research might examine age and gender together as predictors of learning approach in students from different cultural backgrounds who are studying in their own country.
Implications
The present research demonstrated that gender differences in deep learning help to enhance the achievement of older women only. This raises two related points. The first is why deep learning should only matter for the academic achievement of older women. The second is, if deep learning only matters for explaining the academic achievement of older students, then we need to identify what explains the gender difference in achievement among younger students.
The current research suggests that we should reconsider the conception of the older student learner, especially the older woman, as a disadvantaged group in higher education. The discourse on older learners more generally places them as isolated (Mallman and Lee, 2017), sometimes stigmatised by younger students (Mallman and Lee, 2016), with fewer sources of social support (Carney-Crompton and Tan, 2002). Previous research has also suggested that older female students might be uniquely burdened by family tasks that take attention away from their studies (Gill et al., 2015). The findings of this study suggest that once older students, particularly older female students, make the decision to engage with higher education, they have a particularly rich understanding of the meaning and relevance of their studies (McCune et al., 2010). Consistent with previous research (Lin and Wang, 2018; Rubin et al., 2018), our findings suggest that older women appear more motivated to understand the meaning and application of what they are studying. We do not suggest that older women might be more generally motivated to attend higher education. We instead suggest that older women who have already decided to attend a higher education institution are more likely to employ a deep learning approach to their studies, an approach that has explanatory power for this group’s achievement at university. Higher education institutions might wish to pay more attention to the barriers that older women perceive in attending university, as the evidence suggests that once they arrive they are active, independent, and successful learners.
Conclusion
The present research suggests that older women are more likely to deploy learning approaches associated with better achievement, and more likely to subsequently achieve at university. Future research should focus more on how to maximise the potential of this group of higher education students and harness it to support others to succeed in higher education.
Data Availability Statement
The data analyzed in this study is subject to the following licenses/restrictions: Participants did not consent to non-identifiable open access to this data at the time of data collection. For the United Kingdom sample this was in 2002, while the Australian sample was collected in 2013. Neither sample gave consent for this data to be available to other researchers not affiliated with the original research team. Requests to access these datasets should be directed to heather.douglas@newcastle.edu.au.
Ethics Statement
The studies involving human participants were reviewed and approved by the Human Research Ethics Committee at Murdoch University, Perth, Australia. The participants provided their written informed consent to participate in each study.
Author Contributions
HD, MR, JS, ES, and SM contributed to the conception and design of the Australian study. HD collected the data for the Australian sample. JR contributed the conception, design, and data collection of the United Kingdom study. HD performed the statistical analysis. HD and MR wrote the first draft of the manuscript. All authors contributed to the manuscript revision, read, and approved the submitted version.
Funding
The first author was supported by The University of Newcastle Women in Research Fellowship (G1901347). The research reported in Study 1 was funded by an Australian research grant from the National Centre for Student Equity in Higher Education Research Grants Program.
Conflict of Interest
The authors declare that the research was conducted in the absence of any commercial or financial relationships that could be construed as a potential conflict of interest.
Acknowledgments
Part of the work in this manuscript was carried out at Murdoch University Singapore Campus, #06-06 Kings Centre, 390 Havelock Road Singapore 169662. HD has since moved to The University of Newcastle, Australia.
References
Allen, M., Bourhis, J., Burrell, N., and Mabry, E. (2002). Comparing student satisfaction with distance education to traditional classrooms in higher education: a meta-analysis. Am. J. Dist. Educ. 16, 83–97. doi: 10.1207/s15389286ajde1602_3
Andreou, E., Vlachos, F., and Andreou, G. (2006). Approaches to studying among Greek university students: the impact of gender, age, academic discipline and handedness. Educ. Res. 48, 301–311. doi: 10.1080/00131880600992363
Biggs, J., Kember, D., and Leung, D. Y. (2001). The revised two-factor study process questionnaire: R-SPQ-2F. Br. J. Educ. Psychol. 71, 133–149. doi: 10.1348/000709901158433
Blake, K. R., and Gangestad, S. (2020). On attenuated interactions, measurement error, and statistical power: guidelines for social and personality psychologists. Pers. Soc. Psychol. Bull. doi: 10.1177/0146167220913363
Bonneville-Roussy, A., Evans, P., Verner-Filion, J., Vallerand, R. J., and Bouffard, T. (2017). Motivation and coping with the stress of assessment: gender differences in outcomes for university students. Contemp. Educ. Psychol. 48, 28–42. doi: 10.1016/j.cedpsych.2016.08.003
Bowden, M. P., Abhayawansa, S., and Manzin, G. (2015). A multiple cross-cultural comparison of approaches to learning. Compare J. Comparat. Intern. Educ. 45, 272–294. doi: 10.1080/03057925.2013.841465
Broadbent, J., and Fuller-Tyszkiewicz, M. (2018). Profiles in self-regulated learning and their correlates for online and blended learning students. Educ. Technolo. Res. Dev. 66, 1435–1455. doi: 10.1007/s11423-018-9595-9
Caplan, P. J., Crawford, M., Hyde, J. S., and Richardson, J. T. E. (1997). Gender Differences in Human Cognition. New York, NY: Oxford University Press.
Carney-Crompton, S., and Tan, J. (2002). Support systems, psychological functioning, and academic performance of nontraditional female students. Adult Educ. Q. 52, 140–154. doi: 10.1177/0741713602052002005
Cohen, J. (1988). Statistical Power Analysis for the Behavioral Sciences. Hillsdale, NJ: L. Erlbaum Associates.
DeCoster, J., Iselin, A. R., and Gallucci, M. (2009). A conceptual and empirical examination of justifications for dichotomization. Psychol. Methods 14, 349–366. doi: 10.1037/a0016956
Downey, R. G., and King, C. V. (1998). Missing data in likert ratings: a comparison of replacement methods. J. Gen. Psychol. 125:175. doi: 10.1080/00221309809595542
Duff, A. (2003). Quality of learning on an MBA programme: the impact of approaches to learning on academic performance. Educ. Psychol. 23, 123–139. doi: 10.1080/01443410303230
Entwistle, N. (1987). “A model of the teaching-learning process,” in Student Learning: Research in Education and Cognitive Psychology, eds T. E. Richardson John, M. W. Eysenck, and D. W. Piper, (Guildford: SRHE & Open University Press), 13–28.
Entwistle, N., and Tait, H. (1995). Revised Approaches to Studying Inventory. Edinburgh: University of Edinburgh.
Entwistle, N., Tait, H., and McCune, V. (2000). Patterns of response to an approaches to studying inventory across contrasting groups and contexts. Eur. J. Psychol. Educ. 15:33. doi: 10.1007/bf03173165
Fragoso, A., GonÇAlves, T., Ribeiro, C. M., Monteiro, R., Quintas, H., Bago, J., et al. (2013). The transition of mature students to higher education: challenging traditional concepts? Stud. Educ. Adults 45, 67–81. doi: 10.1080/02660830.2013.11661642
Gill, B., Hayes, S., and Senior, C. (2015). The effects of family support and gender on mature student engagement in higher education. Front. Psychol. 6:156. doi: 10.3389/fpsyg.2015.00156
Gow, L., and Kember, D. (1990). Does higher education promote independent learning? Higher Educ. 19, 307–322.
Gustavson, K., von Soest, T., Karevold, E., and Røysamb, E. (2012). Attrition and generalizability in longitudinal studies: findings from a 15-year population-based study and a Monte carlo simulation study. BMC Public Health 12:918. doi: 10.1186/1471-2458-12-918
Harper, G., and Kember, D. (1986). Approaches to study of distance education students. Br. J. Educ. Technol. 17, 212–222.
Hayes, A. F. (2013). Introduction to Mediation, Moderation, and Conditional Process Analysis: A Regression-Based Approach. New York, NY: Guilford Press.
Heijne-Penninga, M., Kuks, J. B. M., Hofman, W. H. A., and Cohen-Schotanus, J. (2008). Influence of open- and closed-book tests on medical students’ learning approaches. Med. Educ. 42, 967–974. doi: 10.1111/j.1365-2923.2008.03125.x
Heijne-Penninga, M., Kuks, J. B. M., Hofman, W. H. A., and Cohen-Schotanus, J. (2010). Influences of deep learning, need for cognition and preparation time on open- and closed-book test performance. Med. Educ. 44, 884–891. doi: 10.1111/j.1365-2923.2010.03732.x
Heikkilä, A., and Lonka, K. (2006). Studying in higher education: students’ approaches to learning, self-regulation, and cognitive strategies. Stud. Higher Educ. 31, 99–117. doi: 10.1080/03075070500392433
Johnson, G. M. (2015). On-campus and fully-online university students: comparing demographics, digital technology use and learning characteristics. J. Univers. Teach. Learn. Pract. 12, 1–13.
Justice, E. M., and Dornan, T. M. (2001). Metacognitive differences between traditional-age and nontraditional-age college students. Adult Educ. Q. 51, 236–249. doi: 10.1177/074171360105100305
Kasworm, C. E. (2018). Adult students: a confusing world in undergraduate higher education. J. Continu. High. Educ. 66, 77–87. doi: 10.1080/07377363.2018.1469077
Ke, F., and Xie, K. (2009). Toward deep learning for adult students in online courses. Internet High. Educ. 12, 136–145. doi: 10.1016/j.iheduc.2009.08.001
Li, I., and Carroll, D. R. (2017). Factors Influencing University Satisfaction, Dropout And Academic Performance: An Australian Higher Education Equity Perspective. Perth: Curtin University.
Li, J. (2003). U.S. and Chinese cultural beliefs about learning. J. Educ. Psychol. 95, 258–267. doi: 10.1037/0022-0663.95.2.258
Li, J. (2005). Mind or virtue: western and chinese beliefs about learning. Curr. Direct. Psychol. Sci. 14, 190–194. doi: 10.1111/j.0963-7214.2005.00362.x
Lin, X., and Wang, C. (2018). Achievement goal orientations and self-regulated learning strategies of adult and traditional learners. New Horiz. Adult Educ. Hum. Resour. Dev. 30, 5–22. doi: 10.1002/nha3.20229
MacCallum, R. C., Zhang, S., Preacher, K. J., and Rucker, D. D. (2002). On the practice of dichotomization of quantitative variables. Psychol. Methods 7, 19–40. doi: 10.1037/1082-989X.7.1.19
MacGregor, C. J. (2002). Personality differences between online and face-to-face students. J. Continu. High. Educ. 50, 14–23. doi: 10.1080/07377366.2002.10401201
Mallman, M., and Lee, H. (2016). Stigmatised learners: mature-age students negotiating university culture. Br. J. Sociol. Educ. 37, 684–701. doi: 10.1080/01425692.2014.973017
Mallman, M., and Lee, H. (2017). Isolated learners: young mature-age students, university culture, and desire for academic sociality. Intern. J. Lifelong Educ. 36, 512–525. doi: 10.1080/02601370.2017.1302012
Marton, F., and Säljö, R. (1976). On qualitative differences in learning: I − outcome and process. Br. J. Educ. Psychol. 46, 4–11. doi: 10.1111/j.2044-8279.1976.tb02980.x
May, W., Chung, E., Elliott, D., and Fisher, D. (2012). The relationship between medical students’ learning approaches and performance on a summative high-stakes clinical performance examination. Med. Teach. 34, e236–e241. doi: 10.3109/0142159X.2012.652995
McCune, V., Hounsell, J., Christie, H., Cree, V. E., and Tett, L. (2010). Mature and younger students’ reasons for making the transition from further education into higher education. Teach. High. Educ. 15, 691–702. doi: 10.1080/13562517.2010.507303
Paver, B., and Gammie, E. (2005). Constructed gender, approach to learning and academic performance. Acc. Educ. 14, 427–444. doi: 10.1080/06939280500347142
Quinn, F. C. (2009). Learning in first-year biology: approaches of distance and on-campus students. Res. Sci. Educ. 41, 99–121. doi: 10.1007/s11165-009-9148-7
Richardson, J. T. (2010). Conceptions of learning and approaches to studying among White and ethnic minority students in distance education. Br. J. Educ. Psychol. 80(Pt 4), 535–556. doi: 10.1348/000709910X489283
Richardson, J. T. E. (1995). Mature students in higher education: II. An investigation of approaches to studying and academic performance. Stud. High. Educ. 20, 5–17. doi: 10.1080/03075079512331381760
Richardson, J. T. E. (2000). Researching Student Learning: Approaches to Studying in Campus-Based and Distance Education. Buckingham: The Society for Research into Higher Education & Open University Press.
Richardson, J. T. E. (2005). Students’ perceptions of academic quality and approaches to studying in distance education. Br. Educ. Res. J. 31, 7–27. doi: 10.1080/014119205200031001
Richardson, J. T. E. (2006). Investigating the relationship between variations in students’ perceptions of their academic environment and variations in study behaviour in distance education. Br. J. Educ. Psychol. 76, 867–893. doi: 10.1348/000709905X69690
Richardson, J. T. E. (2007). Motives, attitudes and approaches to studying in distance education. High. Educ. 54, 385–416. doi: 10.1007/s10734-006-9003-y
Richardson, J. T. E. (2013). Approaches to studying across the adult life span: evidence from distance education. Learn. Individ. Differ. 26, 74–80. doi: 10.1016/j.lindif.2013.04.012
Richardson, J. T. E., Morgan, A., and Woodley, A. (1999). Approaches to studying in distance education. High. Educ. 37, 23–55. doi: 10.1023/a:1003445000716
Richardson, J. T. E., and Price, L. (2003). Approaches to studying and perceptions of academic quality in electronically delivered courses. Br. J. Educa. Technol. 34, 45–56. doi: 10.1111/1467-8535.00303
Robbins, S. B., Lauver, K., Le, H., Davis, D., Langley, R., and Carlstrom, A. (2004). Do psychosocial and study skill factors predict college outcomes? A meta-analysis. Psychol. Bull. 130, 261–288. doi: 10.1037/0033-2909.130.2.261
Royston, P., Altman, D. G., and Sauerbrei, W. (2006). Dichotomizing continuous predictors in multiple regression: a bad idea. Statist. Med. 25, 127–141. doi: 10.1002/sim.2331
Rubin, M., Scevak, J., Southgate, E., Macqueen, S., Williams, P., and Douglas, H. (2018). Older women, deeper learning, and greater satisfaction at university: age and gender predict university students’ learning approach and degree satisfaction. J. Divers. High. Educ. 11, 82–96. doi: 10.1037/dhe0000042
Sadler-Smith, E., and Tsang, F. (1998). A comparative study of approaches to studying in Hong Kong and the United Kingdom. Br. J. Educ. Psychol. 68, 81–93. doi: 10.1111/j.2044-8279.1998.tb01276.x
Salamonson, Y., Weaver, R., Chang, S., Koch, J., Bhathal, R., Khoo, C., et al. (2013). Learning approaches as predictors of academic performance in first year health and science students. Nurse Educ. Today 33, 729–733. doi: 10.1016/j.nedt.2013.01.013
Smith, S. N., and Miller, R. J. (2005). Learning approaches: examination type, discipline of study, and gender. Educ. Psychol. 25, 43–53. doi: 10.1080/0144341042000294886
Tarabashkina, L., and Lietz, P. (2011). The impact of values and learning approaches on student achievement: gender and academic discipline influences. Issues Educ. Res. 21, 210–231.
Voyer, D., and Voyer, S. D. (2014). Gender differences in scholastic achievement: a meta-analysis. Psychol. Bull. 140, 1174–1204. doi: 10.1037/a0036620
Wilson, K. L., Smart, R. M., and Watson, R. J. (1996). Gender differences in approaches to learning in first year psychology students. Br. J. Educ. Psychol. 66, 59–71. doi: 10.1111/j.2044-8279.1996.tb01176.x
Zeegers, P. (2001). Approaches to learning in science: a longitudinal study. Br. J. Educ. Psychol. 71(Pt 1), 115–132. doi: 10.1348/000709901158424
Keywords: academic achievement, age, conditional process analysis, gender differences, learning approach
Citation: Douglas HE, Rubin M, Scevak J, Southgate E, Macqueen S and Richardson JTE (2020) Older Women, Deeper Learning: Age and Gender Interact to Predict Learning Approach and Academic Achievement at University. Front. Educ. 5:158. doi: 10.3389/feduc.2020.00158
Received: 28 April 2020; Accepted: 05 August 2020;
Published: 25 August 2020.
Edited by:
Angela Jocelyn Fawcett, Swansea University, United KingdomReviewed by:
Janet Hoskin, University of East London, United KingdomCristina C. Vieira, University of Coimbra, Portugal
Copyright © 2020 Douglas, Rubin, Scevak, Southgate, Macqueen and Richardson. This is an open-access article distributed under the terms of the Creative Commons Attribution License (CC BY). The use, distribution or reproduction in other forums is permitted, provided the original author(s) and the copyright owner(s) are credited and that the original publication in this journal is cited, in accordance with accepted academic practice. No use, distribution or reproduction is permitted which does not comply with these terms.
*Correspondence: Heather E. Douglas, heather.douglas@newcastle.edu.au