Abstract
Background
Youth body mass index (BMI), derived from self-reported height and weight, is commonly prone to nonreporting. A considerable proportion of overweight and obesity (OWOB) research relies on such self-report data, however little literature to date has examined this nonreporting and the potential impact on research conclusions. The objective of this study was to examine the characteristics and predictors of missing data in youth BMI, height, and weight.
Methods
Using a sample of 74,501 Canadian secondary school students who participated in the COMPASS study in 2018/19, sex-stratified generalized linear mixed models were run to examine predictors of missing data while controlling for school-level clustering.
Results
In this sample, 31% of BMI data were missing. A variety of diet, exercise, mental health, and substance use variables were associated with BMI, height, and weight missingness. Perceptions of being overweight (females: 95% CI (1.42,1.62), males: 95% CI (1.71,2.00)) as well as intentions to lose weight (females: 95% CI (1.17,1.33), males: 95% CI (1.13,1.32)) were positively associated with BMI missingness.
Conclusions
Findings from this study suggest that nonreporting in youth height and weight is likely somewhat related to the values themselves, and hint that social desirability may play a substantial role in nonreporting. The predictors of missingness identified in this study can be used to inform future studies on the potential bias stemming from missing data and identify auxiliary variables that may be used for multiple imputation approaches.
This is a preview of subscription content, access via your institution
Access options
Subscribe to this journal
Receive 12 print issues and online access
$259.00 per year
only $21.58 per issue
Buy this article
- Purchase on Springer Link
- Instant access to full article PDF
Prices may be subject to local taxes which are calculated during checkout


Similar content being viewed by others
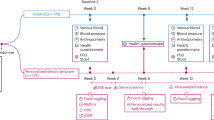
Data availability
Data are from the COMPASS study whose authors may be contacted at adoggett@uwaterloo.ca. COMPASS data are stored at the University of Waterloo on a secure server. STL maintains ownership of all COMPASS data, and will grant access to COMPASS collaborators, their research teams, and external research teams and students. For researchers to gain access to the COMPASS data, they must successfully complete the COMPASS data usage application, which will be reviewed by STL (https://uwaterloo.ca/compass-system/information-researchers). Researchers can contact the University of Waterloo’s Office of Research Ethics (ohrac@uwaterloo.ca) for further information.
References
Lin JY, Lu Y, Tu X. How to avoid missing data and the problems they pose: design considerations. Shanghai Arch Psychiatry. 2012;24:181–5. http://www.ncbi.nlm.nih.gov/pubmed/25324625.
Kelly N, Harpel T, Fontes A, Walters C, Murphy J. An examination of social desirability bias in measures of college students’ financial behavior. Coll Stud J. 2017;51:115–28. https://go.gale.com/ps/i.do?&id=GALE%7CA487602758&v=2.1&u=uniwater&it=r&p=AONE&sw=w.
Latkin CA, Edwards C, Davey-Rothwell MA, Tobin KE. The relationship between social desirability bias and self-reports of health, substance use, and social network factors among urban substance users in Baltimore, Maryland. Addict Behav. 2017;73:133–6. https://www.sciencedirect.com/science/article/pii/S0306460317301752.
Maclennan B, Kypri K, Langley J, Room R. Non-response bias in a community survey of drinking, alcohol-related experiences and public opinion on alcohol policy. Drug Alcohol Depend. 2012;126:189–94. https://www.sciencedirect.com/science/article/pii/S0376871612001779.
Schafer JL, Graham JW. Missing data: our view of the state of the art. Psychol Methods. 2002;7:147–77.
Eekhout I, De Boer RM, Twisk JWR, De Vet HCW, Heymans MW. Missing data: a systematic review of how they are reported and handled. Epidemiology. 2012;23:729–32. http://content.wkhealth.com/linkback/openurl?sid=WKPTLP:landingpage&an=00001648-201209000-00013.
Karahalios A, Baglietto L, Carlin JB, English DR, Simpson JA. A review of the reporting and handling of missing data in cohort studies with repeated assessment of exposure measures. BMC Med Res Methodol. 2012;12:96 https://bmcmedresmethodol.biomedcentral.com/articles/10.1186/1471-2288-12-96.
Himes JH, Faricy A. Validity and reliability of self-reported stature and weight of US adolescents. Am J Hum Biol. 2001;13:255–60. http://doi.wiley.com/10.1002/1520-6300%28200102/03%2913%3A2%3C255%3A%3AAID-AJHB1036%3E3.0.CO%3B2-E.
Sherry B, Jefferds ME, Grummer-Strawn LM. Accuracy of adolescent self-report of height and weight in assessing overweight status: a literature review. Arch Pediatr Adolesc Med. 2007;161:1154–61. http://archpedi.jamanetwork.com/article.aspx?doi=10.1001/archpedi.161.12.1154.
Green MA. Dowe need to think beyond BMI for estimating population-level health risks? J Public Health. 2016;38:192–3.
Adab P, Pallan M, Whincup PH. Is BMI the best measure of obesity? BMJ. 2018;360:15–6. https://doi.org/10.1136/bmj.k1274. Available from
Must A, Anderson SE. Body mass index in children and adolescents: considerations for population-based applications. Int J Obes. 2006;30:590–4.
Himes JH. Challenges of accurately measuring and using BMI and other indicators of obesity in children. Pediatrics [Internet]. 2009;124 Suppl 1:s3–22. http://pediatrics.aappublications.org/lookup/doi/10.1542/peds.2008-3586D.
Guh DP, Zhang W, Bansback N, Amarsi Z, Birmingham CL, Anis AH. The incidence of co-morbidities related to obesity and overweight: a systematic review and meta-analysis. BMC Public Health. 2009;9:88 https://bmcpublichealth.biomedcentral.com/track/pdf/10.1186/1471-2458-9-88?site=bmcpublichealth.biomedcentral.com.
Aceves-Martins M, Whitehead R, Inchley J, Giralt M, Currie C, Solà R. Self-reported weight and predictors of missing responses in youth. Nutrition. 2018;53:54–8. https://doi.org/10.1016/j.nut.2018.01.003.
Arbour-Nicitopoulos KP, Faulkner GE, Leatherdale ST. Learning from non-reported data: Interpreting missing body mass index values in young children. Meas Phys Educ Exerc Sci. 2010;14:241–51. https://journals.scholarsportal.info/pdf/1091367x/v14i0004/241_lfndimmiviyc.xml.
Fonseca H, De Gaspar Matos M, Guerra A, Gomes-Pedro J. Emotional, behavioural and social correlates of missing values for BMI. Arch Dis Child. 2009;94:104–9. http://www.ncbi.nlm.nih.gov/pubmed/17367722.
Tiggemann M. Nonreporting of body mass index: a research note on the interpretation of missing data. Int J Eat Disord. 2006;39:346–9. https://doi.org/10.1002/eat.20264.
Thompson-Haile A, Leatherdale ST. Student-level data collection procedures. 2013. COMPASS technical report series. Vol 1, issue 5. https://uwaterloo.ca/compass-system/publications/student-level-data-collection-procedures.
Leatherdale ST, Brown KS, Carson V, Childs RA, Dubin JA, Elliott SJ, et al. The COMPASS study: a longitudinal hierarchical research platform for evaluating natural experiments related to changes in school-level programs, policies and built environment resources. BMC Public Health. 2014;14:331 https://bmcpublichealth.biomedcentral.com/articles/10.1186/1471-2458-14-331.
Haziza D, Lesage É. A discussion of weighting procedures for unit nonresponse. J Off Stat. 2016;32:129–45. https://doi.org/10.1515/JOS-2016-0006.
Fonseca H, De Gaspar Matos M, Guerra A, Gomes-Pedro J. Emotional, behavioural and social correlates of missing values for BMI. Arch Dis Child. 2009;94:104–9. http://www.ncbi.nlm.nih.gov/pubmed/18801764.
Radloff LS. The CES-D scale: a self-report depression scale for research in the general population. Appl Psychol Meas. 1977;1:385–401.
Spitzer RL, Kroenke K, Williams JBW, Löwe B. A brief measure for assessing generalized anxiety disorder: the GAD-7. Arch Intern Med. 2006;166:1092–7.
Gratz KL, Roemer L. Multidimensional assessment of emotion regulation and dysregulation: development, factor structure, and initial validation of the difficulties in emotion regulation scale. J Psychopathol Behav Assess. 2004;26:41–54.
Diener E, Wirtz D, Tov W, Kim-Prieto C, Choi Dw, Oishi S. et al. New well-being measures: short scales to assess flourishing and positive and negative feelings. Soc Indic Res. 2010;97:143–56.
Marsh HW, Ellis LA, Parada RH, Richards G, Heubeck BG. A short version of the self description questionnaire II: Operationalizing criteria for short-form evaluation with new applications of confirmatory factor analyses. Psychol Assess. 2005;17:81–102.
Neath AA, Cavanaugh JE. The Bayesian information criterion: background, derivation, and applications. Wiley Interdiscip Rev Comput Stat. 2012;4:199–203.
Cavanaugh JE, Neath AA. The Akaike information criterion: background, derivation, properties, application, interpretation, and refinements. Wiley Interdiscip Rev Comput Stat. 2019;11:1–11.
Ten Eyck P, Cavanaugh JE. An alternate approach to Pseudo-likelihood model selection in the generalized linear mixed modeling framework. Sankhya Indian J Stat. 2018;80B:98–122.
Sykes LL, Walker RL, Ngwakongnwi E, Quan H. A systematic literature review on response rates across racial and ethnic populations. Can J Public Health. 2010;101:213–9. http://link.springer.com/10.1007/BF03404376.
Downing A, West RM, Gilthorpe MS, Lawrence G, Forman D. Using routinely collected health data to investigate the association between ethnicity and breast cancer incidence and survival: what is the impact of missing data and multiple ethnicities? Ethn Health. 2011;16:201–12.
Ma Y, Zhang W, Lyman S, Huang Y. The HCUP SID imputation project: improving statistical inferences for health disparities research by imputing missing race data. Health Serv Res. 2018;53:1870–89.
Krieger N, Chen JT, Ware JH, Kaddour A. Race/ethnicity and breast cancer estrogen receptor status: Impact of class, missing data, and modeling assumptions. Cancer Causes Control. 2008;19:1305–18.
Voelker D, Reel J, Greenleaf C. Weight status and body image perceptions in adolescents: current perspectives. Adolesc Health Med Ther. 2015;6:149.
Tourangeau R, Yan T. Sensitive questions in surveys. Psychol Bull. 2007;133:859–83.
Felderer B, Kirchner A, Kreuter F. The effect of survey mode on data quality: disentangling nonresponse and measurement error bias. J Off Stat. 2019;35:93–115.
McCabe MP, Ricciardelli LA. Body image and strategies to lose weight and increase muscle among boys and girls. Heal Psychol. 2003;22:39–46.
Jones DC, Crawford JK. Adolescent boys and body image: weight and muscularity concerns as dual pathways to body dissatisfaction. J Youth Adolesc. 2005;34:629–36.
Sirirassamee T, Phoolsawat S, Limkhunthammo S. Relationship between body weight perception and weight-related behaviours. J Int Med Res. 2018;46:3796–808.
Gaylis JB, Levy SS, Hong MY. Relationships between body weight perception, body mass index, physical activity, and food choices in Southern California male and female adolescents. Int J Adolesc Youth. 2020;25:264–75. https://doi.org/10.1080/02673843.2019.1614465.
Wang Y, Liu H, Wu F, Yang X, Yue M, Pang Y, et al. The association between BMI and body weight perception among children and adolescents in Jilin City, China. PLoS ONE. 2018;13:1–10.
Raffoul A, Goodman S, Hammond D, Kirkpatrick SI. Weight management efforts, but not weight perceptions, are associated with dietary quality among youth and young adults in Canada. J Acad Nutr Diet. 2020:1–10. https://doi.org/10.1016/j.jand.2020.10.011.
Deierlein AL, Malkan A, Litvak J, Parekh N. Weight perception, weight control intentions, and dietary intakes among adolescents ages 10-15 years in the United States. Int J Environ Res Public Health. 2019;16:990.
Malik VS, Schulze MB, Hu FB. Intake of sugar-sweetened beverages and weight gain: a systematic review. Am J Clin Nutr. 2006;84:274–88.
Al-Hazzaa HM, Abahussain NA, Al-Sobayel HI, Qahwaji DM, Musaiger AO. Lifestyle factors associated with overweight and obesity among Saudi adolescents. BMC Public Health. 2012;12:1.
You J, Choo J. Adolescent overweight and obesity: links to socioeconomic status and fruit and vegetable intakes. Int J Environ Res Public Health. 2016;13:307.
Zakrzewski JK, Gillison FB, Cumming S, Church TS, Katzmarzyk PT, Broyles ST, et al. Associations between breakfast frequency and adiposity indicators in children from 12 countries. Int J Obes Suppl. 2015;5:S80–8.
Veltsista A, Laitinen J, Sovio U, Roma E, Järvelin MR, Bakoula C. Relationship between eating behavior, breakfast consumption, and obesity among finnish and Greek adolescents. J Nutr Educ Behav. 2010;42:417–21. https://journals-scholarsportal-info.proxy.lib.uwaterloo.ca/pdf/14994046/v42i0006/417_rbebbcoafaga.xml.
Berkey CS, Rockett HRH, Field AE, Gillman MW, Colditz GA. Sugar-added beverages and adolescent weight change. Obes Res. 2004;12:778–88.
Peart T, Mondragon HEV, Rohm-Young D, Bronner Y, Hossain MB. Weight status in US youth: the role of activity, diet, and sedentary behaviors. Am J Health Behav. 2011;35:756–65.
Livermore M, Duncan MJ, Leatherdale ST, Patte KA. Are weight status and weight perception associated with academic performance among youth? J Eat Disord. 2020;8:1–10.
Shore SM, Sachs ML, Lidicker JR, Brett SN, Wright AR, Libonati JR. Decreased scholastic achievement in overweight middle school students. Obesity. 2008;16:1535–8.
Colunga-RodrĂguez C, Orozco-Solis MG, Flores-Villavicencio ME, de-la-Roca-Chiapas JM, GĂłmez-MartĂnez R, Mercado A, et al. Body image perception and internalization problems indicators in Mexican adolescents. Psychology. 2016;07:1671–81.
Bucchianeri MM, Fernandes N, Loth K, Hannan PJ, Eisenberg ME, Neumark-Sztainer D. Body dissatisfaction: do associations with disordered eating and psychological well-being differ across race/ethnicity in adolescent girls and boys? Cult Divers Ethn Minor Psychol. 2016;22:137–46.
Rawana JS, Morgan AS. Trajectories of depressive symptoms from adolescence to young adulthood: the role of self-esteem and body-related predictors. J Youth Adolesc. 2014;43:597–611.
Woodgate RL, Busolo DS. A qualitative study on Canadian youth’s perspectives of peers who smoke: an opportunity for health promotion. BMC Public Health. 2015;15. https://www.ncbi.nlm.nih.gov/pmc/articles/PMC4692065/pdf/12889_2015_Article_2683.pdf.
Janz T. Current smoking trends. Statistics Canada. 2012. p. 11. http://www.statcan.gc.ca/pub/82-624-x/2012001/article/11676-eng.htm%5Cn. http://www.statcan.gc.ca/pub/82-624-x/2012001/article/11676-eng.pdf.
Bernat D, Gasquet N, Wilson KOD, Porter L, Choi K. Electronic cigarette harm and benefit perceptions and use among youth. Am J Prev Med. 2018;55:361–7. https://doi.org/10.1016/j.amepre.2018.04.043.
Camenga DR, Fiellin LE, Pendergrass T, Miller E, Pentz MA, Hieftje K. Adolescents’ perceptions of flavored tobacco products, including E-cigarettes: a qualitative study to inform FDA tobacco education efforts through videogames. Addict Behav. 2018;82:189–94. https://doi.org/10.1016/j.addbeh.2018.03.021.
Romijnders KAGJ, van Osch L, de Vries H, Talhout R. Perceptions and reasons regarding e-cigarette use among users and non-users: a narrative literature review. Int J Environ Res Public Health. 2018;15:1190 www.mdpi.com/journal/ijerph.
Acknowledgements
The COMPASS study has been supported by a bridge grant from the CIHR Institute of Nutrition, Metabolism and Diabetes (INMD) through the “Obesity—Interventions to Prevent or Treat” priority funding awards (OOP-110788; awarded to STL), an operating grant from the CIHR Institute of Population and Public Health (IPPH) (MOP-114875; awarded to STL), a CIHR project grant (PJT-148562; awarded to SL), a CIHR bridge grant (PJT-149092; awarded to KP/STL), a CIHR project grant (PJT-159693; awarded to KP), and by a research funding arrangement with Health Canada (#1617-HQ-000012; contract awarded to STL), and a CIHR-Canadian Centre on Substance Abuse (CCSA) team grant (OF7 B1-PCPEGT 410-10-9633; awarded to STL). The COMPASS-Quebec project additionally benefits from funding from the Ministère de la Santé et des Services sociaux of the province of Québec, and the Direction régionale de santé publique du CIUSSS de la Capitale-Nationale.
Author information
Authors and Affiliations
Contributions
AD, AC, JPC, and STL contributed to project conceptualization. AD and AC planned the methodology, and AD conducted the formal analysis. AD did the original draft preparation. SL acquired funding, managed resources, and provided supervision for the host study and corresponding data in this manuscript. AD, AC, JPC, and STL reviewed and edited the manuscript for critical content. All authors have read and agreed to the published version of the manuscript.
Corresponding author
Ethics declarations
Competing interests
The authors declare no competing interests.
Additional information
Publisher’s note Springer Nature remains neutral with regard to jurisdictional claims in published maps and institutional affiliations.
Rights and permissions
About this article
Cite this article
Doggett, A., Chaurasia, A., Chaput, JP. et al. Learning from missing data: examining nonreporting patterns of height, weight, and BMI among Canadian youth. Int J Obes 46, 1598–1607 (2022). https://doi.org/10.1038/s41366-022-01154-8
Received:
Revised:
Accepted:
Published:
Issue Date:
DOI: https://doi.org/10.1038/s41366-022-01154-8